Building a Data-Driven Culture: Three Mistakes to Avoid
When people resist changing the way they make decisions, well-intentioned data science projects are doomed. Here’s how to overcome the key challenges.
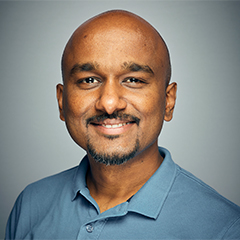
Topics
Column

Carolyn Geason-Beissel/MIT SMR | Getty Images
When one of the largest global telecom companies grappled with high customer attrition, the marketing team used a heuristics-driven approach to retain customers. For example, if a customer hadn’t made any outgoing calls in three weeks, the company would roll out a promotion.
However, this approach didn’t deliver results, and customer retention was at its lowest in years. Based on the weekly business performance reviews, the CEO knew it was time to try something different. He turned to data science tools and a cross-functional effort to solve the retention challenge.
The marketing team deployed machine learning algorithms to study customer usage patterns and predict churn. Simple techniques like decision trees helped spot factors such as billing amounts and outgoing call patterns — both good predictors of a customer’s propensity to leave.
Get Updates on Transformative Leadership
Evidence-based resources that can help you lead your team more effectively, delivered to your inbox monthly.
Please enter a valid email address
Thank you for signing up
Tests run on historical data indicated that this approach could improve customer retention by 39% — a hopeful sign. Then the data science team brought in more advanced AI firepower, adding techniques such as neural networks for deeper pattern-spotting.
This approach turned out to be far more accurate and effective, with the potential to improve customer retention by 66% — a considerable uplift. A four-week pilot run with high-value customers confirmed the results.
The data science solution looked ready for a full rollout. Then things turned south.
The marketing product managers refused to use the solution. They found it hard to trust an algorithm that spat out a list of at-risk customers with little explanation. What’s more, many of the data-backed recommendations were counterintuitive. For example, even some customers with long tenures and steady usage were flagged by the algorithm as high risk.
The entire process felt wrong to the managers. Despite the strong pilot results, the users gave the data science solution the cold shoulder.
If you’re wondering whether this is unusual, I can assure you that it is not. The graveyard of data science initiatives is filled with solutions that are advanced, accurate, and well-meaning yet unused.
A company’s investment in multiple data and analytics projects won’t, on its own, result in employees using data insights to make their own decisions. This end goal requires leaders to take different types of interventions. And it’s hard work: In a recent survey of Fortune 1000 CIOs and data executives by NewVantage Partners, about 6 in 10 leaders acknowledged that they haven’t been able to establish a data- and analytics-driven culture.
Why is it so hard? How can leaders foster an environment where decision-making with data insights becomes a habit? Let’s uncover the root causes of failure and examine three practical ways for leaders to nurture a data-driven culture.
Three Factors That Lead to Failure
I’ve seen common failure patterns emerge among doomed data analytics initiatives during my past decade of global consulting work.
Despite a heavy focus on analytics tools and algorithm accuracy, most initiatives struggle to deliver actionable insights. Even when the insights shared are actionable, people are often not excited about using them. Finally, projects that do see meaningful adoption often lose steam after road shows end — and then die slowly.
Chances are you’ve encountered such failure stories. However, addressing these head-on won’t yield much improvement: These are just symptoms of the problem, not the root causes.
Three core factors cause those symptoms:
Most initiatives are run as technology projects. Often, the entire journey starts as a data science initiative or carries a technical name such as predictive analytics project. This alienates business teams. Given the weak link to business users, the project may not address the most significant problems — or real challenges.
Users resist changes to ways of working. Most humans are wired to seek comfort in the familiar. This inertia can be dangerous for analytics initiatives, which almost always involve changes to business processes or decision-making methods. This change resistance is amplified by fear of AI-driven job loss, an emotion that runs high today.
It’s difficult to demonstrate analytics ROI. Despite data’s immense effectiveness, it is often tough to attribute business outcomes to analytics efforts. Since several factors could drive revenue increases or cost savings, linking them to data-driven decisions may not always be possible. Consequently, the inability to quantify ROI can push data initiatives into a downward spiral, with project leaders squandering momentum and losing funding.
How to Build a Data-Driven Culture and Better Solutions
Business leaders can address the factors outlined above by taking the following actions.
1. Fix burning challenges and ensure business ownership.
How do your data initiatives start? Attempting to use up some available technology budget or coming up with pilots to try out the latest large language model could leave you with a technical solution that has limited impact.
Instead, identify key business function stakeholders, uncover their biggest priorities and pain points, and brainstorm ideas. Get clarity on the target users, and quantify outcomes: Will the initiative drive share of customer wallet or save inventory costs? Prioritize high-impact ideas that are reasonably feasible in terms of data availability, technology capability, and change management. Identify a business owner who will lead the initiative, be accountable, and influence user involvement — from project design through execution and ongoing usage. Here’s what that looks like in action.
