Decisions 2.0: The Power of Collective Intelligence
Information markets, wikis and other applications that tap into the collective intelligence of groups have recently generated tremendous interest. But what”s the reality behind the hype?
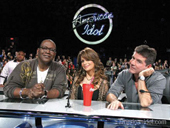
Image courtesy of “American Idol.”
The human brain is a magnificent instrument that has evolved over thousands of years to enable us to prosper in an impressive range of conditions. But it is wired to avoid complexity (not embrace it) and to respond quickly to ensure survival (not explore numerous options). In other words, our evolved decision heuristics have certain limitations, which have been studied extensively and documented over the last few decades, particularly by researchers in the field of behavioral economics. Indeed, the ways in which our brains are biased may be well suited to the environment of our ancestors, when a fast decision was often better than no decision at all. But the hypercompetitive and fast-paced world of business today requires short response times and more accurate responses and more exploration of potential opportunities.
The good news is that, thanks to the Internet and other information technologies, we now have access to more data — sometimes much more data — about customers, employees and other stakeholders so that, in principle, we can gain a more accurate and intimate understanding of our environment. But that’s not enough; decisions still need to be made. We must explore the data so that we can discover opportunities, evaluate them and proceed accordingly. The problem is that our limitations as individual decision makers have left us ill equipped to solve many of today’s demanding business problems. What if, though, we relied more on others to find those solutions?
To be sure, companies have long used teams to solve problems, focus groups to explore customer needs, consumer surveys to understand the market and annual meetings to listen to shareholders. But the words “solve,” “explore,” “understand” and “listen” have now taken on a whole new meaning. Thanks to recent technologies, including many Web 2.0 applications,1 companies can now tap into “the collective” on a greater scale than ever before. Indeed, the increasing use of information markets, wikis, “crowdsourcing,” “the wisdom of crowds” concepts, social networks, collaborative software and other Web-based tools constitutes a paradigm shift in the way that companies make decisions.2 Call it the emerging era of “Decisions 2.0.”
But the proliferation of such technologies necessitates a framework for understanding what type of collective intelligence is possible (or not), desirable (or not) and affordable (or not) — and under what conditions. This article is intended to provide a general framework and overview for helping companies to assess how they might use Decisions 2.0 applications to solve problems and make better decisions.3
A Decision Framework
In the field of operations research, solving a problem entails two high-level tasks: 1) generating solutions, which includes framing the problem and establishing a set of working assumptions about it and 2) evaluating the different alternatives generated in the first step. Each of the tasks is subject to various biases.4 When generating solutions, for example, we tend to seek information that confirms our assumptions (self-serving bias) and to maintain those beliefs even in the face of contrary evidence (belief perseverance). With respect to the evaluation of solutions, we tend to see patterns where none exist (pattern obsession) and to be unduly influenced by how a solution is presented (framing). Those common traps represent just a few from among a much larger number of ways in which our basic human nature can lead us astray when we’re making important decisions.
Collective intelligence can help mitigate the effects of those biases. (See “Using Collective Intelligence to Make Better Decisions.”) For instance, it can provide a diversity of viewpoints and input that can deter self-serving bias and belief perseverance. Diversity can also help combat pattern obsession and negative framing effects. Because of those and other benefits, many companies have begun to tap into collective intelligence through the use of Web 2.0 and other technologies. Some of the applications concentrate on solution generation. Take, for example, InnoCentive, a Web site through which companies can post a problem and solicit solutions, with the winning entry receiving a cash prize.5 Other applications focus on the evaluation task. HSX, for instance, is a mock market in which users buy and sell futures for current movies, and the results are an indicator of the moneymaking potential of those releases. And still other applications address both generation and evaluation. The Digg Web site asks participants both to contribute stories and to vote on them, and the most popular entries are posted on the home page.
Whether the goal is solution generation, evaluation or both, companies should consider three general types of approach to accomplish their objective: outreach, additive aggregation and self-organization. Each has its pros and cons, and many Decisions 2.0 applications have combined the different approaches in various ways.
