Tackling AI’s Climate Change Problem
The AI industry could soon be one of the largest contributors to carbon emissions, if current trends continue.
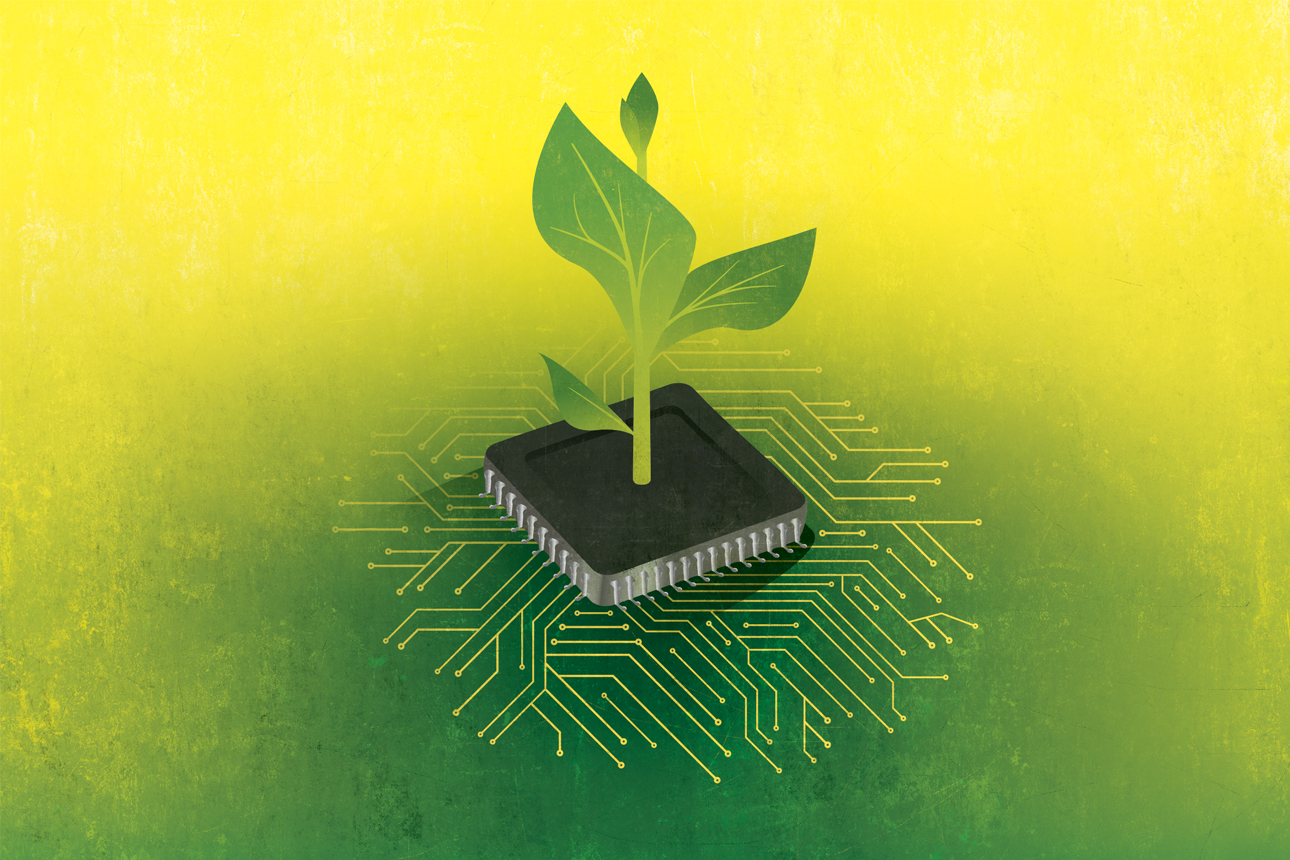
Traci Daberko
In an era defined by both the promise of technological innovation and the threat of climate change, artificial intelligence has emerged as both a valuable tool and a difficult challenge. As we use AI to tackle tough problems, we must also grapple with its hidden environmental costs and consider solutions that will allow us to harness its potential while mitigating its climate impact.
The success of OpenAI’s ChatGPT language model, which is backed by Microsoft, has sparked a technology arms race, with tech giants making enormous investments in building their own natural language processing systems. But the quest for more intelligent machines is quickly running into a web of sustainability challenges. AI has a fast-growing carbon footprint, stemming from its voracious appetite for energy and the carbon costs of manufacturing the hardware it uses. Since 2012, the most extensive AI training runs have been using exponentially more computing power, doubling every 3.4 months, on average.1
Get Updates on Leading With AI and Data
Get monthly insights on how artificial intelligence impacts your organization and what it means for your company and customers.
