Using Analytics to Improve Customer Engagement
Organizations that turn data into insights are gaining competitive advantage through improved connections with consumers.
Bigger Harvests, Better Relationships
For many U.S. farmers, improving agricultural productivity while meeting consumer demand to reduce the use of pesticides and chemicals on crops became a goal during the 2000s. To help farmers manage pests, plant diseases, weather conditions, and yields, dozens of startups emerged to offer apps and data services — part of a precision agriculture boom. Many of these companies failed or struggled as data alone proved insufficient; farmers also needed help interpreting the data. By 2016, a new variety of data-oriented service providers was helping farmers apply their harvested data.
For example, WinField United, the seed and crop-protection division of Land O’Lakes Inc., helps farm operators analyze millions of data points from multiple sources, to boost per-acre production. WinField United taps sources such as controlled experiments at test farms, performance data gathered from sensors provided by equipment manufacturers, and extensive weather data. Analytical and decision-support tools use these myriad data sources to present individualized recommendations to the company’s customers. The analytics helps grow more than crops; it fosters loyal relationships with core customers in the process.
According to Teddy Bekele, WinField United’s vice president of agricultural technology, there is a great disparity in the use of technology and analytics among farmers. But for active adopters, the return on investment can be dramatic. “We are working with farmers who were averaging about 150 bushels per acre but, after following our recommendations, are now producing 195 bushels — paying for their incremental investment five times over,” says Bekele.
By helping its customers achieve measurable gains, WinField United strengthens the bonds it has with them, deepening customer engagement. “Farming is very relationship-based, so, on top of the data, our goal is to get them to say, ‘WinField United is giving me the right advice and helping me make the right choices,’” says Bekele. Analytics is helping the company move from a strictly transactional relationship with its customers to one in which it is perceived to be a trusted adviser.
Winfield United’s program exemplifies heightened demand, across industries, for better customer engagement that depends on data and analytics. The 2018 MIT Sloan Management Review Global Executive Study and Research Report provides a detailed examination of data-driven customer engagement. The study is based on our eighth annual global data and analytics survey, conducted during 2017, which included 1,919 managers from 101 countries and interviews with 17 leading practitioners and thought leaders.
Our findings demonstrate a significant increase in the number of organizations that are using analytics to gain a competitive advantage and innovate — a key component of this shift is more effective use of analytics to improve customer engagement. Data and analytics allow organizations to use intelligence from feedback to tailor offerings that improve customer satisfaction. Several factors appear to be at work, including the use of a wide range of data sources, well-developed core analytics capabilities, and integration of artificial intelligence (AI) and the internet of things (IoT) into processes. Companies that have businesses as their main customers (business-to-business, or B2B) are gaining the most benefits from this shift, in part because they are able to share data with customers in a way that directly strengthens their relationship.
Key findings from this year’s research point to the following trends:
- Competitive advantage from analytics continues to grow. More than half (59%) of managers say their company is using analytics to gain a competitive advantage. This is a higher percentage of respondents than in the previous two years.
- Analytics is driving customer engagement. Organizations that demonstrate higher levels of analytical maturity saw a marked advantage in their customer relationships. The most analytically mature organizations are twice as likely to report strong customer engagement as the least analytically mature organizations.
- Analytically mature organizations use more data sources to engage customers. Many organizations are already making use of data from customers, vendors, regulators, and competitors, but Analytical Innovators are more than four times more likely to glean data from all four sources. They also are much more likely to use a variety of data types — such as mobile, social, and public data — to engage customers compared with less analytically mature organizations.
- Sharing data can improve influence with customers and other groups. Sharing data doesn’t mean giving away the farm. Organizations that share their data with others (customers, vendors, government agencies, and even competitors) reported increased influence with members of their ecosystem. Sharing data can enhance a company’s influence with not only customers but also a broad array of other stakeholders.
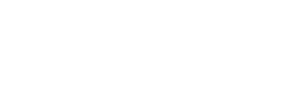
Key Trends
Competitive Advantage From Analytics Increases — Even Though Terrain Is Shifting
The percentage of respondents who attribute significant competitive advantage to their analytics proficiency has risen for the second year, to 59%, up from 51% in 2015. This upward swing is the result of many factors. Our interviews with industry executives and academics highlight three.
One factor is the use of data and analytics to ward off new competitors that are themselves using analytics to enter fresh markets. Because of the more pervasive use of analytics,1 organizations must continue to improve their use of data and analytics to stay ahead. (See Figure 1.)
