Generative AI at Mastercard: Governance Takes Center Stage
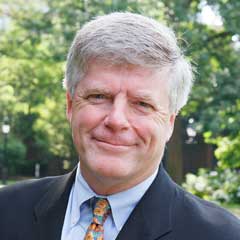
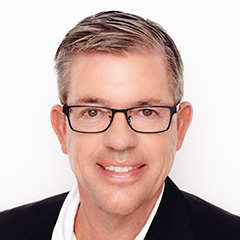
Topics
AI in Action
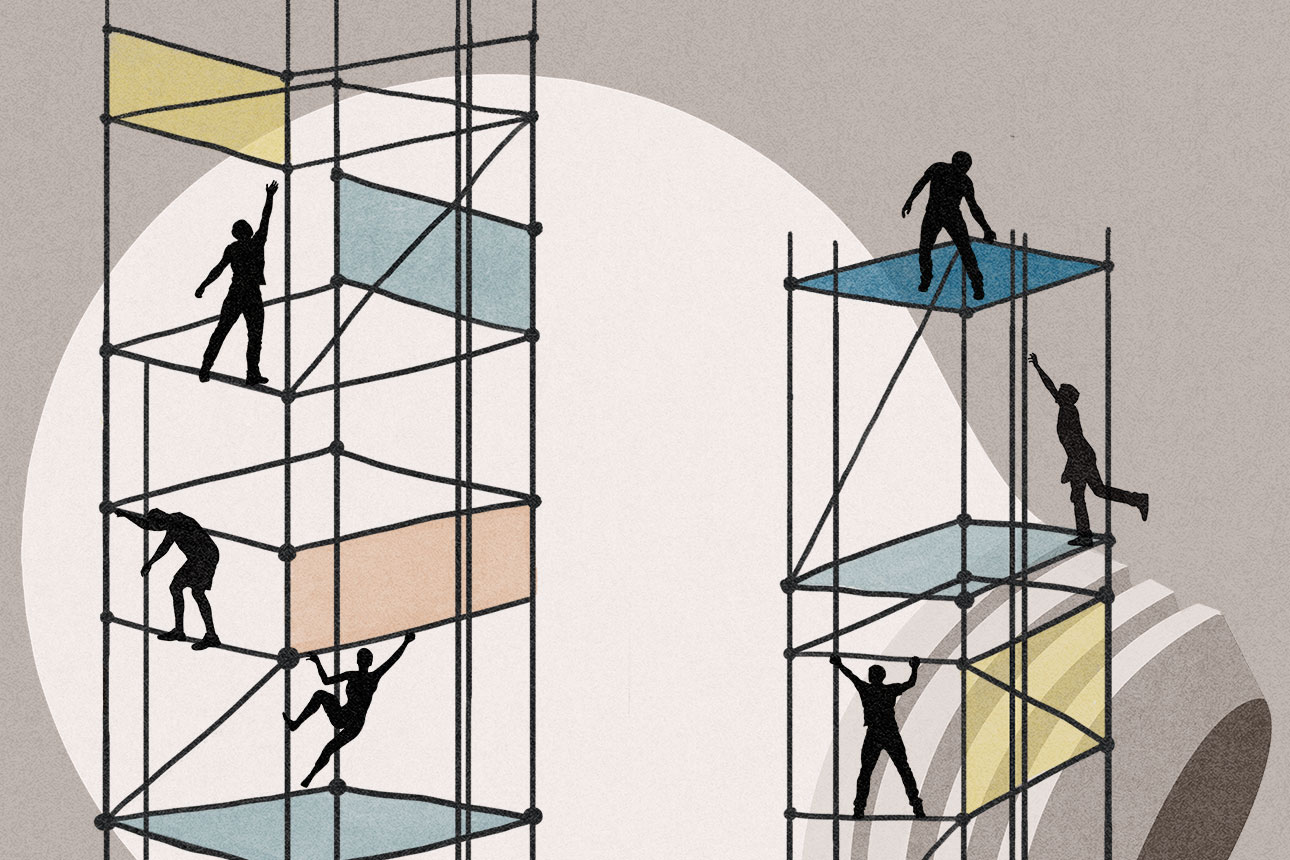
Carolyn Geason-Beissel/MIT SMR | Getty Images
If you saw the action-adventure movie Everything Everywhere All at Once, you might have had the same reaction we did. Impressive and exciting? No doubt — that’s one reason why it won seven Academy Awards. A portent of the future? Perhaps — as scientists explore the idea of a multiverse, the film provides one vision of what it might look like. Somewhat bizarre and confusing? Well, to us anyway.
JoAnn Stonier, until recently Mastercard’s chief data officer, made an apt comparison between the movie and generative AI when she appeared on a recent panel. “Everything everywhere” is a good way to characterize the technology — exciting, confusing, and important all at the same time. We have previously written about Stonier and her unusual position as one of the few CDOs who has been heavily focused on data ethics for many years. We interviewed her on one of her last days as CDO; she’s since become a Mastercard fellow but will still work on data and AI ethics issues.
Get Updates on Leading With AI and Data
Get monthly insights on how artificial intelligence impacts your organization and what it means for your company and customers.
Please enter a valid email address
Thank you for signing up
After the interview, we were somewhat relieved, as you might be, to find out that Stonier and Mastercard are still feeling their way with regard to generative AI. That’s true of many companies. More than half of the respondents to a recent VentureBeat survey said their organizations are experimenting with AI, but only 18% of those companies have begun implementing it. The same percentage said they expect to spend more on the technology in the coming year.