Prioritize high-impact ideas that are reasonably feasible in terms of data availability, technology capability, and change management.
A European engineering giant needed help translating its ambitious, five-year business strategy into a powerful road map that would leverage data analytics in the most appropriate places. Instead of just fishing for analytics use cases, the CEO sought the ones that made the most sense. So they commissioned an advisory initiative with an initial scope spanning four business units. The units’ leadership identified more than 50 leaders across functions to prioritize goals and pain points.
This team came up with 150 ideas and worked with technology teams to map out their feasibility, time frames, and regulatory implications. They then pared down the ideas to 76 use cases that strongly aligned with nine organizational growth themes outlined in the company’s five-year strategy.
The resulting multiyear road map recommended kick-starting the journey with some quick-win projects that scored high on potential impact and feasibility. The technology teams picked the best approach for each project, whether it was data engineering, business intelligence, or AI algorithms. Summing up this cross-functional effort’s results, a divisional manager said the biggest win was in aligning the entire organization on the need for data-driven transformation, sparking ideas by showing the art of the possible, and quantifying the likely value that the enterprise could unlock.
2. Drive adoption with executive storytelling and gamification.
Successful organizational change begins with a clear, inspiring vision. The most effective transformations occur in companies where executives actively walk the data-driven talk. Leaders must communicate the vision and explain what it means for users — encouraging them to both adopt new habits and discard old ones.
Complementing such top-down support with grassroots, user-driven programs is critical. Involve employees by crowdsourcing ideas. Identify ambassadors who can share their stories and evangelize the work among peers. Gamify change with data quizzes, hackathons, or storytelling competitions across business functions to promote healthy rivalry and engagement.
Consider the example of an Asian media company, part of a global media and entertainment giant. It operates more than 60 television channels and produces more than 30,000 hours of content annually. Despite having access to vast amounts of data on viewership, consumer ratings, and enterprise advertising, the company struggled to derive timely insights on how to grow ad revenue.
The company rolled out a visual intelligence platform with deep insights and strong visual narratives, yet its adoption among sales teams was low. Then the leadership team introduced gamification by building leaderboards based on employees’ daily platform usage. Weekly winners were prominently featured on portals and in emails. The excitement was amplified by monthly giveaways for winners, with prizes such as iPads.
Additionally, leadership identified project champions to share insights in chat forums through screenshots and direct application links. This sparked conversations and drove usage. In a few months, user adoption had jumped to 64% and sales teams were able to make data-driven recommendations and better monetize inventory.
3. Identify success metrics early and rally teams around them.
The best time to define success criteria is while you are evaluating a project idea. When prioritizing projects, leaders need to define expected business outcomes, how success will be measured, and the data needed to compute ROI. Often, this data isn’t readily available; you might need to collect new customer feedback or develop alternate employee productivity metrics.
If you’re targeting incremental revenues, you might need to commission small experiments, such as A/B tests, to assess a project’s impact. To measure the effect of an AI-driven recommendation tool on cross-selling, for example, roll it out in select locations while maintaining the status quo in a similar one for comparison. Where this isn’t feasible, you could contrast business performance before and after the rollout, provided that the two scenarios were comparable.
A leading company operating cold chain warehouses across the U.S. aspired to improve truck utilization through optimized packing and routing to client locations. Given high travel costs, the company green-lighted the project and set a goal of optimizing daily load costs. The team ran with this singular objective throughout execution and collected data to measure the load-cost metrics.
Although variability across locations made A/B testing impractical, the team tried comparing costs before and after implementation. To address the impact of seasonality, the team innovated by building forecasting algorithms to estimate the likely costs without the tool intervention. Given access to extensive historical data, they could model the business-as-usual scenario with algorithms, while the new daily load costs were measured in the warehouses after the rollout.
After the attribution approach was meticulously validated by business experts, the solution was projected to save $4 million annually. When these forecasted results were presented with actual results for a few months, the ROI helped secure swift executive approval for a project expansion. The company immediately rolled out the project to the two biggest regions, promising even greater returns.
Building a Data Culture Is a Marathon
Culture is often defined as what people do in an organization when no one is watching. Changing deeply ingrained behaviors takes time, but with a committed start and ongoing reinforcement, it is indeed achievable.
The key to successful cultural transformations lies in the right mix of top-down leadership efforts and grassroots initiatives. The survey by NewVantage Partners referenced above found that only 28.3% of leaders reported having established a data-driven culture in 2019. Fast forward to today, and that number has increased to 42%. This type of change happens gradually: Think five years, not one or two.
In reality, building a data culture is a marathon. Leaders who prioritize executive support backed with meticulous execution will succeed with this transformation.