Consider health insurance. Within the constraints of a heavily regulated environment, the industry has long made extensive use of data and analytics to manage risk — the core competency of its business. Today, however, new competitors — organizations with analytics expertise that has been honed in a largely unregulated environment — are looming. For example, in October 2017, Amazon was granted pharmacy-wholesaler licenses2 in a dozen states, and the market was quick to react, with sell-offs of companies like McKesson Corp. and AmerisourceBergen Corp. The news also affected merger talks between CVS Health and Aetna Inc.3 Industry convergence is forcing some insurers to reconsider their roots as they acknowledge a need to be increasingly data-centric. “You’ve got Amazon considering becoming a pharmacy benefits manager and Google looking at moving into the clinical space, and we’re really going to need to move more in that direction,” notes a business manager we interviewed from a payer organization. “The insurance industry is all about trying to reduce risk, and that’s culturally one of the barriers to admitting we may sometimes need to introduce more of that risk in order for us to disrupt ourselves before we get disrupted by other industries,” he says. Staying the same may be riskier than change.
Another factor is the use of data to enhance customer engagement. Like many brick-and-mortar retailers, Mall of America continually looks for ways to build enduring relationships with its 42 million annual visitors, including a young generation disposed to shop online. While providing the free Wi-Fi access shoppers now expect, the mall uses these hot spots to collect aggregate data about foot traffic patterns, dwell times in particular locations, and the effect of mall events on visitor counts. Robust analytics — and the strategic daily decisions they enable for mall management and retail tenants — has become an indispensable tool that needs continuous refining.
In 2016, with the help of the Carlson Analytics Lab at the University of Minnesota’s Carlson School of Management, Mall of America mapped Wi-Fi data to its floor plans and identified clusters of anonymous shoppers based on their movement through the facility. Similar to online clickstream analytics, the data provided a behavior-based way to track customer segments. The mall also added full-time analysts to its staff. “We are creating a whole analytics mentality,” says Janette Smrcka, Mall of America’s director of information technology. “We now have strong business users who, once they get those dashboards, are really diving in. The more we expose our company to this, the more it spurs ideas.” For instance, Mall of America has begun to use weather data to more accurately predict mall traffic. “In the past, if schools closed due to very cold weather, we could be inundated with traffic unexpectedly, as parents send their kids to the mall,” explains Smrcka. By counting cars in the parking lots and relating the volume to weather patterns, Smrcka is able to provide forecasts to stores inside the mall so that they can adjust staffing levels.

An infographic based on the 2018 Data & Analytics Report illustrates how companies can better engage with customers using analytics.
Another factor driving increased competitive advantage from analytics is the use of data to better predict human behavior, a capability that can improve models at many levels. For example, insurers can better model how social, legal, and economic factors affect insurance loss by better understanding the underlying behaviors from which they stem. At global reinsurance company Swiss Re AG, Riccardo Baron, vice president and senior quant analyst and casualty modeler, is developing forward-looking models that join the quantification of human behavior with expertise in catastrophic disasters through advanced analytics. “We are combining more traditional actuarial modeling techniques with modeling and data science techniques, including machine learning approaches, not only to understand essentially historical data but for predicting the future,” he says. Baron says it will be the company’s focus for the next year. “You can imagine extraordinarily complicated, unforeseen scenarios, for example, in the area of autonomous cars or any IoT device,” he says. “What’s the liability that arises if someone hacks into a device and modifies it? How much of the liability would fall on the manufacturer? How much on the user? Technology raises a lot of questions, and to model these emerging risks, you cannot limit your view to the past. We have to imagine possible scenarios that are radically novel.”
The Continuing Challenge of Gleaning Insight From More and More Data
This year’s survey found that access to useful data is at record levels: Once again, three-quarters of respondents reported more access to useful data compared with the previous year. This is the sixth year in a row that at least 70% of respondents have agreed that their access to useful data has increased from the prior year. (See Figure 2.)
But many companies are still struggling to extract actionable insights from this mushrooming storehouse. Notably, the gap between more access to useful data and the ability to develop practicable insights has doubled, from 14% in 2012 to 28% in 2017. Only 49% of respondents in 2017 reported being able to use data to guide future strategy, compared with 55% in 2016.
How can 59% of the organizations report that they obtain competitive advantage from analytics but only 49% are able to use data to guide future strategy? Christopher Mazzei, global chief analytics officer and global innovation technologies leader at EY, believes at least part of the answer relates to the continued growth in access to data: “Many people cast too wide a view and are not focusing enough on a particular problem. There’s still that notion of, ‘Well, we have a lot of data. What might we learn from it?’ That’s a very different type of challenge than, for example, trying to improve customer retention in a specific part of the business.”
While analytics is providing advantage in many areas, more and more data is available in other areas that companies have not been able to use well. Analytics talent remains a constraint,4 particularly as data is newly available in areas that have lacked data in the past. “A lot of people think they know how to answer business problems using data and analytics, but it’s a difficult skill that people are trained, both academically as well in their experience, to do,” Mazzei says. Worse, “the field is moving fast and the range of business problems that organizations are trying to address through analytics is getting wider.” Having better tools and more data is not enough; removing these prior constraints makes other constraints more salient.
