AI-at-Scale Hinges on Gaining a ‘Social License’
Businesses must make explicit efforts to build trust with all stakeholders if AI implementation is to succeed.
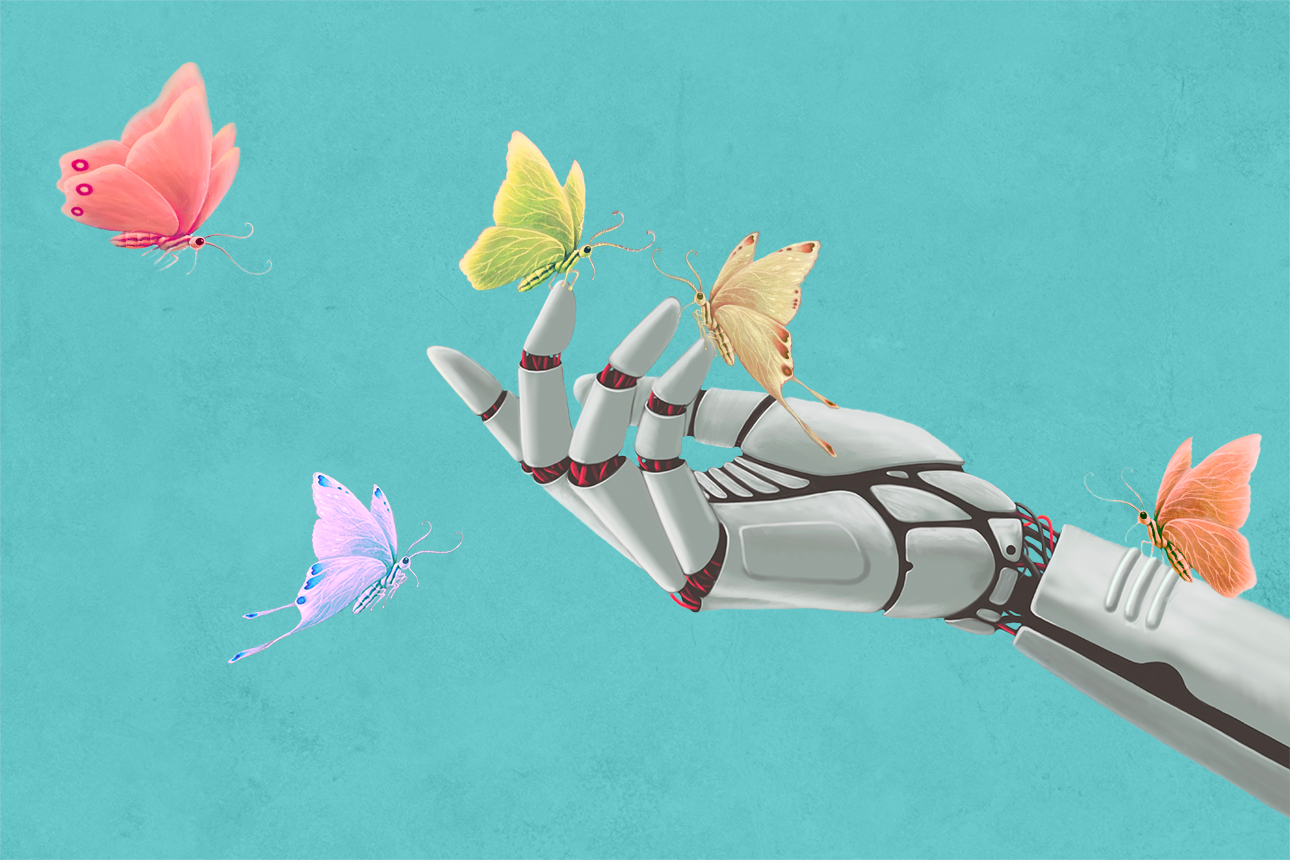
In January 2020, an unknown American facial recognition software company, Clearview AI, was thrust into the limelight. It had quietly flown under the radar until The New York Times reported that businesses, law enforcement agencies, universities, and individuals had been purchasing its sophisticated facial recognition software, whose algorithm could match human faces to a database of over 3 billion images the company had collected from the internet. The article renewed the global debate about the use of AI-based facial recognition technology by governments and law enforcement agencies.
Many people called for a ban on the use of the Clearview AI technology because the startup had created its database by mining social media websites and the internet for photographs but hadn’t obtained permission to index individuals’ faces. Twitter almost immediately sent the company a cease-and-delete letter, and YouTube and Facebook followed suit. When the COVID-19 pandemic erupted in March 2020, Clearview tried to pitch its technology for use in contact tracing in an effort to regain its credibility and gain social acceptance. Although Clearview’s AI technology could have helped tackle the crisis, the manner in which the company had gathered data and created its data sets created a social firestorm that discouraged its use.
Get Updates on Leading With AI and Data
Get monthly insights on how artificial intelligence impacts your organization and what it means for your company and customers.
Please enter a valid email address
Thank you for signing up
In business, as in life, being responsible is necessary but far from sufficient to build trust. As exemplified by the controversies around some corporations’ AI applications — such as Amazon, which had to terminate its experiment with a resume-screening algorithm, and Microsoft, whose AI-based chatbot was a public relations disaster — society will not agree to the use of AI applications, however responsibly they may have been developed, if they haven’t a priori earned people’s trust.
Rational people have a variety of concerns about AI, including the algorithmic institutionalization of income, gender, racial, and geographic prejudices; privacy concerns; and political issues. Indeed, Georgetown University’s Center for Security and Emerging Technology and the Partnership on AI last year launched the AI Incident Database to record cases in which intelligent systems have caused safety, fairness, or other real-world problems; as of July, it listed 1,200 publicly reported cases of such AI failures from the past three years.