Reshaping Business With Artificial Intelligence
Closing the Gap Between Ambition and Action
Executive Summary
Expectations for artificial intelligence (AI) are sky-high, but what are businesses actually doing now? The goal of this report is to present a realistic baseline that allows companies to compare their AI ambitions and efforts. Building on data rather than conjecture, the research is based on a global survey of more than 3,000 executives, managers, and analysts across industries and in-depth interviews with more than 30 technology experts and executives. (See “About the Research.”)
The gap between ambition and execution is large at most companies. Three-quarters of executives believe AI will enable their companies to move into new businesses. Almost 85% believe AI will allow their companies to obtain or sustain a competitive advantage. But only about one in five companies has incorporated AI in some offerings or processes. Only one in 20 companies has extensively incorporated AI in offerings or processes. Less than 39% of all companies have an AI strategy in place. The largest companies — those with at least 100,000 employees — are the most likely to have an AI strategy, but only half have one.
Our research reveals large gaps between today’s leaders — companies that already understand and have adopted AI — and laggards. One sizeable difference is their approach to data. AI algorithms are not natively “intelligent.” They learn inductively by analyzing data. While most leaders are investing in AI talent and have built robust information infrastructures, other companies lack analytics expertise and easy access to their data. Our research surfaced several misunderstandings about the resources needed to train AI. The leaders not only have a much deeper appreciation about what’s required to produce AI than laggards, they are also more likely to have senior leadership support and have developed a business case for AI initiatives.
AI has implications for management and organizational practices. While there are already multiple models for organizing for AI, organizational flexibility is a centerpiece of all of them. For large companies, the culture change required to implement AI will be daunting, according to several executives with whom we spoke.
Our survey respondents and interviewees are more sanguine than conventional wisdom on job loss. Most managers we surveyed do not expect that AI will lead to staff reductions at their organization within the next five years. Rather, they hope that AI will take over some of their more boring and unpleasant current tasks.
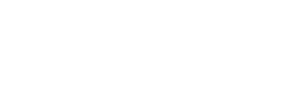
AI at Work
As Airbus started to ramp up production of its new A350 aircraft, the company faced a multibillion-euro challenge. In the words of Matthew Evans, vice president of digital transformation at the Toulouse, France-based company, “Our plan was to increase the production rate of that aircraft faster than ever before. To do that, we needed to address issues like responding quickly to disruptions in the factory. Because they will happen.”
Airbus turned to artificial intelligence. It combined data from past production programs, continuing input from the A350 program, fuzzy matching, and a self-learning algorithm to identify patterns in production problems. In some areas, the system matches about 70% of the production disruptions to solutions used previously — in near real time. Evans describes how AI enables the entire Airbus production line to learn quickly and meet its business challenge:
What the system does is essentially look at a problem description, taking in all of the contextual information, and then it matches that with the description of the issue itself and gives the person on the floor an immediate recommendation. The problem might be new to them, but in fact, we’ve seen something very similar in the production line the weekend before, or on a different shift, or on a different section of the line. This has allowed us to shorten the amount of time it takes us to deal with disruptions by more than a third.
AI empowered Airbus to solve a business problem more quickly and efficiently than prior approaches (such as root-cause analysis based on manual analysis of hundreds or thousands of cases).
Just as it is enabling speed and efficiency at Airbus, AI capabilities are leading directly to new, better processes and results at other pioneering organizations. Other large companies, such as BP, Infosys, Wells Fargo, and Ping An Insurance, are already solving important business problems with AI. Many others, however, have yet to get started.
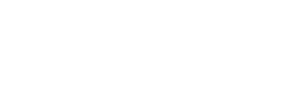
High Expectations Amid Diverse Applications
Expectations for AI run high across industries, company sizes, and geography. While most executives have not yet seen substantial effects from AI, they clearly expect to in the next five years. Across all organizations, only 14% of respondents believe that AI is currently having a large effect (a lot or to a great extent) on their organization’s offerings. However, 63% expect to see these effects within just five years.
Expectations for Change Across Industries and Within Organizations
Expectations for AI’s effects on companies’ offerings are consistently high across industry sectors. (See Figure 1.) Within the technology, media, and telecommunications industry, 72% of respondents expect large effects from AI in five years, a 52-percentage-point increase from the number of respondents currently reporting large effects. However, even in the public sector — the industry with the lowest overall expectations for AI’s effects — 41% of respondents expect large effects from AI within five years, an increase of 30 percentage points from current levels. This bullishness is apparent regardless of the size or geography of the organization.