This disparity points to the critical distinction between data collection and analytics. Robust amounts of data are essential for providing sufficient input, but more data in and of itself cannot provide value to the organization — and may, in fact, add confusion. “I don’t think the relationship between more data and action is a linear one,” explains Lori Bieda, head of the Analytics Centre of Excellence, Personal and Business Bank, Canada, at the Bank of Montreal. “Our ‘customer journey’ data set, which shows how customers engage with our brands across all touchpoints, grew from 65 terabytes to 98 terabytes in just eight months,” says Bieda. “Although it was rich and interesting, we had to contrast that data with other sources such as research and ultimately contextualize it. This process can yield conflicting information, and that can become confusing.”
New dependencies can slow down processes or create new bottlenecks as people wait for their analyses before making decisions in the course of their daily activities. “When we first started doing analytics, we had a lot of data, but it wasn’t a massive amount and we could just append data to existing tables,” explains Bruce Bedford, vice president of marketing analytics and consumer insights at Oberweis Dairy Inc., a multistate home-delivery business and fast-food chain in North Aurora, Illinois. “But at some point, these tables and the database grow to be so large that it becomes very time-consuming to use analytics to calculate variables and run the models. I grew one of the tables to well over 300 million records and found that it was taking hours just to run simple analytics that would have taken a few minutes when we first got started.” This slow growth was not noticeable each day, but over time, it added up. As a result, time left for decision-making withered and managers lost time for developing insight.
In response, Oberweis adopted strategies to optimize its analytics. “We add data incrementally every day, and it’s batch-processed,” says Bedford. “You have to pay attention to runtimes and what the dependencies are and what the schedules of those batch jobs look like. As you add data, things change. It is a living environment, and you have to keep up on it.”
More Analytically Mature Organizations Than Ever Before
A minority of companies excel at meeting the challenge of deriving valuable insights from more access to useful data. The most analytically mature organizations — determined by answers to a set of survey questions 5 — increased to 20% in 2017, up from 17% in 2016. (See Figure 3.) In six years, the size of this group almost doubled, primarily at the expense of the maturity group we label Analytical Practitioners, which dropped to 46% from 60%.
One of the clear differences between Analytical Innovators and the other maturity groups is their ability to successfully use data and analytics to deepen customer engagement along several key dimensions. The most analytically mature organizations are twice as likely to report strong customer engagement compared with the least analytically mature organizations. (See Figure 4.) In what follows, we offer a detailed analysis of the factors behind successful corporate efforts to use data to enhance customer engagement.
Notably, maturity groups claim similar levels of risk regarding the loss of customers. One might expect Analytical Innovators to have a lower risk of losing customers relative to other maturity groups because they have stronger engagement with customers. Analytical Innovators may be able to discern the many ways their customers might be lost to competition, however, just because of their strong analytics capabilities. According to this interpretation, Analytical Innovators’ heightened awareness of customer and competitor behavior leads to a greater appreciation of the risks of customer loss as a result of their data-driven customer intelligence and engagement. Alternatively, one might expect that Analytical Innovators would be at a higher risk of losing customers relative to other maturity groups because customers might perceive their heightened ability to collect data and tailor offerings to be invasive. But our results show that Analytical Innovators obtain business benefits with no worse risk of losing customers compared to other maturity groups.
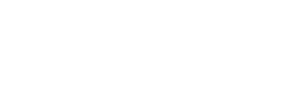
Turning Data Into Customer Engagement
Building the capability to deliver data-driven insights sustainably requires developing competencies around ingesting data, analyzing it, and applying insights from analyses. To determine the relative analytics proficiency of an organization, MIT Sloan Management Review developed the Analytics Core Index, based on the organization’s core analytics capabilities in (1) ingesting data (capturing, aggregating, and integrating data); (2) analyzing (descriptive analytics, predictive analytics, and prescriptive analytics); and (3) applying insights (disseminating data insights and incorporating insights into automated processes). (See “Calculating the Analytics Core Index.”)
Not surprisingly, organizations that use analytics to achieve the highest levels of customer engagement tend to have the highest scores on the Analytics Core Index. (See Figure 5.) But there’s more to the story. Our analysis offers clear evidence that organizations that make effective use of a wide range of data sources — from different types of technologies and different types of entities, such as customers, vendors, competitors, and publicly available sources — are more likely to use analytics to generate higher levels of customer engagement and gain a competitive advantage than organizations that use fewer sources of data. Higher levels of analytical maturity are associated with higher levels of customer engagement, which in turn is associated with higher scores on the Analytics Core Index, which in turn is associated with greater use of diverse data sources.
More Sources, More Value
Analytical Innovators that define today’s best practices take advantage of multiple data sources to glean new customer insights and deepen their relationships. Among Analytically Challenged organizations, 75% tap at least one external data source to inform their models. But companies at the Analytical Practitioner and Analytical Innovator levels regularly integrate data from multiple sources, and Analytical Innovators are almost five times as likely to use data from all four of these external sources (customers, vendors, competitors, and public sources). (See Figure 6.)
