Putting Responsible AI Into Practice
A survey of individuals driving ethical AI efforts found that the practice has a long way to go.
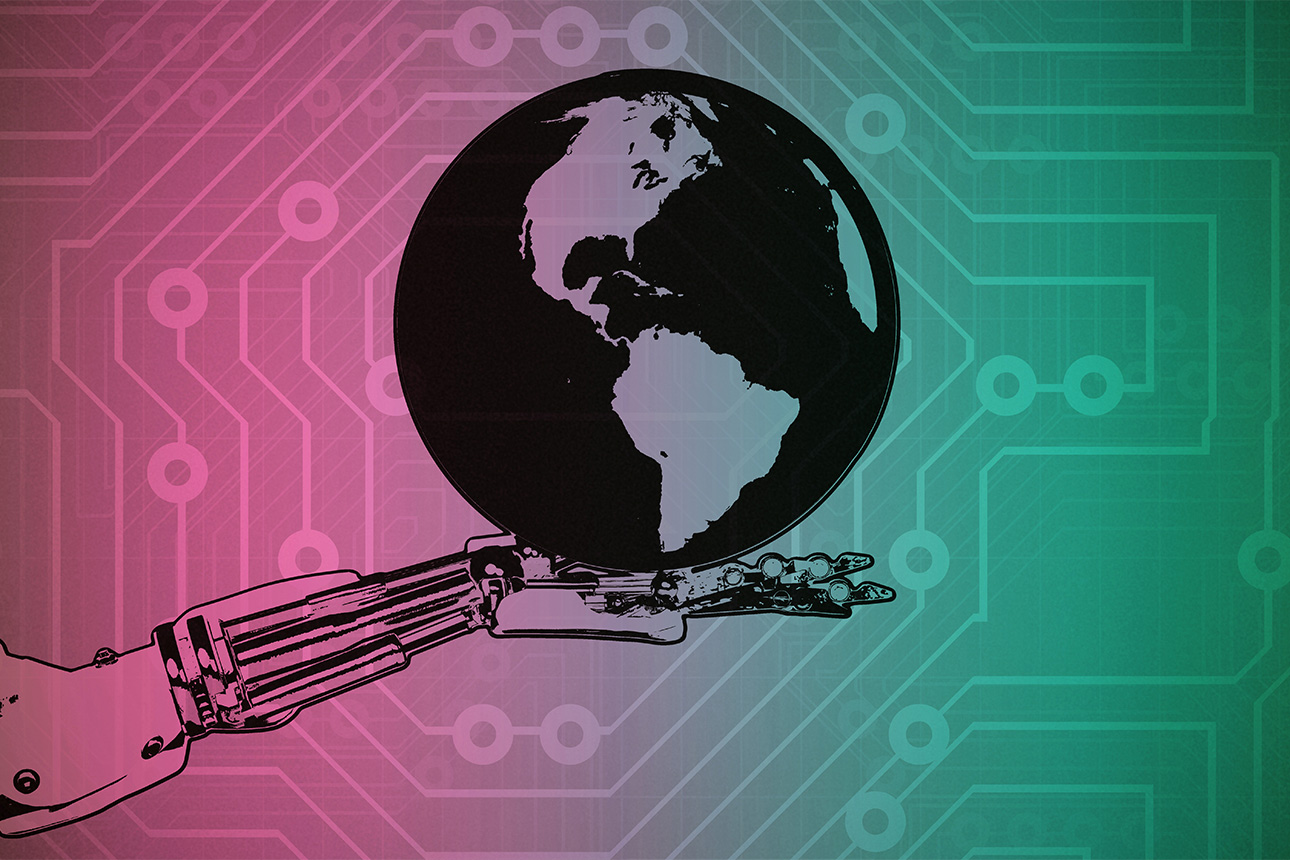
As awareness grows regarding the risks associated with deploying AI systems that violate legal, ethical, or cultural norms, building responsible AI and machine learning technology has become a paramount concern in organizations across all sectors. Individuals tasked with leading responsible-AI efforts are shifting their focus from establishing high-level principles and guidance to managing the system-level change that is necessary to make responsible AI a reality.
Ethics frameworks and principles abound. AlgorithmWatch maintains a repository of more than 150 ethical guidelines. A meta-analysis of a half-dozen prominent guidelines identified five main themes: transparency, justice and fairness, non-maleficence, responsibility, and privacy. But even if there is broad agreement on the principles underlying responsible AI, how to effectively put them into practice remains unclear. Organizations are in various states of adoption, have a wide range of internal organizational structures, and are often still determining the appropriate governance frameworks to hold themselves accountable.
Get Updates on Leading With AI and Data
Get monthly insights on how artificial intelligence impacts your organization and what it means for your company and customers.
Please enter a valid email address
Thank you for signing up
In order to determine whether, and how, these principles are being applied in practice, and to identify actions companies can take to use AI responsibly, we interviewed 24 AI practitioners across multiple industries and fields. These individuals with responsible AI in their remit included technologists (data scientists and AI engineers), lawyers, industrial/organizational psychologists, project managers, and others. In each interview, we focused on three main phases of transition: the prevalent state, which identified where the organization was as a whole with responsible AI; the emerging state, detailing the practices that individuals who were focused on responsible AI had created though they had not yet been fully integrated into the company; and the aspirational state — the ideal state where responsible AI practices would be common and integrated into work processes.
The State of the Field
Most of the practitioners we interviewed indicated that their companies’ approach to responsible AI has been mostly reactive to date. The primary motivators for acting on ethical threats come from external pressures, such as reputational harm or compliance risk. Often, media attention serves to further internal initiatives for responsible AI by amplifying reputational risk.