Within organizations, respondents report similarly high expectations for the large effects of AI on processes. While 15% of respondents reported a large effect of AI on current processes, over 59% expect to see large effects within five years. (See Figure 2.) Most organizations foresee sizable effects on information technology, operations and manufacturing, supply chain management, and customer-facing activities. (See Figure 3.) For example:
Information technology: Business process outsourcing providers serve as an example of the potential of AI. “IT services, where Infosys plays a big role, has seen tremendous growth in the last 20 or so years,” says Infosys Ltd. CEO and managing director Vishal Sikka.1 “Many jobs that moved to low labor-cost countries were the ones that were more mechanical: system administration, IT administration, business operations, verification. With AI techniques, we now have systems that can do more and more of those kinds of jobs. We are still in the early stages and portions of these activities can be automated, but we will get to the point in the next few years where the majority if not all of these jobs will be automated. However, just as AI technologies automate existing, well-defined activities, they also create opportunities for new, breakthrough kinds of activities that did not exist.”
Operations and manufacturing: Executives at industrial companies expect the largest effect in operations and manufacturing. BP plc, for example, augments human skills with AI in order to improve operations in the field. “We have something called the BP well advisor,” says Ahmed Hashmi, global head of upstream technology, “that takes all of the data that’s coming off of the drilling systems and creates advice for the engineers to adjust their drilling parameters to remain in the optimum zone and alerts them to potential operational upsets and risks down the road. We are also trying to automate root-cause failure analysis to where the system trains itself over time and it has the intelligence to rapidly assess and move from description to prediction to prescription.”
Customer-facing activities: Ping An Insurance Co. of China Ltd., the second-largest insurer in China, with a market capitalization of $120 billion, is improving customer service across its insurance and financial services portfolio with AI. For example, it now offers an online loan in three minutes, thanks in part to a customer scoring tool that uses an internally developed AI-based face-recognition capability that is more accurate than humans. The tool has verified more than 300 million faces in various uses and now complements Ping An's cognitive AI capabilities including voice and imaging recognition.
Adoption as Opportunity and Risk
While expectations for AI run high, executives recognize its potential risks. Sikka is optimistic but cautions against hyping AI’s imminent triumph: “If you look at the history of AI since its origin in 1956, it has been a story of peaks and valleys, and right now we are in a particularly exuberant time where everything looks like there is one magnificent peak in front of us.” More than 80% of the executives surveyed are eyeing the peaks and view AI as a strategic opportunity. (See Figure 4.) In fact, the largest group of respondents, 50%, consider AI to be only an opportunity. Some see risks and the potential for increased competition from AI as well as benefits. Almost 40% of managers see AI as a strategic risk as well. A much smaller group (13%) does not view AI as either an opportunity or risk.
What is behind these high expectations and business interest in AI? There is no single explanation. (See Figure 5.) Most respondents believe that AI will benefit their organization, such as through new business or reduced costs; 84% believe Al will allow their organization to obtain or sustain a competitive advantage. Three in four managers think AI will allow them to move into new businesses.
Executives simultaneously recognize that their organization will not likely be the sole beneficiary of AI in their markets. Respondents expect that both new entrants and incumbents would similarly see the potential for benefits. Three-quarters of respondents foresee new competitors using AI to enter their markets while 69% expect current competitors to adopt AI in their businesses. Furthermore, they realize that suppliers and customers in their business ecosystem will increasingly expect them to use AI.
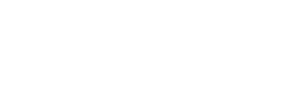
Disparity in Adoption and Understanding
Despite high expectations, business adoption of AI is at a very early stage: There is a disparity between expectation and action. Although four in five executives agree that AI is a strategic opportunity for their organization, only about one in four has incorporated AI in some offerings or processes. Only one in 20 has extensively incorporated AI in their offerings or processes. (See Figure 6.)
The differences in adoption can be striking, particularly within the same industry. For example, Ping An, which employs 110 data scientists, has launched about 30 CEO-sponsored AI initiatives that support, in part, its vision “that technology will be the key driver to deliver top-line growth for the company in the years to come,” says the company’s chief innovation officer, Jonathan Larsen. Yet in sharp contrast, elsewhere in the insurance industry, other large companies’ AI initiatives are limited to “experimenting with chatbots,” as a senior executive at a large Western insurer describes his company’s AI program.
Organizations also report significant differences in their overall understanding of AI. For example, 16% of respondents strongly agreed that their organization understands the costs of developing AI-based products and services. And almost the same percentage (17%) strongly disagreed that their organization understands these costs. Similarly, while 19% of respondents strongly agreed that their organization understands the data required to train AI algorithms, 16% strongly disagreed that their organization has that understanding.