Wescom Credit Union, which operates out of Anaheim, California, for more than 200,000 members, turns to a large number of internal and external data sources to develop highly detailed strategies for customer engagement. “We have a plan to deliver what Amazon and Netflix are similarly doing using billions of data points,” says David Gumpert-Hersh, vice president of Wescom’s business analytics group. Wescom established a daily data flow from myriad sources, including third-party systems, and uses the data to build members’ status and behavior profiles. “We use that to come up with highly targeted recommendations for them,” says Gumpert-Hersh. For instance, if Wescom determines that a member recently made large purchases at a do-it-yourself building center or has significantly increased the balance in a savings account, the action would trip a “hurdle level” indicating the member is most likely in need of a mortgage. The credit union would then create a mortgage marketing campaign specifically for that member. “If you’ve opted in, you’ll receive an email within 24 hours while you’re still motivated from that purchase or still in the process of pursuing financing,” says Gumpert-Hersh.
Wescom sees this level of personalization — with both relevant messaging and optimal timing — as key to drawing in marketing-wary consumers. “I think we’ve swung one way where people are opting out of everything,” says Gumpert-Hersh. “Now, I think it’s going to start swinging back, and we will find more and more ways to use the same information but more efficiently. You’ve got a mosaic, millions of little tiles, that you’re putting together to create a bigger picture. We’re looking at data that we’ve never explored before, and we’re creating new data points from derivation.”
Multiple sources of data can also be used to provide value to customers indirectly; as organizations improve processes, customers benefit too. Devon Energy Corp., an independent energy company in Oklahoma City, Oklahoma, engaged in finding and producing oil and natural gas, uses data analytics in diverse areas of its energy-exploration efforts. To track the life cycle of underground gas reserves, Devon recently developed a “completions mapping” tool that provides hydraulic fracturing and production details from well completions. The data includes completions performed not only by Devon but also its competitors. Adding data from public sources allows the company to confirm and augment its own predictive modeling, better forecast production curve declines, and schedule operations with greater accuracy and cost savings.
Devon also uses a variety of data collection tools, from IoT sensors on equipment to real-time reports from field personnel via text from mobile devices. Thanks to advances in the company’s analytics tools, large volumes of natural-language (non-numeric) data are collected, digested, and used to support decision-making — a big change from past practices. It used to be that Devon’s employees would include written comments in their field reports, but most managers could not “physically read that many,” says Kathy Ball, Devon’s data management manager for exploration and production analytics. “Through the use of analytics, we can now review all of the comments and start to see how they tie into the numerical predictions for a piece of equipment,” she says. Ball calls this tapping the “internet of people.”
“How do we connect people and devices together? The sensors are just getting you raw numbers from the field,” she says. “They can provide you a pattern and tell you what’s taking place on your equipment, but analyzing the comments will also then inform you: How is that person interacting with that machine? How are you affecting that predictive failure? How can we look at human decisions and make the process even better?” Even when not directly visible to customers, data and analytics can still improve customer engagement.
Data Sources and Innovation
Across all industries, organizations that have a higher ability to innovate with data are more likely to use data from mobile devices (47%) than those with a lower ability to innovate (24%). (See Figure 7.) Anindya Ghose, professor of business at New York University’s Stern School of Business and author of Tap: Unlocking the Mobile Economy, points to the vast potential of mobile as a source of customer data. “One of the most fascinating sources of new data is the entire mobile ecosystem, which is basically a combination of telecom providers, brands, publishers, advertisers, and ad exchanges on various networks coming together,” says Ghose, who points out that mobile accounts for nearly 40% of all transactions. “Smartphones rule our lives. We text, swipe, and shop from our devices. And every time businesses tap into these phones, they are generating valuable streams of data that, using combinations of machine learning, data science, and artificial intelligence, they can put together and figure out a better way to curate their messages and their offers.”
Some companies with large bases of installed products are exchanging sensor- and IoT-based usage data with their customers to innovate their offerings, such as inventory management. When global technology company Lenovo Group Ltd. began installing telemetry software on customer machines as part of a quality improvement program, customers were able — and willing — to provide much more granular information about usage, and in effect they produced a rich store of data that proved useful well beyond Lenovo’s customer-service organization. “As people agreed to work with us as a part of our quality improvement program, it has been helping us solve another long-standing business issue,” says Arthur Hu, Lenovo’s chief information officer. “With channel partners, there’s a key question of how much inventory do you have in stock and how much have you sold, especially for some of the markets that have multitiered distribution channels and different layers. Enhanced data can help answer the question of, ‘Did someone buy the PC or not?’ in a more timely and accurate way.”
