Use Open Source for Safer Generative AI Experiments
Commercial AI services can put proprietary data at risk — but there are alternatives.
Topics
Frontiers
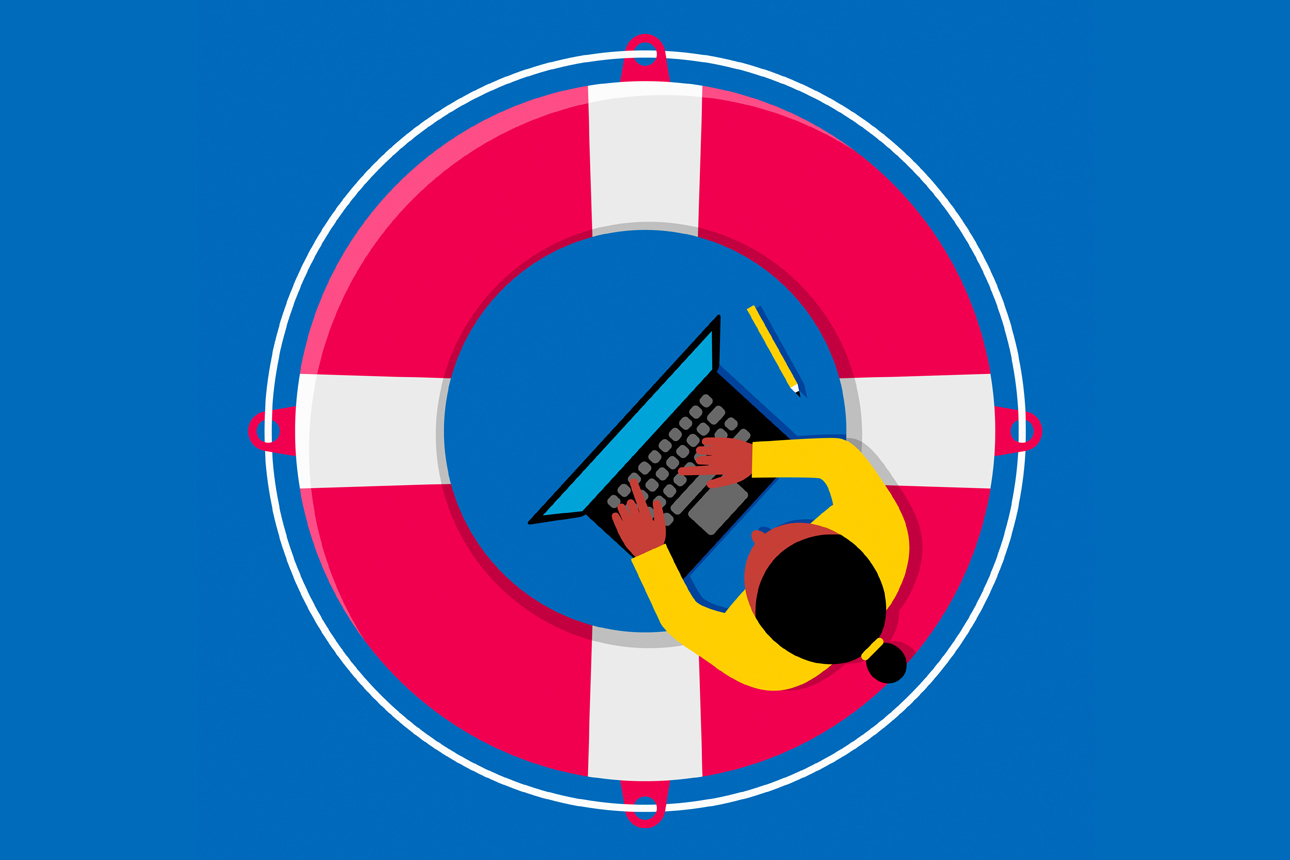
Patrick George/Ikon Images
Integrating artificial intelligence into the daily workflow of employees across organizations, from upper management to front-line workers, holds the promise of increasing productivity in tasks such as writing memos, developing software, and creating marketing campaigns. However, companies are rightly worried about the risks of sharing data with third-party AI services, as in the well-publicized case of a Samsung employee exposing proprietary company information by uploading it to ChatGPT.
These concerns echo those heard in the early days of cloud computing, when users were worried about the security and ownership of data sent to remote servers. Managers now confidently use mature cloud computing services that comply with a litany of regulatory and business requirements regarding the security, privacy, and ownership of their data. AI services, particularly generative AI, are much less mature in this regard — partly because it is still early days, but also because these systems have a nearly inexhaustible appetite for training data.
Get Updates on Leading With AI and Data
Get monthly insights on how artificial intelligence impacts your organization and what it means for your company and customers.
Please enter a valid email address
Thank you for signing up
Large language models (LLMs) like OpenAI’s ChatGPT have been trained on an enormous corpus of written content accessed via the internet, without regard for the ownership of that data. The company now faces a lawsuit from a group of bestselling authors, including George R.R. Martin, for having used their copyrighted works without permission, enabling the LLM to generate copycats. Proactively seeking to protect their data, traditional media outlets have engaged in licensing discussions with AI developers; negotiations between OpenAI and The New York Times, however, broke down over the summer.
Of more immediate concern to companies experimenting with generative AI, however, is how to safely explore new use cases for LLMs that draw on internal data, given that anything uploaded to commercial LLM services could be captured as training data. How can managers better protect their own proprietary data assets and also improve data stewardship in their corporate AI development practice in order to earn and maintain customer trust?
The Open-Source Solution
An obvious solution to issues of data ownership is to build one’s own generative AI solutions locally rather than shipping data to a third party. But how can this be practical, given that Microsoft spent hundreds of millions of dollars building the hardware infrastructure alone for OpenAI to train ChatGPT, to say nothing of the actual development costs? Surely, we can’t all afford to build these foundational models from scratch.