Combining survey responses to questions around AI understanding and adoption, four distinct organizational maturity clusters emerged: Pioneers, Investigators, Experimenters, and Passives.2
- Pioneers (19%): Organizations that both understand and have adopted AI. These organizations are on the leading edge of incorporating AI into both their organization’s offerings and internal processes.
- Investigators (32%): Organizations that understand AI but are not deploying it beyond the pilot stage. Their investigation into what AI may offer emphasizes looking before leaping.
- Experimenters (13%): Organizations that are piloting or adopting AI without deep understanding. These organizations are learning by doing.
- Passives (36%): Organizations with no adoption or much understanding of AI.
If expectations and sense of opportunity are so high, what prevents organizations from adopting AI? Even in industries with extensive histories of integrating new technologies and managing data, barriers to AI adoption can be difficult to overcome. In financial services, for example, Simon Smiles, chief investment officer, ultra high net worth at UBS, puts it this way: “The potential for larger-scale financial institutions to leverage technology more actively, including artificial intelligence, within their business, and to harness their data to deliver a better client experience to the end user, is huge. The question there is whether these traditional institutions will actually grab the opportunity.” Taking advantage of AI opportunities requires organizational commitment to get past the inevitable difficulties that accompany many AI initiatives.
These differences are less about technological limitations and much more about business. In aggregate, respondents ranked competing investment priorities and unclear business cases as more significant barriers to AI implementation than technology capabilities. Evans of Airbus makes the critical distinction: “Well, strictly speaking, we don’t invest in AI. We don’t invest in natural language processing. We don’t invest in image analytics. We’re always investing in a business problem.” Airbus turned to AI because it solved a business problem; it made business sense to invest in AI instead of other approaches.
Smiles at UBS notes that organizations do not all face the same challenges. With respect to incumbents and fintech startups, he says: “There is a bifurcation between the groups that have the scale needed to develop incredibly valuable platforms and those unencumbered by legacy business models and systems to arguably have the better model going forward, but don’t have the clients and accompanying data to capitalize fully on the opportunity.” Differences like these lead to differences in rates of AI adoption.
Barriers to Adoption
The clusters of organizations demonstrate how barriers to AI differ and affect rates of adoption. (See Figure 7.) Pioneers have overcome issues related to understanding: three-quarters of these companies have identified business cases for AI. Senior executives are leading organizational AI initiatives. Their biggest hurdles are grappling with the practicalities of developing or acquiring the requisite AI talent and addressing competing priorities for AI investment. They are also much more likely to be attuned to the security concerns resulting from AI adoption. Passives, by contrast, have yet to come to grips with what AI can do for them. They have not identified solid business cases that meet their investment criteria. Leadership may not be on board. Technology is a hurdle. Many are not yet even aware of the difficulties in sourcing and deploying talent with AI expertise.
Our clustering also reveals nuanced differences in understanding among the clusters.
- Business potential: AI may change how organizations create business value. Pioneers (91%) and Investigators (90%) are much more likely to report that their organization recognizes how AI affects business value than Experimenters (32%) and Passives (23%). Evans at Airbus reports that “there was no question of value; it was trying to address an in-service issue on one of our aircraft.”
- Workplace implications: Integrating the capabilities of humans and machines is a looming issue. AI stands to change much of the daily work environment. Pioneers and Investigators better appreciate that the presence of machines in the workplace will change behavior within the organization. Julie Shah, an associate professor of aeronautics at MIT, says, “What people don’t talk about is the integration problem. Even if you can develop the system to do very focused, individual tasks for what people are doing today, as long as you can’t entirely remove the person from the process, you have a new problem that arises — which is coordinating the work of, or even communication between, people and these AI systems. And that interaction problem is still a very difficult problem for us, and it’s currently unsolved.”
- Industry context: Organizations operate in regulatory and industry contexts; respondents from Experimenter and Passive organizations do not feel that their organization appreciates how AI may affect industry power dynamics.
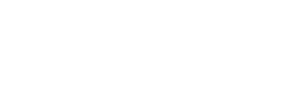
The Need for Data, Training, and Algorithms
Perhaps the most telling difference among the four maturity clusters is in their understanding of the critical interdependence between data and AI algorithms. Compared to Passives, Pioneers are 12 times more likely to understand the process for training algorithms, 10 times more likely to understand the development costs of AI-based products and services, and 8 times more likely to understand the data that’s needed for training AI algorithms. (See Figure 8.)
Most organizations represented in the survey have little understanding of the need to train AI algorithms on their data so they can recognize the sort of problem patterns that Airbus’s AI application revealed. Less than half of respondents said their organization understands the processes required to train algorithms or the data needs of algorithms.
