The Data Problem Stalling AI
AI efforts can fail to move out of the lab if organizations don’t carefully manage access to data throughout the development and production life cycle.
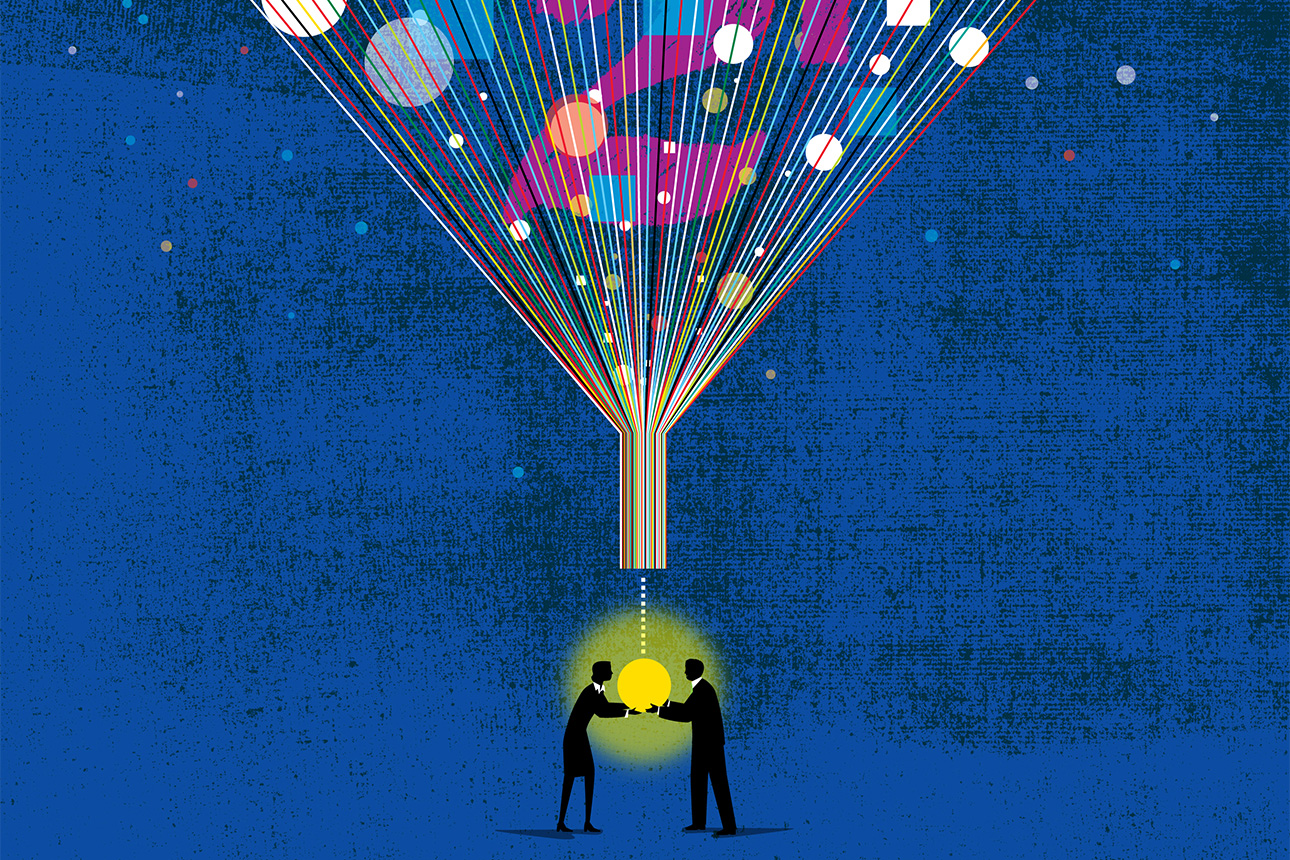
Image courtesy of Michael Austin/theispot.com
A large North American hospital was excited about the potential of an AI-enabled system that would improve patient care. As it was planning to move from concept to building a prototype, it discovered that the data required to build and operate the system was scattered across 20 legacy systems and retrieving that data would be too complex. The project had to be scrapped.
Advanced analytics and artificial intelligence promise to generate insights that will help organizations stay competitive. Their ability to do that is heavily dependent on the availability of good data, but sometimes organizations just don’t have the data to make AI work.
Get Updates on Leading With AI and Data
Get monthly insights on how artificial intelligence impacts your organization and what it means for your company and customers.
Please enter a valid email address
Thank you for signing up
We recently studied how organizations move their AI initiatives from R&D, lablike settings into production and the problems they encounter in doing so. The research is based on interviews with key AI leaders and informants in six North American companies of different sizes and operating in different industries. A key finding is that, although many people focus on the accuracy and completeness of data to determine its quality (see “What Is Good Data?”), the degree to which it is accessible by machines — one of the dimensions of data quality — appears to be a bigger challenge in taking AI out of the lab and into the business. More important, we found that data accessibility is too often treated exclusively as an IT problem.
References
1. R. Kimball and M. Ross, “The Data Warehouse Toolkit: The Definitive Guide to Dimensional Modeling,” 3rd ed. (Indianapolis: John Wiley & Sons, 2013).
2. R. Hughes, “Agile Data Warehousing Project Management: Business Intelligence Systems Using Scrum” (Waltham, Massachusetts: Morgan Kaufmann, 2013); and K. Collier, “Agile Analytics: A Value-Driven Approach to Business Intelligence and Data Warehousing” (Boston: Addison-Wesley, 2011).
i. R.Y. Wang and D.M. Strong, “Beyond Accuracy: What Data Quality Means to Data Consumers,” Journal of Management Information Systems 12, no. 4 (spring 1996): 5-33; L.L. Pipino, Y.W. Lee, and R.Y. Wang, “Data Quality Assessment,” Communications of the ACM 45, no. 4 (April 2002): 211-218; and B. Baesens, R. Bapna, J.R. Marsden, et al., “Transformational Issues of Big Data and Analytics in Networked Business,” MIS Quarterly 40, no. 4 (December 2016): 807-818.