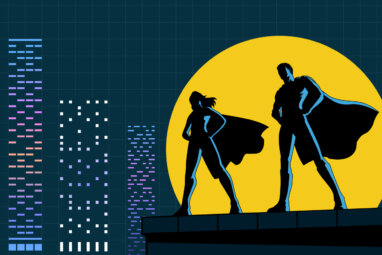
AI & Machine Learning
AI and Statistics: Perfect Together
Business leaders can identify and avoid flawed AI models by employing statistical methods and statistics experts.
Business leaders can identify and avoid flawed AI models by employing statistical methods and statistics experts.
Is your AI project using the right data, and is it set up to succeed? Ask the right questions to head off failure.
Employees in connector roles can bridge the gaps between departments that often thwart data science project success.
Data science project failure can often be attributed to poor problem definition, but early intervention can prevent it.
The collective intelligence of remote teams, synthetic data for machine learning, and delegation to bridge virtual distance.
AI techniques can generate training data that retains the predictive power of real-world data.
Kay Firth-Butterfield (the World Economic Forum), Ya Xu (LinkedIn), and Charlotte Degot (BCG GAMMA) join MIT SMR senior project editor Allison Ryder for a discussion on innovating with artificial intelligence.
New research highlights nine key factors impeding organizations’ ability to advance their data science progress.
Organizations that struggle to gain payback from data science efforts can recognize and overcome five common obstacles.
Bridge-building for business and data teams, responsible AI practices, and smart time management.
Using AI and simulations in health care can help doctors better serve patients.
To drive major change, companies must link data quality and data science within the organization.
Leaders must focus on managing the gaps in AI skills and processes within the organization.
A successful AI-enabled workforce requires key hiring, training, and risk management considerations.
Shareholders and stakeholders, data science’s pandemic shift, and combating workplace discrimination.
Companies are beginning to reboot their machine learning and analytics, disrupted by the global pandemic.
How U.S. companies are commemorating Juneteenth; what a 2020 recession may mean for data analytics.
There has been a huge demand for data scientists in the past decade. Is that about to change?
It’s early in the age of experimentation — and the right time to start building expertise.
To solve the issue of advanced analytics talent concentration, companies need to think creatively.