Of course, Mastercard is an old hand at data, analytics, and AI: We wrote about its efforts toward becoming an “AI powerhouse” a year ago, and Stonier discussed the company’s use of AI on the MIT Sloan Management Review podcast Me, Myself, and AI. Mastercard has been in the AI space for more than a decade, most significantly in the cybersecurity realm. That a leader and company so experienced with AI is still trying to work out the details of a generative AI strategy should be comforting to many other companies and managers.
What’s Already Clear
Mastercard’s extensive experience with previous forms of AI led it to build a robust methodology and governance process. At a high level, this process involves understanding the data, understanding the models, and reviewing the output and the related outcomes.
Each of those steps will come into the process for evaluating generative models, but they are likely to have a different flavor. Mastercard has no shortage of structured numerical data, of course, but the data that generative AI processes are often images and less-structured text. Given the complexity and size of generative models, understanding exactly how a given input produces a specific output is very challenging. That places even more importance on the step of reviewing outcomes, which need to be assessed for factual accuracy, biased or toxic language, and value to the user, as well as unintended consequences to individuals, organizations, and the ecosystems in which the outcomes might operate.
Despite the uncertainties inherent in the technology, Mastercard has already established some policies with regard to generative AI. Shortly after the introduction of ChatGPT in November 2022, the company put guidelines in place to ensure that employees innovate responsibly with the new technology. This encourages internal exploration of the models its vendor provides while safeguarding confidential company information.
Some companies have gone so far as to ban ChatGPT and the use of other large language models by employees, but Mastercard hasn’t been tempted to do so. “Some of the use cases have been more creative than others, but we haven’t had any problems thus far,” Stonier said. Perhaps that’s because, as she noted, “everybody in the company is more aware and digitally engaged now, and they see governance as part of everyone’s job.”
We think that virtually every company should be educating its senior executives and board members about generative AI, and Mastercard has been predictably active in that regard. It has held multiple sessions for the senior executive team and board members to address different aspects of the technology, including the opportunities it provides, the regulation it needs, and the process the company should follow in implementing it. Numerous outside experts have participated in these briefings, and Stonier noted that there are several tech-savvy board members who already understood the technology well.
Mastercard has an established council of AI-informed leaders from all areas of the business that evaluates AI use cases before their deployment, and it has added generative AI use cases to those that are reviewed. Some of the use cases developed so far involve areas like fraud detection, internal knowledge management, and personalization. Even more use cases are in the experimentation phase and are not yet intended for production deployment.
We think that virtually every company should be educating its senior executives and board members about generative AI.
Although detailed government policies for generative AI haven’t been issued yet for the U.S., Stonier said that it’s already clear that there will be different policies for the many different capabilities of generative AI. Because Mastercard depends heavily on reliable information systems, for example, using generative AI to develop programming code will be treated differently than using it to create marketing copy.
It’s also clear that an interdisciplinary and cross-functional approach will be required to manage generative AI at Mastercard. Already, lawyers, HR professionals, and systems and data engineers and architects are engaged in developing the company’s approach to the technology. As use cases become more customer-oriented (they are primarily internal now), it’s likely that more parts of the organization will become involved. External regulators will want to see how the company built any “black box” models, where the logic and data sources the models are built on aren’t readily visible to users. Stonier said that governance of generative AI is a challenge, given all of the possible use cases and aspects of the organization that are involved. She expects that, consistent with the previous AI development process, governance will have to address not only what the users of the technology are trying to achieve but also what the most likely outcomes will be.
What’s Still Evolving
Stonier said that many aspects of generative AI are still being discussed, as they should be. This is a period of experimentation for the company and its employees, and Stonier noted that no one wants to dampen it as long as the company’s data responsibility principles are being followed. Mastercard wants to use generative AI as an opportunity for learning and to eventually benefit from it as much as it has gained from conventional AI in its fraud-reduction and cybersecurity initiatives.
At the moment, the primary benefits being realized are internal productivity gains for the company’s own processes. Use cases for Mastercard’s customers, including merchants who accept Mastercard, will come later. There is the potential for highly personalized data analysis and messages for customers in the future.
Mastercard already has a well-defined process for rolling out data and data products and even a business unit — called Data & Services, which Tom wrote about a couple of years ago — for making them available to customers. Stonier believes that Data & Services and the company’s Cyber & Intelligence business will be the most likely to implement generative AI-based products in the future, adding to a wide array of existing AI solutions. She is confident that these generative AI uses will employ multiple types of language models and that they will make extensive use of humans in the loop, perhaps through reinforcement learning with human feedback. There will be extensive testing, she said, to ensure that there are no substantial hallucinations or other problematic outputs in the products. We think this is important; if machine learning is like analytics on steroids, generative AI is like machine learning on LSD. That captures the unpredictable aspect of the technology.
The specific architectures for generative AI models have also yet to be determined. Stonier feels that there will definitely be multiple models, but she’s not sure whether they will be stacked — combined for one use case — or arrayed, with a front-end system determining which is most appropriate for the context.
We asked Stonier whether she feels that generative AI is an incremental or hugely transformative technology. “It’s been incremental so far, but eventually it may be transformative,” she said. “But any transformation won’t be a big-bang moment — it will take place over time. Improvements in operating efficiency will come relatively quickly, but changes in products and services will take longer.”
If your company is wrestling with how to govern and fully take advantage of generative AI, Mastercard’s experience should make you feel somewhat more relaxed. This technology may or may not transform your business, but it certainly raises many new issues and opportunities. They don’t all need to be figured out overnight.