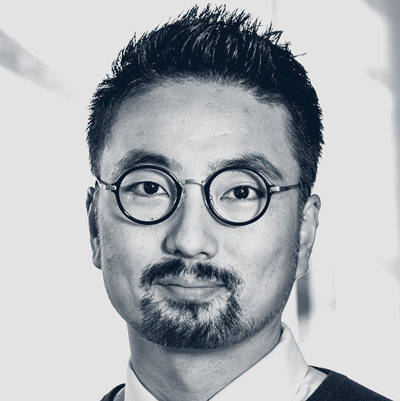
Tae Wan Kim is an associate professor of business ethics and the Xerox Junior Faculty Chair at Carnegie Mellon’s Tepper School of Business. He is also a faculty member of the Block Center for Technology and Society at Heinz College and Carnegie Mellons CyLab. Kim is on multiple editorial boards and has served as a committee member of the IEEE Global Initiative for Ethical Considerations in Artificial Intelligence and Autonomous Systems and the AAAI/ACM Conference on Artificial intelligence, Ethics, and Society. He has a doctorate in business ethics and legal studies from The Wharton School.
Voting History
Statement | Response |
---|---|
Mature RAI programs minimize AI system failures. Strongly agree | “AI systems can fail or succeed, and any successful AI systems must be ethically responsible. The idea that engineering and ethics can be separated in certain ways should be rejected. Saying, “This system is itself successful but only ethically fails,” is a contradiction in terms. That’s similar to saying, “He is a great person but just harms others.” If an AI system is really successful, it must be ethically mature. Hence, if it is not ethically mature, it is not a successful AI. A simple logic: modus tollens.” |
RAI constrains AI-related innovation. Strongly disagree | “Responsible AI constraints are currently major forces of AI-related innovation. XAI (explainable AI) is a great example. For instance, the Equal Credit Opportunity Act, a precursor of the “right to explanation,” demands that financial firms provide decision rationales to customers in the United States. However, while AI systems provide judgments, classifications, and guidance, they rarely provide a rationale or explanation (unless explicitly designed to do so). This lack of explanation by the algorithm can lead to a loss of trust by expert users (such as traders and management) as well as those who are impacted by the subsequent AI-driven actions (such as clients, regulators, and policy makers). The critical need for explanations and justifications by AI systems has led to calls for explainable AI. Now, search for “explainable AI” in Google Scholar. You will see a number of attempts to develop transparent algorithms that mimic the black box problem with high fidelity. Furthermore, the ethical demand for XAI even pushes the AI scholarship to develop high-performing algorithms that are inherently transparent from the beginning. It also motivates researchers to move beyond the current correlation-based AI models.” |
Organizations should tie their responsible AI efforts to their corporate social responsibility efforts. Disagree | “Much of CSR is strategy, but responsible AI is mandatory. It’s true that ethics can be used as a strategy for reputation management, and companies do so under the name of CSR. However, such a perspective is insufficient. Take Enron, for example, a company that was engaged in several philanthropic (beyond duty) activities while it was also recklessly infringing upon ethical duties around deception and manipulation. Of course, there are wide overlaps between what is right and what is profitable, which makes ethics a viable strategy. And yet some ethical values cannot be translated into the language of strategy as a specialty tool. If companies regard ethics purely as a strategy, they can fall into the trap, “If the only thing one has is a hammer, then everything starts to look like a nail.” Relatedly, CSR efforts must be visible to customers, but much of responsible AI efforts is technical and difficult to explain to customers. Note that CSR has not been as viable in B2B as much as B2C, because doing right and good in B2C is visible to customers, whereas the visibility does not exist in B2B.” |
Responsible AI should be a part of the top management agenda. Neither agree nor disagree | “How top management behaves makes a big impact on low-ranking employees. But we need more evidence about whether having responsible AI as part of the top management agenda makes a positive or negative impact. Strengthening independence of an internal ethics team is important, and there can be trade-offs. Think about a recent case at Google. Google, which probably has the largest ethical AI team, recently fired an African American woman, who was a machine learning researcher in the ethics team, because of her paper that studied ethical problems of large language models. This decision put Google into moral turmoil and provoked public outcry. The top management’s involvement with the ethics team turned out to be negative.” |