1. Outreach When collecting ideas (generation) or assessing them (evaluation), a company might want to tap into people or groups that haven’t traditionally been included. It might, for instance, want to reach across hierarchical or functional barriers inside the organization, or it could even desire to obtain help from the outside. The value of outreach is in numbers: broadening the number of individuals who are generating or evaluating solutions. The development of open-source software is perhaps the best example of the power of sheer numbers. “With enough eyeballs, all bugs are shallow” is the commonly quoted expression, which means that, with enough people working on a project, they will uncover every mistake. The underlying philosophy here is that there are people out there who can help you and, moreover, those individuals are not necessarily where you might expect them to be. At InnoCentive, the solutions to problems often come from an entirely different field.6
2. Additive aggregation Companies can collect information from myriad sources and then perform some kind of averaging. The process can be used to aggregate data from traditional decision groups, or it can also be combined with outreach to include information from a broader set of people. Here, the whole is by definition equal to the sum of its parts (or some average of it). The simplest example involves the direct application of the law of large numbers — for example, asking a crowd to estimate the number of jelly beans in a jar and then taking an average of all the responses. More complex examples involve applications like information, or prediction, markets. The key here is to maintain the right balance between diversity and expertise. Both are needed in varying degrees, depending on the application. At Best Buy Co. Inc.,7 for example, internal information markets are used for a number of forecasting activities. A number of those tasks have been successful, but the internal market tends to perform poorly when asked questions about the competition, which is apparently a topic about which Best Buy employees have limited knowledge.
3. Self-organization Mechanisms that enable interactions among group members can result in the whole being more than the sum of its parts.8 Examples of such constructive uses of self-organization in which the interactions create additional value include Wikipedia, Intellipedia (the CIA’s version of Wikipedia for the intelligence community) and Digg. Such applications enable people to create value by adding to or deleting from other participants’ contributions. But there is a danger: If the interaction mechanisms are not designed properly, the whole can end up being much less than the sum of its parts. Groupthink is but one example of the downside of self-organization.
Key Issues
An application that taps into collective intelligence for improved decision making may be simple in concept, but it can be extremely difficult to implement. As with many systems, the devil is definitely in the details. At a minimum, managers need to consider the following important issues.
Control One key concern, common to all forms of collective intelligence, is a loss of control, which can manifest itself in a variety of ways. One is, simply, unwanted and undesirable outcomes — the collective makes a decision that could harm the company, revealing either a flaw in managers’ thinking or the improper application of collective intelligence. Another is unpredictability — a decision might not necessarily be bad per se, but the organization is caught unprepared to deal with it. A third is unassigned liability — who is responsible for a poor decision made collectively? Moreover, companies need to be aware of the potential for snowball effects. Through self-
organization, an opinion might gain nonlinear momentum through self-amplification. This can lead to public relations nightmares if the collective involves participants external to the organization. Consequently, one of the biggest issues with respect to control is whether to include outsiders in the process.
The choice to expand your decision-maker set beyond the walls of your organization should not be made lightly. Not only will you be disclosing information about your organization to the external world, you’ll also be providing a forum for outsiders who might not always have your best interests at heart. If the collective veers in an unexpected and potentially harmful direction, the resulting damage could be difficult (and costly) to contain. Once the genie is out of the bottle, it can’t be put back in. On the other hand, tapping into the diversity and expertise of the outside world can lead to substantially superior outcomes when executed properly.
Diversity versus expertise As mentioned earlier, decision making that makes use of a collective requires a company to strike the right balance between diversity and expertise. Certain problems lend themselves to a diversity-based approach more than others, but no amount of diversity will help if the participants are completely ignorant of the issues.9 Another factor to consider is the actual composition of diversity: In the same way that sampling biases exist in polls, the diversity of a large population can also be skewed, leading to distorted decisions. Thus, organizations need to decide which people to involve based on the ability of those individuals to understand the problem at hand and collectively make positive contributions to solving it.
Consider the cautionary tale of Pallokerho-35 (PK-35), a Finnish soccer club. A few years ago, the team’s coach invited fans to help determine the club’s recruiting, training and even game tactics by allowing them to vote using their cell phones.10 Unfortunately, the season was a disaster, ending with PK-35 firing its coach and scrapping its fan-driven decision-making ways. The lesson here is that many applications do require a large number of participants to ensure the quality of the output, but those individuals must still have the necessary knowledge to make useful contributions.
Other decisions require a significant amount of expertise that is found only in a handful of people, either inside or outside the organization. For such situations, traditional tools like the Delphi method, a systematic and iterative approach to forecasting, can help foster quality decisions. Some companies, though, are investigating the use of newer approaches. HP Labs, for example, has designed an intriguing solution for tapping the collective brainpower of a small group using a prediction market. The approach extracts the participants’ risk profiles with a simple game and then uses that information to adjust the market’s behavior.