Generating business value from AI is directly connected to effective training of AI algorithms. Many current AI applications start with one or more “naked” algorithms that become intelligent only upon being trained (predominantly on company-specific data). Successful training depends on having well-developed information systems that can pull together relevant training data. Many Pioneers already have robust data and analytics infrastructures along with a broad understanding of what it takes to develop the data for training AI algorithms. Investigators and Experimenters, by contrast, struggle because they have little analytics expertise and keep their data largely in silos, where it is difficult to integrate. While over half of Pioneer organizations invest significantly in data and training, organizations from the other maturity clusters invest substantially less. For example, only one-quarter of Investigators have made significant investments in AI technology, the data required to train AI algorithms, and processes to support that training.
Misunderstandings About Data for AI
Our research revealed several data-related misconceptions. One misunderstanding is that sophisticated AI algorithms alone can provide valuable business solutions without sufficient data. Jacob Spoelstra, director of data science at Microsoft, observes:
I think there’s still a pretty low maturity level in terms of people’s understanding of what can be done through machine learning. A mistake we often see is that organizations don’t have the historical data required for the algorithms to extract patterns for robust predictions. For example, they’ll bring us in to build a predictive maintenance solution for them, and then we’ll find out that there are very few, if any, recorded failures. They expect AI to predict when there will be a failure, even though there are no examples to learn from.
No amount of algorithmic sophistication will overcome a lack of data. This is particularly relevant as organizations work to use AI to advance the frontiers of their performance.
Some forms of data scarcity go unrecognized: Positive results alone may not be enough for training AI. Citrine Informatics, a materials-aware AI platform helping to accelerate product development, uses data from both published experiments (which are biased toward successful experiments) and unpublished experiments (which include failed experiments) through a large network of relationships with research institutions. “Negative data is almost never published, but the corpus of negative results is critical for building an unbiased database,” says Bryce Meredig, Citrine’s cofounder and chief science officer. This approach has allowed Citrine to cut R&D time in half for specific applications. W.L. Gore & Associates, Inc., developer of Gore-Tex waterproof fabric, similarly records both successful and unsuccessful results in its push to innovate; knowing what does not work helps it to know where to explore next.3
Sophisticated algorithms can sometimes overcome limited data if its quality is high, but bad data is simply paralyzing. Data collection and preparation are typically the most time-consuming activities in developing an AI-based application, much more so than selecting and tuning a model. As Airbus’ Evans says:
For every new project that we build, there’s an investment in combining the data. There’s an investment sometimes in bringing in new sources to the data platform. But we’re also able to reuse all of the work that we’ve done in the past, because we can manage those business objects effectively. Each and every project becomes faster. The upfront costs, the nonrecurring costs, of development are lower. And we’re able to, with each project, add more value and more business content to that data lake.
Pioneer organizations understand the value of their data infrastructure to fuel AI algorithms.
Additionally, companies sometimes erroneously believe that they already have access to the data they need to exploit AI. Data ownership is a vexing problem for managers across all industries. Some data is proprietary, and the organizations that own it may have little incentive to make it available to others. Other data is fragmented across data sources, requiring consolidation and agreements with multiple other organizations in order to get more complete information for training AI systems. In other cases, ownership of important data may be uncertain or contested. Getting business value from AI may be theoretically possible but pragmatically difficult.
Even if the organization owns the data it needs, fragmentation across multiple systems can hinder the process of training AI algorithms. Agus Sudjianto, executive vice president of corporate model risk at Wells Fargo & Co., puts it this way:
A big component of what we do is dealing with unstructured data, such as text mining, and analyzing enormous quantities of transaction data, looking at patterns. We work on continuously improving our customer experience as well as decision-making in terms of customer prospecting, credit approval, and financial crime detection. In all these fields, there are significant opportunities to apply AI, but in a very large organization, data is often fragmented. This is the core issue of the large corporation — dealing with data strategically.
Make Versus Buy
The need to train AI algorithms with appropriate data has wide-ranging implications for the traditional make-versus-buy decision that companies typically face with new technology investments. Generating value from AI is more complex than simply making or buying AI for a business process. Training AI algorithms involves a variety of skills, including understanding how to build algorithms, how to collect and integrate the relevant data for training purposes, and how to supervise the training of the algorithm. “We have to bring in people from different disciplines. And then, of course, we need the machine learning and AI people,” says Sudjianto. “Somebody who can lead that type of team holistically is very important.”
Pioneers rely heavily on developing internal skills through training or hiring. Organizations with less experience and understanding of AI put more emphasis on gaining access to outsourced AI-related skills, but this triggers some problems. (See Figure 9.)
The chief information officer of a large pharma company describes the products and services that AI vendors provide as “very young children.” The AI tech suppliers “require us to give them tons of information to allow them to learn,” he says, reflecting his frustration. “The amount of effort it takes to get the AI-based service to age 17, or 18, or 21 does not appear worth it yet. We believe the juice is not worth the squeeze.”