Using customer data to innovate may be more of an opportunity than some believe. According to Ghose, organizations may wrongly believe that because of privacy concerns, consumers are apprehensive about the use of their data. “I find that millennials, Gen Z, and, to some extent, Gen Y consumers are more than happy to exchange in this economy,” says Ghose, who believes many consumers are willing, for example, to share their location data if they know they will be targeted with relevant (but infrequent) offers. “Consumers want a brand to act as a butler and a concierge, and they are willing to give up their information if an organization acts responsibly,” he says.
The utility of adding more data sources has many limits. The incremental value from additional data may not be worth the effort — or other, less costly data sources may prove equally effective, as the team from Oberweis Dairy discovered. Previously, sophisticated models to predict individual value from potential customers required external data that was relatively expensive. Worse, the model results separated individuals in ways that were difficult to target. Now, Oberweis has more actionable analysis based on less expensive data. The company purchased inexpensive carrier-route data and combined it with other data sets to generate an overlay of carrier routes that matches its home-delivery footprint. Oberweis can use this information to model retention at the carrier-route level. “We figured out that we can get granularity sufficient to take advantage of postal discounts and still get great targeting for customers on the single behavior that matters most to our business from a financial perspective, which is retention rate,” says Bedford.
Becoming a Source of Data Creates Value
The benefits derived from a strong analytics core are not limited to information about customers. Companies that integrate data from external sources may themselves become sources of data for others, and thereby develop new ways to generate business value from their data.
Organizations that share their data externally, for instance, report an increase in their influence with groups they share with, including customers (86%), vendors (72%), and even competitors (75%). (See Figure 8.) This result may seem counterintuitive to managers who believe that sharing their data with others will cause their company to lose control of the information or lose the ability to extract value from it in ways that can make their organization uniquely valuable in a business ecosystem. Companies don’t need to monetize their data directly to cull value from it.
Mall of America finds that the shopper data it captures — such as traffic patterns, seasonality, and daily forecasts — is valuable to its retailers. These insights can help secure and retain high-value tenants. As evidence-based patterns of shopper behavior are shared, Mall of America establishes its value as a partner and source of essential data.
For Lenovo’s Hu, framing requests for access to data in this customer-oriented way helped dispel suspicion around data sharing, even within the organization. “You need to work through the classic ‘If I surrender or share that data, am I giving up power?’ concern,” he says. Lenovo found that it was helpful to not present the ask around an internal need — say, needing the data for internal reviews or management scorecards — but instead frame it as an immediate benefit to the customer. “It wasn’t a hard leap to say, ‘People are complaining because they don’t want to be asked their serial number five times.’ Adopting an external orientation helps a lot,” Hu says.
Reaching Outside the Enterprise: More Sources Correlate to Better Engagement
Across several customer engagement measures — such as the ability to tailor individualized offerings — companies that used more data sources scored higher on the Analytics Core Index. (See Figure 6.) These results were uniform across virtually all metrics, and the survey findings were echoed in the interviews conducted for this report. A natural interpretation of these results, supported by our interviews, is that a higher Analytics Core Index score is necessary to integrate and interpret a wider range of data sources.
“We know that people want personalized communications, because a one-size-fits-all approach no longer works,” says Kristen D’Arcy, head of performance marketing and media at American Eagle Outfitters Inc. She says:
What retailers are working towards is a much better view of the full marketing funnel, and deeper understanding of where consumers are in their journey to purchase. So, for example, I get an email from brand X, then I see brand X’s ad on Facebook, and based on these touches, I go into the store where I make my purchase. And then I see brand X’s Google ad a week later, and then I purchase again, but this time, online. That combination of touches drives brand awareness and, ultimately, purchases online and in-store.
D’Arcy adds, “But the holy grail is trying to understand which and how many it takes to convert. And that looks different based on certain demographics. Understanding what ingredients and when is key to making the recipe work most efficiently and is something that is on everybody’s radar right now.”
It can be challenging to analyze consumer-purchase data when it is derived from multiple sources, but Ghose points to encouraging advances in measuring attribution: “Some of the newer technologies that Google is deploying lets it track to a fairly reliable degree to what extent people who saw a search ad on a device actually ended up coming offline to that particular store. We’ve actually come a long way in addressing these gaps in measurement.”
Important for B2C But Even More Important for B2B
B2C (business-to-consumer) organizations use customer data to boost customer engagement, but the benefits can be even more significant in B2B relationships. For organizations that can share data about their clients’ customers, the perceived value can be even more salient. Notably, companies with a B2B focus have a higher level of customer engagement with analytics than companies with either a B2C focus or a split focus. This finding holds true across maturity groups. (See Figure 9.)