Engagement What motivates people to participate in a collective undertaking can vary widely. Incentives such as cash rewards, prizes and other promotions can be effective in stimulating individuals to participate in activities like prediction markets, for which explicit rewards seem to matter greatly. With other applications — for example, submitting T-shirt designs to the Threadless Web site — cash rewards seem to matter less than recognition. Value-driven incentives can also be important. As the open-source movement, Wikipedia and other similar efforts have shown, participation in a community, the desire to transfer knowledge or share experiences, and a sense of civic duty can be powerful motivators. For ongoing internal efforts, maintaining a high level of employee engagement can be difficult. At Google, Inc., new employees are the most excited about — and engaged in — the organization’s internal prediction markets, but their enthusiasm decreases over time. Thus, organizations must provide a continuous flow of new, enthusiastic participants to keep engagement high, or they need to provide incentives to sustain people’s motivation over time.
Policing When people are allowed to contribute to decisions, the likelihood that some will misbehave increases with group size. To control such transgressions, mutual policing can be effective in situations for which an implicit code of conduct helps govern people’s behavior. But concern for one’s reputation can also have a negative effect, leading to excessively conservative decisions, as participants become unduly worried about being wrong. For some collective activities such as prediction markets, a central authority akin to the Securities and Exchange Commission might be necessary to thwart any attempts at market manipulation.
Intellectual property Another concern is that of intellectual property, and this issue manifests itself in two ways. The most obvious is that a company needs to disclose information about its problems to get others to think about them, and adjusting to and managing such transparency can be very difficult for many businesses, particularly those that have kept close wraps on their intellectual property. Second, when a company seeks ideas from outside the organization, it needs to determine whether and how it will assume ownership of the resulting intellectual property. (It also needs to ensure that the intellectual property is the participant’s to give.)
Mechanism Design
Perhaps the most difficult issue of all is mechanism design, which must address numerous basic questions. For instance, should every participant be given an equal voice, or should some individuals have a greater say in the collective? And if it’s the latter, how should those special individuals be selected? Indeed, designing the right mechanisms for collective decision making is neither simple nor straightforward, and the “rules of engagement” can make an enormous difference in the outcome.
Another basic question regarding mechanism design is whether to utilize distributed versus decentralized decision making. In distributed decision making, which is the most familiar form of collective intelligence, a number of people contribute to one decision. In decentralized decision making, many people are empowered to make their own independent decisions. Consider Web sites like ManyEyes and Swivel, where participants can upload data sets for others to explore with easy-to-use tools. Say someone uploads carbon-dioxide data for a particular geographic area and someone else uploads housing development information for that same neighborhood, a third person could then study those two sets of data to investigate whether they were correlated. Numerous examples of disaster response, from the 2004 tsunami to hurricane Katrina, have demonstrated the power of decentralized decision making by people on the front lines.11 In such situations, sophisticated plans devised by central bureaucrats tend to fall apart. The lesson is that decisions made at the head office may not fit local or field realities. Harnessing the collective intelligence of those who have the necessary information for the benefit of those who must take action in the field can be a surer path to success than the use of top-down, template-based decisions. For such situations, the organization can aid the decision-making process by effectively becoming a broker of information.
Up to now, collective decision making has largely been empirically driven, and for every success story like Wikipedia, there are likely numerous projects that have failed because of faulty mechanism designs. And even an application like Wikipedia, which might look simple on the surface, relies on a complex hierarchy of carefully selected editors. Even small changes to the design of a successful mechanism can lead to large, unintended negative consequences. As a result, some organizations have decided to replicate an application exactly. A case in point is Intellipedia, a tool developed for the U.S. intelligence community that is a direct copy of the Wikipedia design.
Defining Success
Companies have implemented Decisions 2.0 applications for a variety of purposes, including research and development, market research, customer service and knowledge management. Obviously, the type of application will affect how its success should be assessed, but managers should remember that certain key metrics can only be determined late in the process, if not at its very end. For example, the accuracy of a forecast generated by an information market can be measured only after the event has occurred. In such cases, managers should consider using key indicators to serve as proxies to assess an application before a final evaluation can be made. (See “Does Collective Intelligence Lead to Better Decisions?”) In an information market, for instance, key indicators might be the sheer number and demographic diversity of the participants.