To be sure, for some support functions, such as IT management and payroll support, companies might choose to outsource the entire process (and pass along all of their data). Even if companies expect to rely largely on external support, they need their own people who know how to structure the problem, handle the data, and stay aware of evolving opportunities. “Five years ago, we would have leveraged labor arbitrage arrangements with large outsourcers to access lower cost human labor to do that work,” the pharma company CIO says. “What the vendors have done in the meantime is begin to automate those processes, oftentimes on our systems using our infrastructure, but using their technology. And I would not want it to be characterized as just rule-based. They actually have quite a bit more sophisticated heuristics to automate those things.” But such an approach is clearly not suited for companies’ distinctive offerings or core processes.
Eric Horvitz, director of Microsoft Research, argues that the tech sector is quickly catching up with the new model of offering technology tools to use with proprietary data, or “providing industry with toolsets, computation, and storage that helps to democratize AI.” Many AI algorithms and tools are already in the public domain, including Google’s TensorFlow, GitHub, and application programming interfaces from tech vendors. According to Horvitz:
Because this is a competitive space now in itself, the tools are getting easier to use and people that are there to help sell, market, and use these tools are becoming more efficacious in their abilities. That doesn’t mean that people don’t need to have their own in-house expertise and experts. While the tools and services are out there and that will make things easier, it is still going to be important for organizations to have their own experts in machine learning and AI more generally.
Privacy and Regulation
The data and the algorithms constituting AI cannot simply be accurate and high performing; they also need to satisfy privacy concerns and meet regulatory requirements. Yet only half the respondents in our survey agree that their industries have established data privacy rules.
Ensuring data privacy depends on having strong data governance practices. Pioneers (73%) are far more likely to have good data governance practices than the Experimenters (34%) and Passives (30%). (See Figure 10.) This large gap represents another barrier for companies that are behind in developing their AI capabilities.
The data issues can be pronounced in heavily regulated industries such as insurance, which is shifting from a historic model based on risk pooling toward an approach that incorporates elements that predict specific risks. But some attributes are off limits. For example, while sex and religion factors could be used to predict some risks, they are unacceptable to regulators in some applications and jurisdictions.
Regulators in other financial markets also have stringent transparency requirements. As Wells Fargo’s Sudjianto says: “Models have to be very, very transparent and checked by the regulators all the time. When we choose not to use machine learning as the final model, it’s because regulatory requirements oftentimes demand solutions be less ‘black box’ and something the regulator can see very clearly. But we use machine learning algorithms to assess the model’s non-linear construction, variables and features entered, and as a benchmark for how well the traditional model performs.”
As technology races ahead of consumer expectations and preferences, companies and the public sector tread an increasingly thin line between their AI initiatives, privacy protections, and customer service. Some financial services providers are using voice-recognition technology to identify customers on the phone to save time verifying identity. Customers welcome rather than balk at this experience, in part because they value the service and trust the company not to misuse the capability or the data that enables it. Likewise, a technology vendor offers an AI-based service to help call center operators recognize when customers are getting frustrated, using real-time sentiment analysis of voice data. Less welcome applications may be on the horizon, however. In a few years, any of the 170 million installed cameras in China or the 50 million cameras in the U.S. will be able to recognize faces. In fact, jaywalkers in Shanghai can already be fined (or shamed) based on such images.4
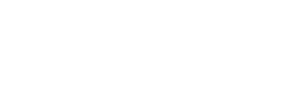
Beyond Technology: Management Challenges
AI requires more than data mastery. Companies also face many managerial challenges in introducing AI into their organizations.
Unsurprisingly, respondents at Pioneer organizations rate their companies higher in several general management and leadership areas: vision and leadership, openness and ability to change, long-term thinking, close alignment between business and technology strategy, and effective collaboration. As with other technology-driven transformations, these are essential general capabilities for high-performing companies.
However, there are also some specific challenges: Executives may still need to (1) learn more about AI; (2) deepen their perspective on how to organize their business around AI; and (3) develop a more expansive view of the competitive landscape in which their business operates.
