How Developers Can Lower AI’s Climate Impact
AI development can be a power-hungry process, but there are tactics for reducing energy use and carbon emissions.
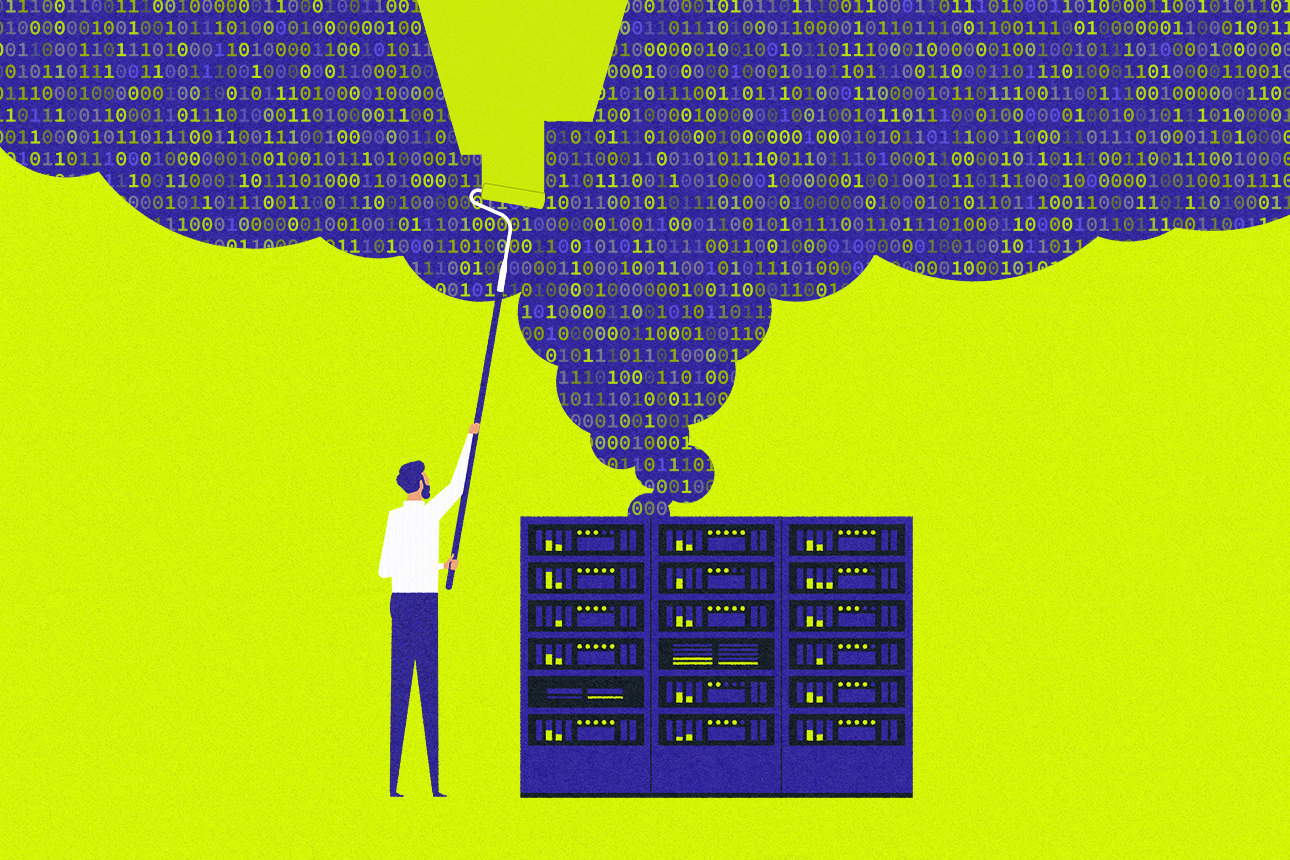
Carolyn Geason-Beissel/MIT SMR | Getty Images
AI is booming. The public release of large language models like ChatGPT has popularized the technology, which was already becoming a critical driver of companies’ efforts to innovate and grow. But as these models get bigger, so too does their appetite for energy: Training the open multilingual language model BLOOM produced nearly 24.7 tons of carbon emissions. AI itself might be a valuable tool for helping to find opportunities for sustainability improvements, but it could also become a drag on collective efforts to mitigate the global climate emergency.
Managers know that accurate metrics are the starting point for getting a handle on any problem, but it’s not easy to estimate the energy consumption of AI and machine learning (ML) models. Most AI companies don’t measure and disclose this parameter. Energy consumed during deployment is even less well understood than consumption during training.
Get Updates on Leading With AI and Data
Get monthly insights on how artificial intelligence impacts your organization and what it means for your company and customers.
Please enter a valid email address
Thank you for signing up
There are tools available to help. The Software Carbon Intensity specification from the Green Software Foundation outlines a reliable approach for determining a carbon emissions baseline that can then be used for comparison over time or across applications. The Green Algorithms project offers a simple calculator to estimate the total emissions of an AI project. Amazon Web Services, Google Cloud Platform, and Microsoft Azure offer carbon accounting tools specific to their cloud services. Researchers at Stanford, working with industry stakeholders, have published a lightweight framework for reliable, simple, and precise reporting of the energy, compute, and carbon impacts of machine learning systems.
Taking a Life-Cycle Approach to Mitigation
While measurement can reveal the status quo and help organizations track their progress on efforts to improve, actually moving the needle on AI-related carbon emissions requires addressing each step of the development, implementation, and adoption life cycle.
Moving the needle on AI-related carbon emissions requires addressing each step of the development, implementation, and adoption life cycle.
Different frameworks are emerging to meet this need. A joint study by Google and the University of California, Berkeley demonstrated that the energy consumption of ML training can be lowered up to 100x and CO2 emissions up to 1,000x by applying