Please enter a valid email address
Thank you for signing up
AI’s Environmental Costs
The environmental impact of information technology is often overlooked, even though data centers and transmission networks account for 1% to 1.5% of global electricity use. They also account for 0.6% of global carbon emissions, which need to be cut in half to achieve a net-zero emissions scenario by 2050, according to the International Energy Agency.2 A single average data center consumes the equivalent of heating 50,000 homes yearly. Electronic waste is the fastest-growing waste stream in the world, amounting to a staggering 57 million tons generated each year, about the same weight as the Great Wall of China.3
Several factors contribute to the carbon footprint of AI systems throughout their life cycles:
Large and complex models: Large language models (LLMs) require tens of thousands of cutting-edge high-performance chips for training and for responding to queries, leading to high energy consumption and carbon emissions. The greater the model’s complexity, the more task times increase, resulting in more energy consumption.4 LLMs like ChatGPT are among the most complex and computationally expensive AI models. The capabilities of OpenAI’s GPT-3 LLM are made possible by its 175 billion-parameter model, one of the largest when it was launched. Its training alone is estimated to have used 1.3 gigawatt-hours of energy (equivalent to 120 average U.S.
References
1. “AI and Compute,” OpenAI, May 16, 2018, https://openai.com.
2. “Data Centers and Data Transmission Networks,” International Energy Agency, accessed Oct. 16, 2023, www.iea.org; and D. Patterson, J. Gonzalez, Q. Le, et al., “Carbon Emissions and Large Neural Network Training,” Arxiv, April 23, 2021, https://arxiv.org.
3. O. Rosane, “This Year’s E-Waste to Outweigh Great Wall of China,” World Economic Forum, Oct. 18, 2021, www.weforum.org.
4. R. Cho, “AI’s Growing Carbon Footprint,” State of the Planet, June 9, 2023, https://news.climate.columbia.edu.
5. Patterson, “Carbon Emissions and Large Neural Network Training.”
6. M. Schreiner, “GPT-4 Architecture, Datasets, Costs and More Leaked,” The Decoder, July 11, 2023, https://the-decoder.com.
7. P. Schmid, O. Sanseviero, P. Cuenca, et al., “Spread Your Wings: Falcon 180B Is Here,” Hugging Face, Sept. 6, 2023, https://huggingface.co.
8. This figure is based on the author’s calculations, given reasonable assumptions regarding GPU energy demands and energy mix.
9. M. O’Brien and H. Fingerhut, “Artificial Intelligence Technology Behind ChatGPT Was Built in Iowa — With a Lot of Water,” Associated Press, Sept. 9, 2023, https://apnews.com.
10. P. Dhar, “The Carbon Impact of Artificial Intelligence,” Nature Machine Intelligence 2, no. 8 (August 2020): 423-425.
11. “A New Circular Vision for Electronics: Time for a Global Reboot,” PDF file (Geneva: World Economic Forum, January 2019), www.weforum.org.
12. E. Mehlum, D. Hischier, and M. Caine, “This Is How AI Will Accelerate the Energy Transition,” World Economic Forum, Sept. 1, 2021, www.weforum.org.
13. M. Javaid, A. Haleem, I. Haleem Khan, et al., “Understanding the Potential Applications of Artificial Intelligence in Agriculture Sector,” Advanced Agrochem 2, no. 1 (March 2023): 15-30.
14. Cho, “AI’s Growing Carbon Footprint”; and N. Sundberg, “Sustainable IT Playbook for Technology Leaders” (Birmingham, U.K.: Packt Publishing, 2022).
15. D. Patterson, J. Gonzalez, U. Hölzle, et al., “The Carbon Footprint of Machine Learning Training Will Plateau, Then Shrink,” Computer 55, no. 7 (July 2022): 18-28.
16. Sundberg, “Sustainable IT Playbook for Technology Leaders.”
17. Patterson et al., “The Carbon Footprint.”
18. Ibid.
19. K. Foy, “AI Models Are Devouring Energy. Tools to Reduce Consumption Are Here, if Data Centers Will Adopt,” MIT Lincoln Laboratory, Sept. 22, 2023, www.ll.mit.edu.
20. Patterson et al., “The Carbon Footprint.”
21. T. Jackson and I.R. Hodgkinson, “What Is ‘Dark Data’ and How Is It Adding to All of Our Carbon Footprints?” World Economic Forum, Oct. 5, 2022, www.weforum.org.
22. M. van Rijmenam, “Building a Greener Future: The Importance of Sustainable AI,” The Digital Speaker, Feb. 23, 2023, www.thedigitalspeaker.com.