South African bank Nedbank Group Ltd. provides a useful illustration. In 2015, the bank began packaging its data analytics insights for commercial clients. It called the new service Market Edge, which essentially made its big-company analytics available to small and midsize businesses that make up a large part of Nedbank’s core clients. “When I told a store manager who believed that most of his business was derived from local residents that, in fact, half of his business was coming from residents who lived in a town 10 kilometers away, his eyes went wide. He said, ‘How do you know that?’” recalls Nedbank client Judy Gounden, a group marketing executive at Iliad Africa Ltd. Gounden was able to access — and more important, analyze — her own customers’ data using Market Edge. “The tool helps us think about how we market in each region,” says Gounden.6
For Baron, of Swiss Re, the value of the company’s analytics capabilities is compelling for its clients who might not have access to such extensive data or analytics talent. “We see liability models as an additional service we can provide to our clients,” he says. Baron explains:
We develop innovative models and visualization solutions for addressing problems that are relevant for our clients — especially those who wouldn’t have the capabilities to use the technologies that we have adopted. In return, we ask them to reinsure their risks with us instead of with our competitors. Because we take up their own risks, they know it is in our own interest that the models we offer are robust and trustworthy. We push forward-looking modeling as one of the primary ingredients of this new suite of solutions.
For a multinational electrical equipment manufacturer, getting closer to clients also means finding ways to engage customers by providing them with real-time insights. “Because we’re in the B2B market, a very large portion of our business is through partners, intermediaries, and distributors who sell to the end customer either directly or in partnership with us,” explains a vice president from the company. “We know that customers come to our website to interact with product information; that’s why our pages are so specialized — with CAD drawings, user manuals, and technical characteristics. We want an engineer or a panel builder to really get what they need in terms of all the details. Engaging them with chat, with a bot, or taking them to a purchase page so they can immediately access the information they need — that’s part of our engagement model.”
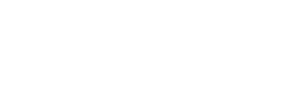
Moving More Quickly From Data to Customer Insights
Automation will be increasingly important to companies as they consume ever-larger quantities and varieties of data — not only to handle the volume but to deliver insights quickly so that customers can be engaged while they are tuned in to their relationship with the company and are poised to make key decisions. Having timely data is correlated with stronger scores on each of the customer engagement metrics we measured. (See Figure 10.) Respondents who use AI are 50% more likely to report that their data is timely than respondents who don’t.
Bank of Montreal is accelerating the pace at which data is delivered to decision-makers. For instance, its customer service agents receive an alert when a customer has trouble resetting a password — it triggers a process for resolving the issue. This is a high priority for the bank, since password resets have a clear impact on how likely a customer will be to take up other products.
American Eagle Outfitters is improving the speed at which it collects data and passes on insights. The company is piloting automated real-time “conversations” with customers who inform the retailer about their product preferences. The company and sub-brands such as lingerie retailer Aerie frequently assess the performance of these chatbot programs and take action based on insights from the automated customer interactions.
American Eagle’s D’Arcy is looking forward to the metrics from the pilot program. “We’ll see how the performance results look, where there’s drop-off in the experience, and where we’re seeing the most engagement as well as points of conversion — all of which can be measured in near real time,” she says. D'Arcy continues:
We’ll then perfect that experience before we port it over to other places, such as the website, among others. Once customers find a product that they like from our recommendation engine in the bot, they can shop for it via Aerie’s website. In addition to shopping and consuming content, users can share their thoughts about the chat experience with American Eagle Outfitters directly. All of that data can be used to make our broader marketing communications smarter across the board.
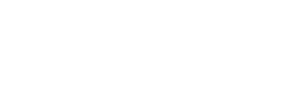
Enduring Challenges
While the organizations we most recently surveyed reported successes with analytics in creating competitive advantage and engaging with customers, none of them suggested these benefits are easy to achieve. Incorporating many data sources, moving quickly from data to decision, and aligning incentives remain fundamentally difficult tasks.
Our research continues to highlight a few persistent challenges as follows.
Striking the Balance in Data-Human Partnerships
Continued advances in machine learning, IoT sensor analytics, and AI represent one frontier of analytics. Swiss Re’s Baron describes how his organization uses algorithms to quantify expected future losses and price risk in areas for which only limited historical data is available: “Traditional actuarial work has to do with looking at the past, at historical losses in terms of frequency and severity.” Swiss Re’s forward-looking approach instead uses a scenario-based model. “We are now running actuarial models on scenarios that occurred in the past as well as on those that are hypothetical,” he explains. “This process doesn’t rely on the assumption — which is extremely restrictive — that the future will be shaped by the same risk drivers as the past did.”