This reactive response is driven in part by a lack of metrics to assess the success of responsible-AI initiatives. Without standards for implementation, assessment, and tracking, it is difficult to demonstrate whether an algorithmic model is performing well from a responsibility standpoint. Where model performance metrics exist, they are generally not integrated with ethical touch points and rather focus on things like efficiency, engagement gains, and short-term profitability.
Interviewees reported being measured on productivity and contributions to revenue, with little value placed on preventing reputational or compliance harm and mitigating risk. Understandably, practitioners find it difficult to measure and determine the value of long-term benefits to the organization when they come at the expense of short-term product success as measured by deliverables and revenue.
In order to succeed, responsible-AI efforts need more organizational support in the form of governance and accountability, and coordinated participation from a range of relevant stakeholders such as legal, HR, communications, design, and engineering.
The majority of interviewees reported misalignment between individual-, team-, and organizational-level incentives and mission statements. A lack of organizational governance of AI systems leads to competing incentive structures and power plays. In most cases, these ambiguities created conflict among teams as each competed to show value across metrics that were at odds with each other. With no clear ownership or governance structure, problems remain unresolved and organizational inertia discourages many of those tasked with responsible-AI efforts from continuing to pursue their work.
Where Are We Heading in the Short Term?
As the field matures, internal and external levers are beginning to enable more forward-thinking processes. Practitioners we spoke with are cautiously optimistic about the near-term future of responsible AI.
One bright point is the emergence of more internal processes for communicating about and overseeing ethical risks in AI. Internal cross-functional reviews of responsibility and fairness aspects of AI were often the result of grassroots action from within the organization. One participant shared that they used to take screenshots of problematic algorithmic outcomes and circulate them among key internal stakeholders to support the case for responsible-AI oversight. Respondents also reported a growing number of educational initiatives for training employees on responsible-AI practices such as fairness, transparency, and “explainability.”
Although education is an important element, it is still just one piece of the overall picture. Organizations must have clear processes in place for raising, investigating, and addressing issues related to responsible AI. Just as organizations have established clear channels for raising HR, compliance, and even broader ethical concerns, there must be a pathway for reporting issues with how AI is used.
Responsible AI should also become part of employee performance discussions. The implementation of appropriate success metrics for individuals was repeatedly identified as an area to target for development. As companies hire more people to staff responsible-AI roles, these individuals may sit in human resources, legal, engineering, IT, or marketing, depending on the organization. Given the diversity of primary job functions and reporting structures, it’s important to clearly define how employees in each of these areas are expected to foster responsible AI within the organization. Providing career incentives to act ethically will help drive success.
What Is the Future of Responsible AI?
When asked what an ideal future state would look like, interviewees preferred an approach that anticipates rather than reacts to risk. In order to achieve that, organizations need standard processes, communication, and transparency.
To the first point, they must provide a framework that explains responsible-AI processes but is both general and flexible enough that it can be applied to different contexts. For example, ensuring that a framework identifies and mitigates bias for an employment model may mean very different things (from a legal and ethical perspective) than mitigating bias in a video recommendation algorithm.
They must also ensure that communication around these issues is understandable to multiple audiences, whether the legal and compliance team, nontechnical stakeholders, or end users. And finally, processes must be transparent. Identifying not only accountable parties (plural) but also clearly defining the scope of what they are responsible for enables confidence in adopting responsible-AI practices. For end users, this may mean being clear about how decisions were arrived at and how they can refute or report incorrect outcomes.
According to our experts, in an ideal future state, both product and person metrics are intertwined. An ideal state is one in which the organization employs a data-informed approach to managing ethical challenges that celebrates proactive pioneers, qualitative work, and harder-to-measure innovations that pay off in longer timelines. For products, this may mean expanding the time frame by which performance goals are mapped, appreciating that ethical considerations may require a different or longer amount of time. For individuals, this would mean respecting the variety of skill sets required to ensure true responsible-AI implementation and rewarding innovative behavior.
An ideal state of organizational culture is one in which there is no fear of retribution or harm for internally reporting ethical issues, there are clear channels through which issues can be escalated, and teams are able to bring in individuals with specific expertise as needed. Leadership must articulate values and principles, make it clear that practices must be aligned with these values, and provide resources to support those practices.
How Do We Get There?
In bringing their principles to practice, companies can take a few key steps.
First, they must create organizational transparency and diffuse accountability. This can be achieved by strong governance methodologies and a clear chain of command and serves to resolve tensions, create appropriate priorities, and move from a reactive to an anticipatory response to ethical harm. Many companies have moved to create AI review boards, for example, consisting of leadership from different parts of the company. Next steps are to create governance structures and identify roles and expectations around responsible-AI practices. What are the steps and who are the people to best identify and mitigate potential harm at all stages of the project development process?
Second, coordinate the drivers for change inside and outside of the organization. External groups — whether academic bodies or industry research organizations — are a valuable resource for best practices and guidelines, but they need to be adapted and implemented in ways that make sense for each organization and address the issues it is most likely to encounter. Governance guidelines should clearly address why they are in place and leave little room for interpretation, which can lead to the slippery slope of “exceptions to the rule.” This means ensuring that teams across different functions — such as leadership, data science, and legal — understand the imperatives and incentives of each.
Finally, expand the notion of measurable value to evolve from a short-term to longer-term mindset while also appreciating the importance of the immeasurable. If organizations are truly to build value-driven technology, their organizational structures will have to evolve to meet these lofty aspirations.