Challenge 1: Develop an Intuitive Understanding of AI
The notion that executives and other managers need at least a basic understanding of AI is echoed by executives and academics. J.D. Elliott, director of enterprise data management at TIAA, a Fortune 100 financial services organization with nearly $1 trillion in assets under management, adds, “I don’t think that every frontline manager needs to understand the difference between deep and shallow learning within a neural network. But I think a basic understanding that — through the use of analytics and by leveraging data — we do have techniques that will produce better and more accurate results and decisions than gut instinct is important.” Avi Goldfarb, professor of marketing at the University of Toronto's Rotman School of Management, notes, “You worry that the unsophisticated manager might see one prediction work once and think that it’s always good, or see one prediction that was bad and think it’s always bad.” Joi Ito, head of the MIT Media Lab, contends that “every manager has to develop an intuitive understanding of AI.”5
To develop their understanding of digital, many executives have taken trips to Silicon Valley to experience digital natives, design-thinking approaches, fail-fast cultures, and more. While these are all core to building digital businesses, such trips are not particularly rewarding to learn about AI. For those who have already been exposed to the marvels of robots, self-driving vehicles, or poker-playing machines, there is little new to experience at AI companies. Instead, managers should take some time to learn the basics, possibly starting with simple online courses or online tools. They should understand how programs learn from data, maybe the most important facet of understanding how AI can benefit a particular business.
Challenge 2: Organize for AI
Adopting AI broadly across the enterprise will likely place a premium on soft skills and organizational flexibility that enable new forms of collaboration, including project teams composed of humans and machines.
Our survey finds companies exploring many approaches to developing AI capabilities. Pioneers are relatively evenly split among centralized, distributed, and hybrid organizational models. Investigators and Experimenters also pursue a mix of approaches, but almost 30% of both clusters have not yet set clear responsibility for AI in their organization. Some 70% of Passives also have not even started to lay out clear responsibilities for AI initiatives, perhaps (in part) because fewer than 50% of Passives see AI having a large effect on their processes and offerings in the next five years.
Ultimately, a hybrid model may make the most sense since many companies need AI resources both centrally and locally. TIAA, for example, has an analytics center of excellence and a number of decentralized groups. “The center of excellence is not intended to be the group that will provide all analytics for the entire organization. It provides expertise, guidance, and direction to other internal teams that are working to deploy AI and analytics,” says TIAA’s Elliott.
While companies in all four clusters rate cultural resistance to AI approaches relatively low on the list of barriers, only about half said that their company understands the required changes of knowledge and skills for future AI needs. Jessica Tan, group executive vice president, group chief operating officer, and chief information officer of Ping An, says the biggest challenges at her company have been getting units to work together; acknowledging the fact that “humans don’t want to train algorithms”; establishing centralized and decentralized technology teams; and finding the right people. It's looking in particular for three types of people: technical people who have the means to try different ways of working, technical people who understand specific business domains, and people with consulting or project management skills who are able to network and bring them all together.
Challenge 3: Re-think the Competitive Landscape
More than 60% of respondents say that a strategy for Al is urgent for their organizations, but only half of those say their organizations have a strategy in place. (See Figure 11.) In terms of company size, the largest companies (those with more than 100,000 employees) are the most likely to have an AI strategy, but only half (56%) have one.
Amy Hoe, chief technology and operations officer of insurer FWD Group, says that she sees access to data as key for competitive advantage for her company. FWD aims to secure a wide range of data sources, including partnerships with other companies, such as telecommunications companies and ride-hailing services, its customer base, agencies, social media, the public domain, and external data analysis providers. As the volume of data doubles every few years, gaining privileged access to data is nonstop work.
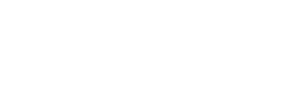
What to Do Next
Is AI just an element of a company’s overall digital transformation — or does AI require new approaches? On the one hand, AI presents many of the same issues and challenges as other digital technologies, and companies can build in many ways on their digital and analytics programs. However, AI also has distinctive features.
Ensure customer trust. AI capabilities are similar to many digital initiatives that depend on both customer data and customers’ trust that the company will respect and safeguard their personal data. Ensuring that AI is trustworthy is different from other data-dependent digital initiatives, however, in several ways. First, managers may not be able to explain exactly how a customer’s personal data is being used to produce a certain outcome from an AI product. The inner workings of some machine-learning programs are opaque. Second, a growing number of AI systems are able to mimic human agents, putting the onus on managers to clearly communicate to customers whether they are engaging with machines or human agents in a given setting. Third, some AI systems are able to assess emotions and discern quite personal details — at a distance. This capability creates new information management issues, including which employees have access to such information and under what circumstances.
Perform an AI health check. This has some similarities with digital health checks, from applications across processes to enabling infrastructure, technical skills, agile processes, and a fail-fast atmosphere. As with many digital initiatives, success with AI depends on access to data sources, be they existing internal or external data or investments in data infrastructure. Big companies may well have the data they need, but if it is fragmented and siloed, this significantly constrains strategy development and progress. Unlike other digital initiatives, an AI health check involves an assessment of the skills necessary to properly execute the training of AI, from first nurturing the system to become intelligent all the way to continuing to learn after deployment. This is both new and decisive — and a capability most companies need to build themselves. Off-the-shelf AI programs are likely to be limited in their capability and effect.