Given current technology, humans still have an important role to play in delivering the fruits of automation and analytics to both customers and employees. For instance, when the tolerance for error narrows — as it does for Siemens AG customers using the company’s light railway systems — pairing automated processes with human experience is prudent. “Our processes using data-human partnerships are already quite good. It requires incredible accuracy to warrant our investing in a new approach, and it would not be worth it if there were too many false alarms,” says Gerhard Kress, vice president of data services at Siemens. He notes that companies often believe they can predict failures with a high rate of accuracy. “You think, if Amazon is right with 80% of the books they recommend to you, you’re totally happy. Unfortunately, 80% accuracy in our world is useless. Because these assets fail so seldom, I need prediction accuracies that are in the upper 90th percentiles,” says Kress. “But if you want to come to the 95% to 99% range, you need to incorporate information that is not part of the data usually, and this means you need insight provided by experienced people.”
As new developments in IT, AI, augmented reality, and even neuroscience are introduced, one frontier lies in the marrying of data-centric and consumer-centric lenses, or as Carl Marci, chief neuroscientist for Nielsen Co.’s Nielsen Consumer Neuroscience division, puts it, “the Facebooks and Googles” versus “the NBCs and CBSs.”
“From the beginning, the Facebooks and Googles have had this back-end data and have been mining it for analytics, and they’ve become enamored with it because it’s very powerful,” Marci says. “But televisions, for many, many years, had nothing on the back end and had to actually focus on the viewer — talk to them, count them, measure them. Both approaches have value, and I think that Silicon Valley’s eventually going to make the leap that you have to measure and talk to people on the other side.”
Leadership and Culture
Whether it’s Siemens executives cautiously approaching their conservative customers about applications beyond predictive maintenance or a health insurer’s analytics team helping company leadership see the value of data sharing with outside collaborators, promoting robust analytics activities can challenge long-standing norms and practices. One health insurance executive summarizes the dynamic: “In health care in general, there’s not a huge culture where data sharing is widely accepted. I’m seeing regulation slowly change that; it’s creating new relationships where competitors are now seen as partners. But the culture and the human aspect behind it is a lot more difficult to change. It is that cultural piece that’s going to be the biggest obstacle, not the technology piece.”
Kress agrees. “We are usually searching for a few people who understand what we are doing, and who can be forward-thinking,” he says of Siemens’ railway customers. “Very often, we spend the first couple of weeks educating the customer on what the data analytics can do for them. They have three, maybe four people in the organization who understand what we’re trying to do and who can think ahead in a way to help identify transformative use cases.”
But for organizations where the culture is analytics-driven, conversations in the C-suite are taking on a new tenor, as one executive we spoke with describes: “You can go to a meeting and have a conversation, but if people don’t come with the data, it’s almost not a legitimate conversation. Coming with facts about digital customer experience makes it easier to rise above anecdotal evidence of a service issue when managers can analyze data to surface the most significant, recurrent, and impactful problems. Very simply, you can prioritize one over another. That, to me, makes analytics a really transformative tool.”
Pervasive access to analytics — for not only decision-making but also planning and rallying teams around broader organizational initiatives — is therefore important. The same executive explains:
It helps align the organization. If I tell my team of technologists our next year’s focus is going to be, once more, customer satisfaction with our website, they may at first say it has nothing to do with them. But if you can help people draw the parallel between the digital engagement platforms that they build and enhance and the final outcome with customer satisfaction results, then they rally around it, and they’re celebrating part of the success every time we move five points forward towards our objective. Everybody’s very happy and feels they’re contributing to it. It’s a cultural motivator.
Organizing for Analytics
Cultural entrenchment may not be the only obstacle to overcome in moving toward becoming an Analytical Innovator. Leaders at Bank of Montreal acknowledge that traditional structures and silos within an organization, while necessary for fostering deep expertise, can be an impediment to enterprisewide coordination. “Everyone is interested in analytics, and so they should be,” says Bieda. “The question of where it resides and how it interfaces with business groups is continuous. It needs to fit with an organization’s strategy and goals for it to make sense. There are things which make sense to run at the center and things which are best managed in localized teams, but that tension is always present as organizations seek to find that balance.”
At Wescom, a dramatic restructuring around business analytics aimed to allow for broader access. The credit union instituted a single data platform across the organization in an effort to maximize the flow of data and increase the speed of data-driven customer response. “We are setting up a daily flow of all data sources, whether they’re our system or a third-party system, into our analytical data platform,” says Gumpert-Hersh. “The analytical data platform has these models embedded and can continually run and update our customers’ behavior and choice profiles.”
According to Lenovo’s Hu, it is essential to open up the analytics conversation beyond limited functional objectives to design solutions that provide sustainable value. Lenovo’s technical experts, for example, weren’t accustomed to taking an empathetic, customer-service point of view. “They were more used to saying, ‘We can take a call. We can route a ticket, and if customers are not happy, get a support ticket to help the escalation.’ It was about completing transactions and not delivering the right outcome that will help customers walk away feeling better about Lenovo,” says Hu.