Although both the key metrics and indicators can vary depending on the application itself, one universal key indicator is engagement — whether the application has stimulated and maintained the active participation of people in a meaningful way. Engagement should not to be taken lightly. Indeed, for a large fraction of Decisions 2.0 projects that have flopped, the primary cause of failure appears to be a lack of engagement. Participants expect to be treated in a certain way and, more often than not, they also want the organizers of the application to be engaged as well.
Generally speaking, the performance of many Decisions 2.0 applications has been less than optimal for a number of reasons. For one thing, many tools do not provide information on the participants, which then raises concerns about the accuracy of the output and the possibility that the process might be vulnerable to manipulation. In addition, the applications often lack any explicit refereeing process that might provide some degree of quality assurance.12 On the other hand, even applications that have a massive number of participants can be managed successfully. Wikipedia might be the most well-known example of that, but there are many others. Take, for example, Delicious, a tool that allows users to tag Web bookmarks with specific keywords, thus providing useful metadata about those sites.
But a pressing question remains: Does collective intelligence really correct for decision biases? There is no simple answer. Before the 2006 U.S. elections, the Intrade Web site correctly predicted that the Democrats would gain control over both houses of Congress — something many political pundits and expert prognosticators had failed to foresee. But then in 2008, the company discovered that a lone member of the prediction market had single-handedly been responsible for an unusual spike in the prediction that Senator John McCain would beat Senator Barack Obama in the presidential election. In a number of other documented cases, the collective decision-making process was shown to be biased. With information markets, for example, those biases can manifest themselves in all the ways they do for individual decision making: pattern obsession, framing and so on.
That said, Decisions 2.0 applications have generally worked better in practice than the available theories might have predicted. As an early contributor to the field of collective intelligence, I was certain that Wikipedia would fail. Why it works as well as it does is still largely a mystery to me. Although I have come to appreciate the degree to which people want to express themselves, the quality of Wikipedia (and of many of its offspring) is still surprising. Today, with the one notable exception of prediction markets (for which a large body of work helps us understand what works and why), practice is still far ahead of theory in the field of collective intelligence.
But the fact that we can’t explain the success of many Decisions 2.0 initiatives is not necessarily a bad thing. In fact, we use many tools on a daily basis that we don’t fully understand — our intuitive minds, for example. Even so, advances in research and theory — and the development of better success metrics — should lead to substantial improvements in the practical design and implementation of various Decisions 2.0 applications. To that end, efforts are under way by several groups of researchers, including those at HP Laboratories and International Business Machines Corp. Research Division, to understand the dynamics of successful applications like Digg and Wikipedia.
Even given the current lack of theory, a survey of the different applications leads me to two general observations. First, collective intelligence tends to be most effective in correcting individual biases in the overall task area of generation. I speculate that we, as individuals, are far weaker explorers than evaluators, and that, for all the flaws in our heuristics, we are pretty good at detecting patterns. Thus, when tapping a collective, companies are now more likely to obtain greater value from idea generation than from idea evaluation.
Second, a striking feature of most applications is that feedback loops between generation and evaluation tend to be weak or nonexistent. Here, the fundamental mechanism of variation and selection from biological evolution could provide an effective model: Ideas could be generated and evaluated, and the output of that assessment could be used in the creation of the next generation of ideas. The market research firm Affinnova, Inc., for instance, uses collective feedback from consumers to create a new generation of product designs that are then submitted back to the collective for evaluation. Companies should consider deploying such feedback loops with greater frequency because the iterative process taps more fully into the power of a collective.
That collective intelligence is at all possible is not a new idea. Until recently, though, Charles Mackay’s 1841 book, Extraordinary Popular Delusions and the Madness of Crowds, was the default framework for the collective at work. According to it, the most likely outcome of collective human dynamics is market bubbles, instability and chaos. And indeed, as demonstrated by the TV show “American Idol” and Google’s Zeitgeist, which displays the most frequent search queries, the crowd does not necessarily have good taste. Nor is the crowd any better at solving certain types of problems than experts are.