Brace for uncertainty. The adage “No idea is born good; you have to nurture it over time” applies to AI as well as to digital technologies — only more so. Companies often prioritize their initiatives by estimating the value of, and time required for, establishing a process or offering. But hard estimates are particularly difficult with AI. As a consequence, experimentation and learning with AI can take much longer than other digital initiatives, with a higher variability of success and failure. Managers need to brace themselves for more uncertainty, and this affects how effective they are at prioritizing projects and investments.
Adopt scenario-based planning. Like digital, AI has the potential to shift the ways in which businesses generate value — in multiple markets, processes, and functions. AI requires even more radical thinking, as it affects knowledge- and judgment-based professions, and the new entrants in markets could be machines. Thus, companies need to think even more expansively about their businesses, build cohesive future scenarios, and test the resilience of their directional plans against such scenarios.6 This kind of scenario-based planning can also sharpen the ability to recognize events that could trigger large effects on their business.
Add a workforce focus. AI stands to create significant unease, since even the most knowledgeable expert has difficulties specifying how programs will play out, which functions or processes should be off limits, or where AI should stop. The threat to jobs and careers in their current form is real and can easily lead to employee anxiety and unrest. Establishing a clear focus and work plan for AI initiatives — where they will be pursued and how, including regular communication, education, and training — should be a component of an AI program. Attracting and developing people who combine both business and technical skills will be critical, as will the ability to deploy cross-functional teams, requiring both individual and organizational flexibility.
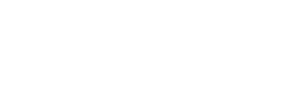
The Way Forward: Implications for the Future
The adoption of AI may have profound effects on the workplace, value creation, and competitive advantage. Beyond the near term, how should companies prepare for these changes?
The Future of Work
As AI is increasingly applied to knowledge work, a significant shift will likely take place in the workplace, affecting many jobs in the Western middle class. Contrary to recent dire predictions about AI’s effect on employment, our survey suggests cautious optimism. Most respondents, for example, do not expect that AI will lead to a reduction of jobs at their organization within the next five years. Nearly 70% also said they are not fearful that AI will automate their own jobs. By a similar margin, respondents hope that AI will take over some of their presumably boring and unpleasant current tasks. However, respondents overwhelmingly agree that AI will both require employees to learn new skills within the next five years and augment their existing skills. (See Figure 12.) Taken together, these portend adjustment, not annihilation. “Even with rapid advances,” says Erik Brynjolfsson, Schussel Family Professor at the MIT Sloan School of Management, “AI won’t be able to replace most jobs anytime soon. But in almost every industry, people using AI are starting to replace people who don’t use AI, and that trend will only accelerate.”7
Shifting Value Creation
Where will AI create, destroy, or shift economic value?
Consider the health care industry, one of the largest and most resilient sources of economic activity in the world. Health care spending makes up one-sixth of the U.S. economy, and on average, about one-tenth of the economies of Organisation for Economic Co-operation and Development (OECD) member nations. AI is already altering the health care value chain: Machines read diagnostic images, surgeons rely on robots, and an ever-increasing number of real-time medical devices contribute and communicate data to improve preventive and chronic care.
While AI may create value within an industry, it is far from clear exactly which organizations will see their fortunes rise and which will see decline. When IT vendors, medtech companies, radiologist networks, hospitals, specialized startups, and even insurance companies all strive to take advantage of AI to improve and lower the costs of diagnostics, the effects of AI will likely be uneven.
It’s too early to tell which types of organizations may benefit from AI in health care. But if regulatory concerns can be worked out, the industry has numerous sources of detailed data. And as Marcus Winter, head of reinsurance development at Munich Re Group, remarks, “In today’s world, with the proliferation of Big Data, there are precious few exclusive data sets. Most of the time, we can triangulate what we need to know via other sources.” In other words, the combination of data and AI algorithms create the possibility of new and more effective workarounds. For example, when diagnostic imaging is unavailable, an evermore accurately analyzed sample of blood or other body fluids might help with diagnosis. As a result, shifts in value creation are difficult to predict.
Building Competitive Advantage
Managers expect significant improvement in performance of current processes or products from AI. Many companies are focused on addressing those. However, mere improvement does not create a sustainable competitive advantage — when everyone finds the same efficiencies, only the baseline shifts. For AI to become a prominent feature in future strategies, companies must figure out how humans and computers can build off each other’s strengths to create competitive advantage. This is not easy: Companies need privileged access to data — which, as we’ve seen, many do not now have. They must learn how to make people and machines work effectively together — a capability relatively few Pioneers have at present. And they need to put in place flexible organizational structures, which means cultural changes for both company and employee.