Effective use of multiple data sets to improve customer engagement depends on having processes and policies that allow data sharing. Without effective data-sharing practices, integrating and updating data sets may not be quick enough to achieve customer engagement goals, such as optimal customer-service response times. In heavily siloed organizations, data sharing may be harder to achieve than in other kinds of organizations. One leadership task is to identify data bottlenecks within the organization and support cross-functional data sharing.
Tricky Metrics
As more corners of an organization take up the analytics mantle, it’s important to examine the practices and particulars in carefully defining the measures on which to focus. One interviewee references disparate metrics as a common pitfall — even when people across an organization are using a common data set: “We spend a lot of time on redefining our metrics. We focus on what we want to achieve and then have to align on how to measure those successes. How do we know we’re successful or not? And then we start thinking about the proxies. Do we have a measure? Do we know what the drivers are?”
Redfin Corp. offers brokerage services while operating one of the largest real estate databases in the United States. CEO Glenn Kelman focuses another aspect of metrics definition in terms of encouraging individual stakeholders and line-of-business executives to set their own goals — discover their own metrics — that are designed to improve the business, instead of just pleasing their bosses. “I don’t listen to people when they say, ‘It’s unrealistic for me to set my own goals’ or ‘I can’t really understand these numbers,’” Kelman notes. “Someone will say, ‘I can’t really increase traffic to our website because I don’t control our advertising budget.’ And then the person running the advertising budget says, ‘I don’t control the site.’ You just have to get used to the idea that you never control everything, so part of that is just structuring a metric to exclude ad-driven traffic.”
Data Quality
A perennial caution is paying careful attention to the integrity of the data you are collecting and analyzing. Analytical Innovators understand the critical nature of data cleansing and invest accordingly. “The quality of the data is really everything. You have to be vigilant,” says Oberweis Dairy’s Bedford, noting that this has remained a mainstay challenge for a long time. Oberweis, for example, ensures that its customer-service representatives understand how critical accurate data entry is to the company’s survival. “One of the easiest ways to do that is to show them how the data is used and communicate through the analytics itself,” says Bedford, who uses visual outputs to reinforce the point with field representatives. “When I can show somebody a very interesting retention map, for example, I find that they are a lot more motivated to make sure the data they enter is clean, because they begin to take ownership of that map and they want it to be accurate.”
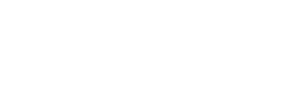
Governance in Complex Environments
These enduring challenges clearly demonstrate that increasing the use of data and analytics to bolster customer engagement and gain competitive advantage is no easy task. However, the task may be even more complicated if leaders are to be successful in using data to deepen customer engagement. Why? Technology change over time has resulted in clever solutions to yesterday’s problems, while also making operating environments more complex. Leaders need to pay extra attention to the following issues:
- Using data to deliver more tailored offerings increases the need for better data security. Loyalty gains from data-driven customized offerings can quickly evaporate if customer data gets into the wrong hands. One critical leadership task is to ensure that cybersecurity investments and attention are aligned with marketing uses of data and analytics (along with other departments’ uses of customer-related data). The advent of IoT, AI, and cloud computing has heightened the importance of this issue. Developing a system that identifies your company’s most critical data, where it is located, and who has access to it can be a critical first step. Beyond IT investments, has your company’s board of directors reviewed or audited its crisis response protocol for data breaches?
- Relatedly, companies need strong data governance practices to ensure that customer data is used appropriately by anyone with legitimate access to it. For instance, companies that rely on a developer community may offer customer data to developers to help them refine their products. But third-party uses of that data need to be governed as well. Critics have pointed out that Facebook’s data governance practices should do a better job of regulating developers’ use of Facebook data.7 In addition, Europe’s new data protection regulations have worldwide implications for any organization that collects and uses customer data.8
When these practices touch strategic business concerns, who has the responsibility for tackling these issues? While some companies have established cross-functional data governance councils, governance, like security, is tempting to ignore until it’s too late.
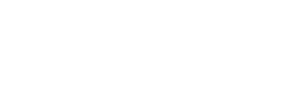
About the Research
MIT Sloan Management Review’s eighth annual data and analytics survey, conducted in 2017, reveals a continued rise in the number of companies reporting that their use of analytics helps them stay ahead of the competition. These survey results include responses from 1,919 managers, executives, and data professionals from companies around the globe. Survey respondents came from a number of sources, including MIT alumni, MIT Sloan Management Review subscribers, and other interested parties.
To provide further insight and context, we interviewed 17 subject matter experts from a number of industries, who uncovered some of the practical issues organizations face while using analytics. We have incorporated their perspectives in our assessment of how analytics is currently being used in a variety of organizations.
In this report, the term analytics refers to the use of data and related business insights developed through applied analytical disciplines (for example, statistical, contextual, quantitative, predictive, cognitive, and other models) to drive fact-based planning, decisions, execution, management, measurement, and learning.