But a growing number of applications have shown that a group of diverse, independent and reasonably informed people might outperform even the best individual estimate or decision. Indeed, James Surowiecki’s 2004 book, The Wisdom of Crowds (a play on the title of Mackay’s classic tome) offers a collection of such examples.14 At the very least, the emergence of Web-based tools for bringing people together in a variety of formats has made it possible to experiment with a number of different mechanisms for tapping into the decision-making capabilities of the collective. Moreover, the wide availability of Web 2.0 applications has led to the increasing emergence of professional amateurs: From ornithologists to photographers, people who previously had the passion but no tools are now empowered with technology that enables them to perform at the same level as professionals.15
The bottom line is this: For many problems that a company faces, there is potentially a solution out there, far outside of the traditional places that managers might search, within or outside the organization. The trick, though, is to develop the right tool for locating that source and then tapping into it. Indeed, although a success like Wikipedia might look simple on the surface, that superficial simplicity belies a complex underlying mechanism for harnessing the power of collective intelligence. Consequently, any company that is developing a Decisions 2.0 application would do well to understand some fundamental issues, such as the balance between diversity and expertise, and the distinction between decentralized and distributed decision making. After all, without such basic knowledge, a business could easily end up tapping into a crowd’s madness — and not its wisdom.
References
1. A.P. McAfee, “Enterprise 2.0: The Dawn of Emergent Collaboration,” MIT Sloan Management Review 47, no. 3 (Spring 2006): 21-28; and S. Cook, “The Contribution Revolution: Letting Volunteers Build Your Business,” Harvard Business Review 86 (October 2008): 60-69.
2. J. Howe, “Crowdsourcing: Why the Power of the Crowd Is Driving the Future of Business” (New York: Crown Business, 2008); D. Bollier, “The Rise Of Collective Intelligence: Decentralized Co-Creation of Value as a New Paradigm of Commerce and Culture,” A Report of the Sixteenth Annual Aspen Institute Roundtable on Information Technology (Washington, D.C.: The Aspen Institute, 2007); C. Li and J. Bernoff, “Groundswell: Winning in a World Transformed by Social Technologies” (Boston: Harvard Business School Press, 2008); B. Libert and J. Spector, “We Are Smarter Than Me: How to Unleash the Power of Crowds in Your Business” (Upper Saddle River, New Jersey: Pearson Education, Wharton School Publishing, 2007); A. Shuen, “Web 2.0: A Strategy Guide: Business Thinking and Strategies Behind Successful Web 2.0 Implementations” (Sebastopol, California: O’Reilly Media, 2008); T.W. Malone, “The Future of Work: How the New Order of Business Will Shape Your Organization, Your Management Style and Your Life” (Boston: Harvard Business School Press, 2004); and E. von Hippel, “Democratizing Innovation” (Cambridge, Massachusetts: MIT Press, 2005).
3. A much more exhaustive coverage of the field, as well as a very good taxonomy of collective-intelligence applications, can be found at the MIT Center for Collective Intelligence (www.cci.mit.edu).
4. D.G. Myers, “Intuition: Its Powers and Perils” (New Haven, Connecticut: Yale University Press, 2004).
5. K.R. Lakhani and J.A. Panetta, “The Principles of Distributed Innovation,” Innovations: Technology, Governance, Globalization 2, no. 3 (2007): 97-112.
6. Ibid.
7. J. Surowiecki, “The Wisdom of Crowds: Why the Many Are Smarter Than the Few and How Collective Wisdom Shapes Business, Economies, Societies and Nations” (New York: Doubleday, 2004); and R. Dye, “The Promise of Prediction Markets: A Roundtable,” McKinsey Quarterly, no. 2 (2008): 83-93.
8. E. Bonabeau and C. Meyer, “Swarm Intelligence: A Whole New Way to Think About Business,” Harvard Business Review 79 (May 2001): 106-114.
9. S.E. Page, “The Difference: How the Power of Diversity Creates Better Groups, Firms, Schools, and Societies” (Princeton, New Jersey: Princeton University Press, 2007).
10. I. Wylie, “Who Runs This Team, Anyway?” Fast Company (March 2002).
11. D.W. Stephenson and E. Bonabeau, “Expecting the Unexpected: The Need for a Networked Terrorism and Disaster Response Strategy,” Homeland Security Affairs III (2007): 1-8; and C.H. Shirky, “Here Comes Everybody: The Power of Organizing Without Organizations” (New York: The Penguin Press, 2008).
12. D.E. O’Leary, “Wikis: ‘From Each According to His Knowledge,’” Computer (February 2008): 34-41.
13. D. Tapscott and A.D. Williams, “Wikinomics: How Mass Collaboration Changes Everything” (New York: Portfolio, 2008).
14. Surowiecki, “The Wisdom of Crowds.”
15. Howe, “Crowdsourcing”; and C.R. Sunstein, “Infotopia: How Many Minds Produce Knowledge” (New York: Oxford University Press, 2006).
Comments (2)
Leslie Brokaw
Ben Towne