What Managers Should Ask About AI Models and Data Sets
Business leaders must make the right calls on whether and how broadly to deploy AI models. Ask these tough questions.
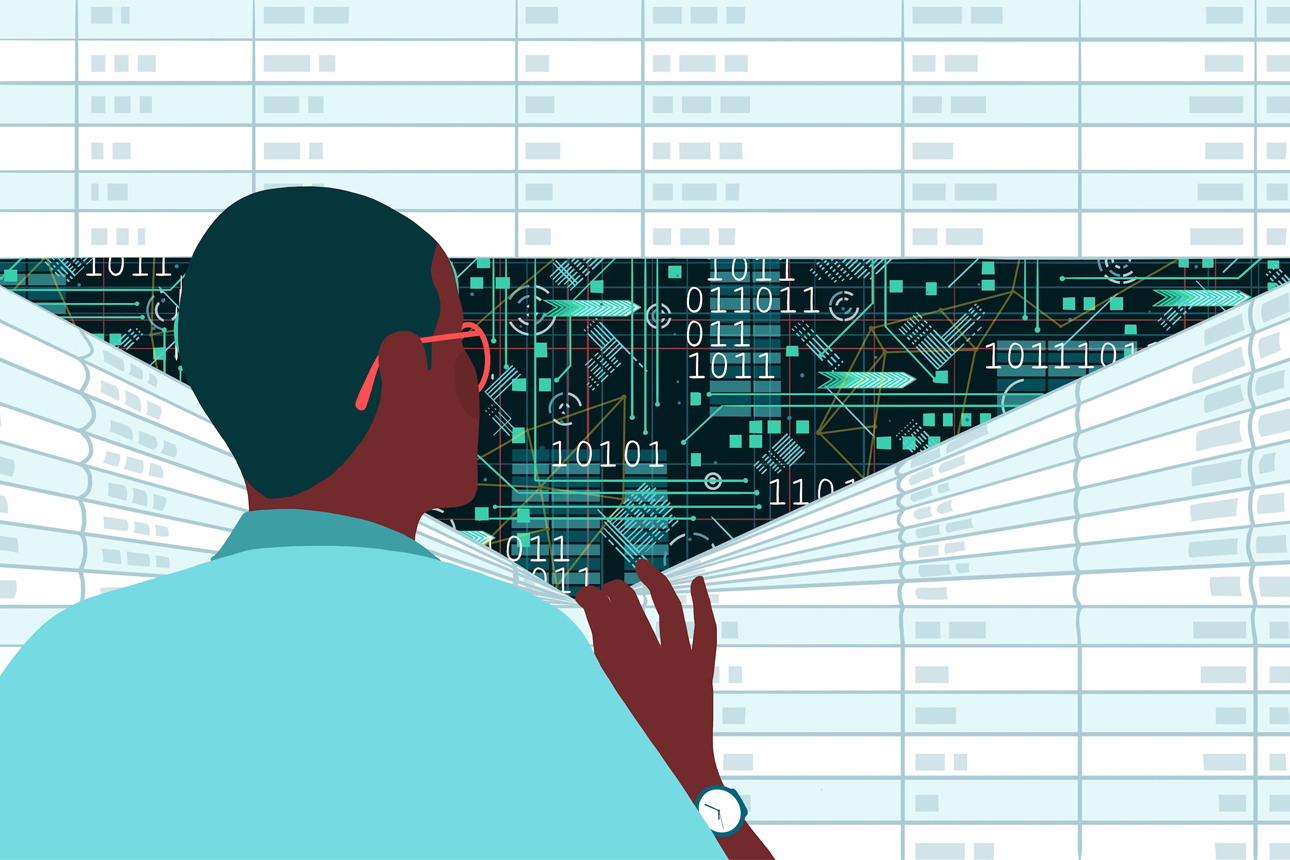
Alice Mollon/Ikon Images
The power of AI and the machine learning models on which it is based continue to reshape the rules of business. However, too many AI projects are failing — often after deployment, which is especially costly and embarrassing. Just ask Amazon about its facial recognition fiascos, or Microsoft about its blunders with its Tay chatbot. Too often, data scientists write off such failures as individual anomalies without looking for patterns that could help prevent future failures. Today’s senior business managers have the power — and the responsibility — to prevent post-deployment failures. But to do so, they must understand more about the data sets and data models in order to both ask the right questions of AI model developers and evaluate the answers.
Maybe you’re thinking, “But aren’t data scientists highly trained?” The vast majority of training for today’s data scientists focuses on the mechanics of machine learning, not its limitations. This leaves data scientists ill-equipped to prevent or properly diagnose AI model failures. AI developers must gauge a model’s ability to work into the future and beyond the limits of its training data sets — a concept they call generalizability. Today this concept is poorly defined and lacks rigor.
Get Updates on Leading With AI and Data
Get monthly insights on how artificial intelligence impacts your organization and what it means for your company and customers.
Please enter a valid email address
Thank you for signing up
A saying in analytics asserts that model developers and artists share the same bad habit of falling in love with their models. Data, on the other hand, doesn’t get the attention it requires. For example, it’s all too easy for AI model developers to settle for readily available data sets rather than seeking ones more fit for the problem at hand.
Senior business managers, lacking advanced degrees in technical disciplines, are even less equipped to spot trouble related to AI models and data sets. Yet it’s these business leaders who ultimately decide whether and how broadly to deploy AI models. Our goal for this article is to help managers do that better, using:
- A framework that delivers needed context. In particular, we’ll introduce the concept of “the right data.” Mismatches between the right data and the data actually employed in an AI project can be risky.
- A set of six questions to ask their organization’s AI model developers before and during modeling work and deployment.
- Guidance on how to assess AI model developers’ answers to those six questions.