Just about any company today needs a plan with respect to AI. Most do not have one, and those that have been slower to move have some catching up to do. Those that continue to fall behind may find the playing field tilted evermore steeply against them.
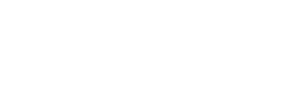
Appendix: Work in the Longer Term
Survey respondents and most interviewees both expect big changes from AI in the next five years. But the more dramatic effects of AI may occur within 10 to 20 years. What can we expect in that time frame?
Automation of Tasks ≠ Automation of Jobs. History shows that jobs evolve as tasks shift. BP’s Ahmed Hashmi says the company’s engineers used to spend a lot of time hunting for data to put together their reports, but “now that’s all automated. We’ve got a data lake, which gives engineers ready access to all the data. We employ the same number of engineers, but they’re improving the business rather than searching for data to get ready to improve the business.” In other words, extrapolation from the automation of repetitive tasks to the automation of jobs in a high tech industry is risky business.
AI as Job Creator. Increased organizational reliance on AI will create new needs as it meets current needs. The job of an insurance underwriter, for example, tops many “most endangered species” lists. However, AI simultaneously expands the universe of insurable events. And, as James Platt, chief operating officer of Aon Risk Solutions, has said, “Many things that people would like to insure themselves against, such as brand and reputational risks or wider cybersecurity coverage, are ‘uninsurable’ today. There is simply no one offering an insurance option.” As new methods of assessing risks become available, underwriters can start offering such new services. Missy Cummings, director of the Humans and Autonomy Laboratory at Duke University, puts it this way: “What we often don’t think of are the jobs that are created as other new businesses come up around a technology.”
If it’s hard to imagine AI as doing anything other than eliminating jobs, step back and consider the scope of the problem. The 2016 World Economic Forum report, “The Future of Jobs,” noted that “upcoming disruptions to the employment landscape are going to be a lot more complex and multifaceted than conveyed by a narrow focus only on automation”8 — saying, in a nutshell, that digital technologies and AI are not the only forces transforming the nature of work. It has been clear for some time that technological change — not just AI — obliges employees to become lifelong learners and embrace career flexibility, but as the WEF report observes, even more factors are at play: “technological, socioeconomic, geopolitical, and demographic developments and the interactions between them will generate new categories of jobs and occupations while partly or wholly displacing others. They will change the skill sets required in both old and new occupations in most industries and transform how and where people work.”9 Yet we have also seen digital technologies be used to address this problem. Accompanying the expansion of AI are many new learning options for humans: Augmented reality, new training tools, and digitally accessible forms of education (such as massive open online courses [MOOCs] and “nanodegrees”) are proliferating.
Against a canvas of even broader social, demographic, environmental, and global political developments, predictions of aggregate employment levels based on AI alone are difficult; there are too many countervailing forces to discuss any one of them in isolation. But it is not unreasonable to imagine an opportunity for AI to cushion some of its own impacts, and perhaps the impacts of other factors, by helping to anticipate the coming changes in the job market and identify (and meet) workforce training needs as they arise.
Even So, Inertia Is Not an Option. Big global uncertainties should not deter corporations from acting today, when action is required. Infosys, for example, has trained more than 120,000 employees in design thinking. This new capability will enable its employees both to shape a world of new AI-based service offerings and automate historic business processing services.
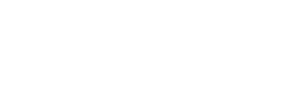
About the Research
To understand the challenges and opportunities associated with the use of artificial intelligence, MIT Sloan Management Review, in collaboration with The Boston Consulting Group, conducted its inaugural annual survey of more than 3,000 business executives, managers, and analysts from organizations around the world.
The survey, conducted in the spring of 2017, captured insights from individuals in 112 countries and 21 industries, from organizations of various sizes. More than two-thirds of the respondents were from outside of the United States. The sample was drawn from a number of sources, including MIT Sloan Management Review readers, and other interested parties.
In addition to our survey results, we interviewed business executives from a number of industries and academia to understand the practical issues facing organizations today. Their insights contributed to a richer understanding of the data.
For the purpose of our survey, we used the definition of artificial intelligence from the Oxford Dictionary: “AI is the theory and development of computer systems able to perform tasks normally requiring human intelligence, such as visual perception, speech recognition, decision-making, and translation between languages.” However, AI is evolving rapidly, as is the understanding and definition of the term.
Comments (4)
jing su
Jeniffer de Souza
Jonathan Obise
Josafat Valle