A Practical Guide to Gaining Value From LLMs
Getting a return from generative AI investments requires a systematic approach to analyzing appropriate use cases.
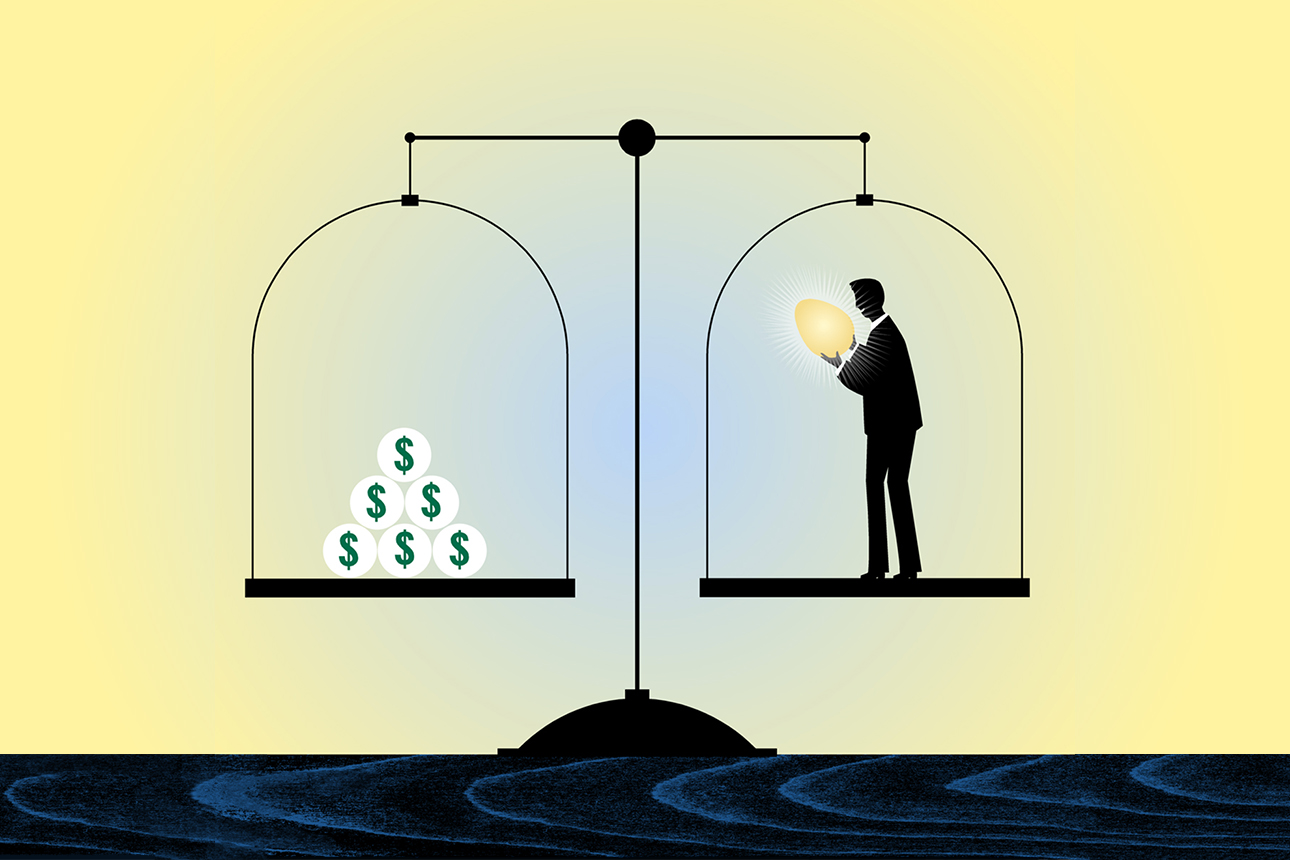
Michael Austin/theispot.com
When large language models exploded onto the scene in 2022, their powerful capabilities to generate fluent text on demand seemed to herald a productivity revolution. But although these powerful AI systems can generate fluent text in human and computer languages, LLMs are far from infallible. They can hallucinate information, exhibit logical inconsistencies, and produce irrelevant or harmful outputs.
While the technology has been widely disseminated, many managers are struggling to identify LLM use cases where productivity improvements outweigh the costs and risks of the tools. What’s needed is a more systematic approach to effectively utilizing LLMs to increase the efficiency of a business process while mitigating their shortcomings. I recommend an approach that involves three steps. First, disaggregate the process into discrete tasks. Second, assess whether each task satisfies the generative AI cost equation, which I’ll explain in this article. When a task meets that requirement, launch a pilot project, iteratively evaluate the results, and make changes to improve the outputs when necessary.
The core of this approach rests on developing a clear-eyed understanding of how the strengths and weaknesses of LLMs map to the nature of the task in question, the techniques by which LLMs are adapted to improve their performance on a task, and how all of this shapes the cost-benefit analysis — and the risk-reward picture — for using LLMs to increase the efficiency of the task.
LLMs: Remarkable Strengths, Surprising Weaknesses
When we experience LLMs responding with humanlike fluency to a prompt, it’s easy to forget that they can get simple questions wrong. If you ask even an advanced, large-scale model like GPT-4 the question “What is the fifth word of this sentence?” the answer will often be incorrect, as in, “The fifth word of the sentence ‘What is the fifth word of this sentence?’ is ‘fifth.’”1
Another example: “I have two coins in my pocket, and they add up to 30 cents. One of them is not a nickel. What are the coins?” GPT-4 provides a seemingly well-reasoned explanation but ultimately gives the wrong answer: “The coins in your pocket are a penny and a nickel. The trick in the riddle is the statement ‘One of them is not a nickel,’ which does not mean that both coins are not nickels.
References (15)
1. Credit for this example is due to X user Dean Buono (@deanbuono); credit for subsequent examples in this section is due to Colin Fraser (@colin_fraser).
2. L. Berglund, M. Tong, M. Kaufmann, et al., “The Reversal Curse: LLMs Trained on ‘A Is B’ Fail to Learn ‘B Is A,’” arXiv, submitted Sept. 21, 2023, https://arxiv.org.
Comment (1)
Jaideep Ghosh