Data Decisions: You Can’t Be Held Responsible (Or Can You?)
In the wake of the 2009 earthquake in L’Áquila, Italy, seven Italian scientists were convicted of manslaughter charges for not adequately communicating the potential threat.
Topics
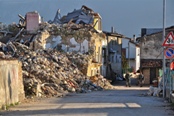
In the wake of the 2009 earthquake in L’Áquila, Italy, seven Italian scientists were convicted of manslaughter charges for not adequately communicating the potential threat.
Image courtesy of Flickr user Darkroom Daze.
Back in 1633, Italian mathematician, physicist and astronomer Galileo was sentenced, as part of the Roman Inquisition, to lifelong house arrest. His crime? Suggesting that the sun, and not the earth, was the center of the universe.
Fast forward nearly 400 years and scientific opinions are again embroiled in controversy.
In October, a group of seven Italian scientists were convicted of manslaughter charges for not adequately communicating the potential threat of something they couldn’t scientifically predict: the deadly 2009 L’Áquila, Italy, earthquake.
The fact that this group of highly regarded scientists has been sentenced to prison for providing what ABC news called “inaccurate, incomplete and contradictory information” — for giving people a false sense of security — raises the question: Could there be implications for organizations that utilize data science to guide decision making?
In other words, what happens when a strategy goes awry, a product flops or investors lose money? Can data analysts be held accountable?
Data mining newsletter KDNuggets asked its readers the question: Should data scientists/data miners be responsible for their predictions? Its results:
- 45% said no, data scientists/data miners should not be held responsible for their predictions;
- 37% said data scientists/data miners can be held financially responsible, if they also benefit from correct predictions;
- 5% said data scientists/data miners can be held criminally responsible for wrong predictions; and
- 13% weren’t sure which direction the wind was blowing
Experts that I spoke with agree on two points. First, that basing a decision on data is no guarantee that a strategy is going to work, though it will typically mean fewer failures. And second, that basing a decision on data, where data is available, beats intuition alone.
Andrew McAfee, principal research scientist at MIT’s Center for Digital Business, points out that analytics-based decision makers shouldn’t be called out for a bad decision any more than their counterparts who do not utilize analytics. At the same time, says McAfee, “as more data becomes available, decision making should become more rigorous.
Comments (2)
somkdsr
dbhalbur