Risky Business: How Data Analytics Can Help
Kathleen Long is using a combination of behavioral analytics, Bayesian engineering and big data to help companies better determine and mitigate business risk.
Topics
Competing With Data & Analytics
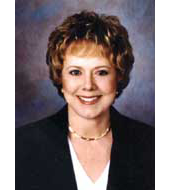
Kathleen Long, CEO of Montage Analytics
A socio-cybernetician and behavioral scientist, Kathleen Long battles operational risk. As CEO of Montage Analytics, a Mountain View, Calif., consultancy offering risk assessment software services and analytic reports, Long has combined her training with the experience of Montage Analytic’s CTO, Doug Campbell, in Bayesian network design. The company helps organizations better understand and mitigate everything from risky business practices and employee fraud to the big, unwieldy, nearly undetectable risks referred to as “black swans.”
This isn’t an easy undertaking. Part of the problem, according to Long, is that not everyone knows how to define operational risk (if you can’t define it, you can’t guard against it). At the same time, the risk landscape is changing so fast that what happened yesterday is no longer a marker for what might happen tomorrow.
“We are living in unprecedented times. You can’t say the past is a reliable predictor of the future,” says Long. “Too many events happen that are black swans that come out of the blue — Bernie Madoff, 9/11. Things have changed dramatically. And the fact that we are living in an increasingly globalized and networked world means that even small events happening somewhere on the other side of the world can quickly cascade around the globe and affect us in places we didn’t expect.”
In a conversation with Renee Boucher Ferguson, a contributing editor at MIT Sloan Management Review, Long discussed the changing risk landscape and how big data and behavioral science can help.
What are the different types of risk organizations need to think about, from operational risk to the so-called black swans?
A lot of people don’t understand what operational risk is, but it has to do with the people, processes and systems in place to produce the company’s product or service. That said, there are many different kinds of operational risk. For example: Internal fraud, external fraud, client, products and business practices, damage to physical assets, business disruption and system failures. Then there are execution, delivery and process management risks. Did I mention employment practices and workplace safety? Those are all the kinds of operational risk.
What is a black swan risk?
A black swan risk has been recently defined — it’s actually a metaphor that was based on a theory developed by
Comments (2)
sanchezjb@attglobal.net
raguand