Avoid ML Failures by Asking the Right Questions
Machine learning solutions can miss the mark when data scientists don’t check their assumptions. Adopting a beginner’s mindset in any domain can help.
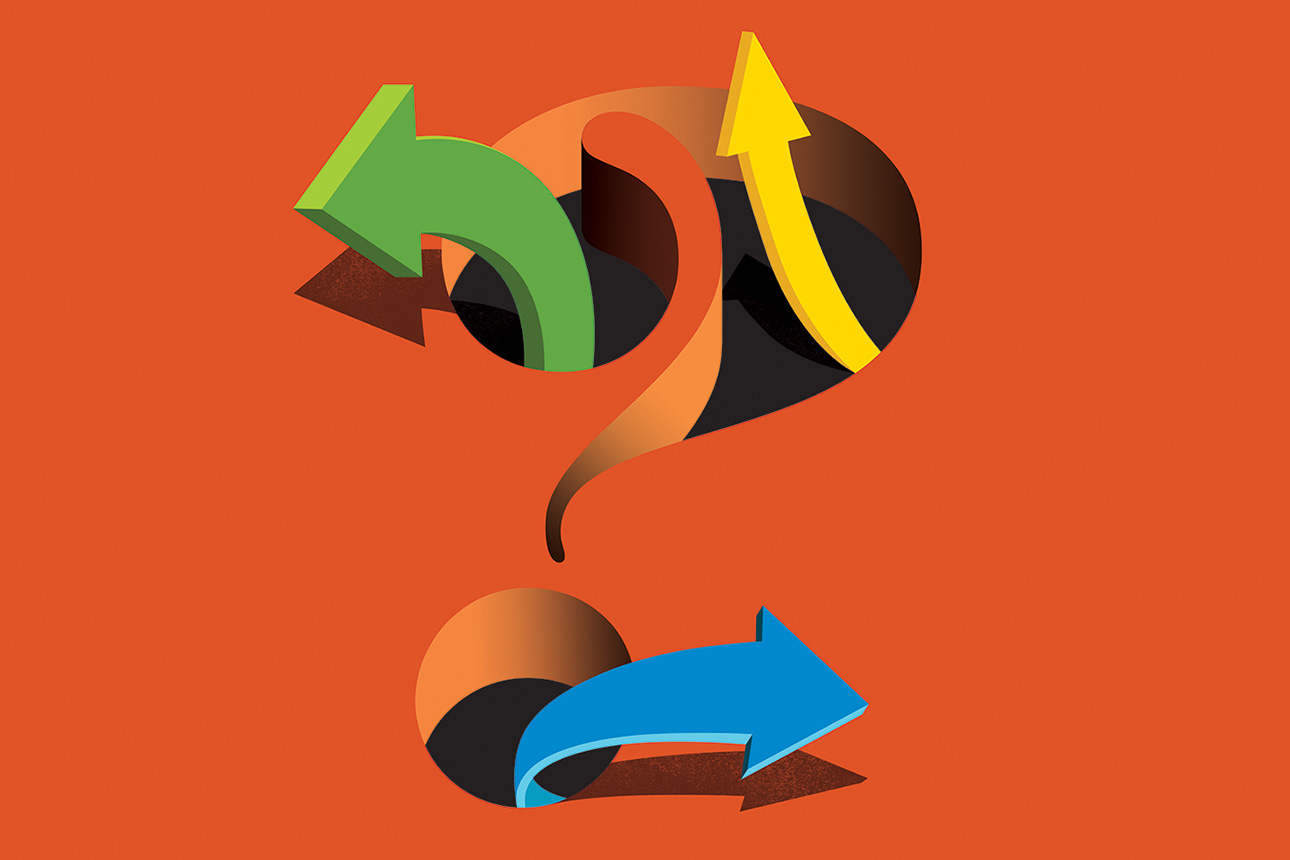
Paul Garland
In our collective decades of experience building, leading, and studying companies’ machine learning (ML) deployments, we have repeatedly seen projects fail because talented and well-resourced data science teams missed or misunderstood a deceptively simple piece of the business context. Those gaps create obstacles to correctly understanding the data, its context, and the intended end users — ultimately jeopardizing the positive impact ML models can make in practice.
We have discovered that small mistakes and misunderstandings are much less likely to cascade into failed projects when development teams engage with colleagues on the business side and ask enough questions to deeply understand the process and the problem at hand. Asking questions might seem like a simple step, but that might not be part of a company’s, team’s, or an industry’s culture. Appearing to be in command of all the information needed may be one of the ways employees signal competence in the organization. And while data scientists might possess technical mastery, they can lack the soft skills to reach a deep, accurate mutual understanding with business partners.
Get Updates on Leading With AI and Data
Get monthly insights on how artificial intelligence impacts your organization and what it means for your company and customers.
Please enter a valid email address
Thank you for signing up
At the same time, business partners often hesitate to ask questions themselves and don’t necessarily know what information or context would be helpful to share with a data science team. It’s hard work on both sides to have the kinds of interactions that allow everyone to surface and question assumptions, and identify the most important elements of business context.
Setting ML projects up for success with those kinds of useful interactions requires leaders to foster a culture that normalizes and values asking questions with a beginner’s mindset — and putting aside ego and past expertise. One data scientist we’ve worked with became very intentional about this after noticing that he makes the fewest mistakes when he is in a new context and must ask a lot of questions. But what are the right questions to ask? In this article, we present three examples where significant ML projects failed and explore how asking the right questions with a beginner’s mindset could have improved the interactions between data scientists and business partners, and helped their ML deployments succeed in creating business value.