Manage AI Bias Instead of Trying to Eliminate It
To remediate the bias built into AI data, companies can take a three-step approach.
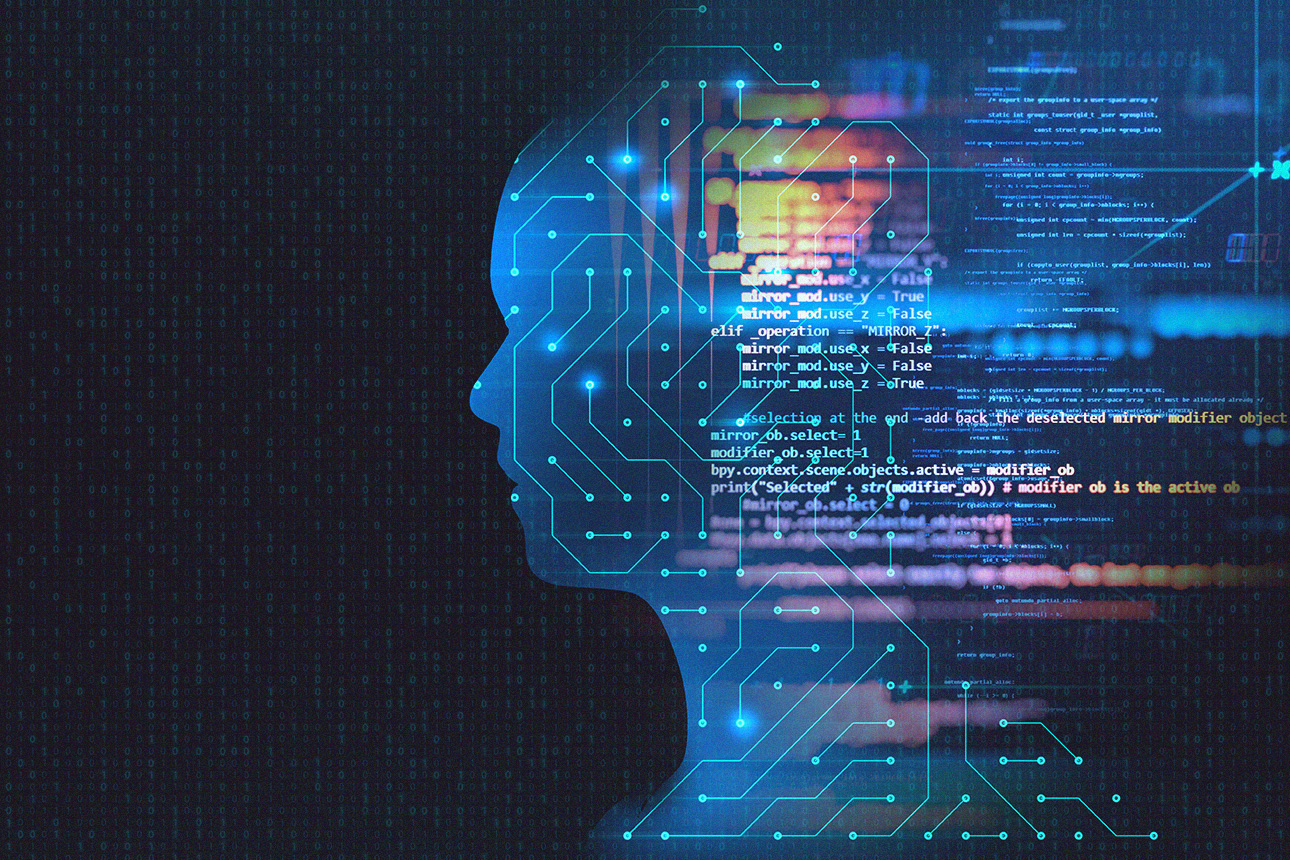
Businesses and governments must face an uncomfortable truth: Artificial intelligence is hopelessly and inherently biased.
Asking how to prevent such bias is in many ways the wrong question, because AI is a means of learning and generalizing from a set of examples — and all too often, the examples are pulled straight from historical data. Because biases against various groups are embedded in history, those biases will be perpetuated to some degree through AI.
Traditional and seemingly sensible safeguards do not fix the problem. A model designer could, for example, omit variables that indicate an individual’s gender or race, hoping that any bias that comes from knowing these attributes will be eliminated. But modern algorithms excel at discovering proxies for such information. Try though one might, no amount of data scrubbing can fix this problem entirely. Solving for fairness isn’t just difficult — it’s mathematically impossible.
Get Updates on Leading With AI and Data
Get monthly insights on how artificial intelligence impacts your organization and what it means for your company and customers.
Please enter a valid email address
Thank you for signing up
Hardly a day goes by without news of yet another example of AI echoing historical prejudices or allowing bias to creep in. Even medical science isn’t immune: In a recent article in The Lancet, researchers showed that AI algorithms that were fed scrupulously anonymized medical imaging data were nevertheless able to identify the race of 93% of patients.
Business leaders must stop pretending that they can eliminate AI bias — they can’t — and focus instead on remediating it. In our work advising corporate and government clients at Oliver Wyman, we have identified a three-step process that can yield positive results for leaders looking to reduce the chances of AI behaving badly.
Step 1: Decide on Data and Design
Since complete fairness is impossible, and many decision-making committees are not yet adequately diverse, choosing the acceptable threshold for fairness — and determining whom to prioritize — is challenging.
There is no single standard or blueprint for ensuring fairness in AI that works for all companies or all situations. Teams can check whether their algorithms select for equal numbers of people from each protected class, select for the same proportion from each group, or use the same threshold for everyone. All of these approaches are defensible and in common use — but unless equal numbers of each class of people are originally included in the input data, these selection methods are mutually exclusive.