Using Federated Machine Learning to Overcome the AI Scale Disadvantage
A promising new approach to training AI models lets companies with small data sets collaborate while safeguarding proprietary information.
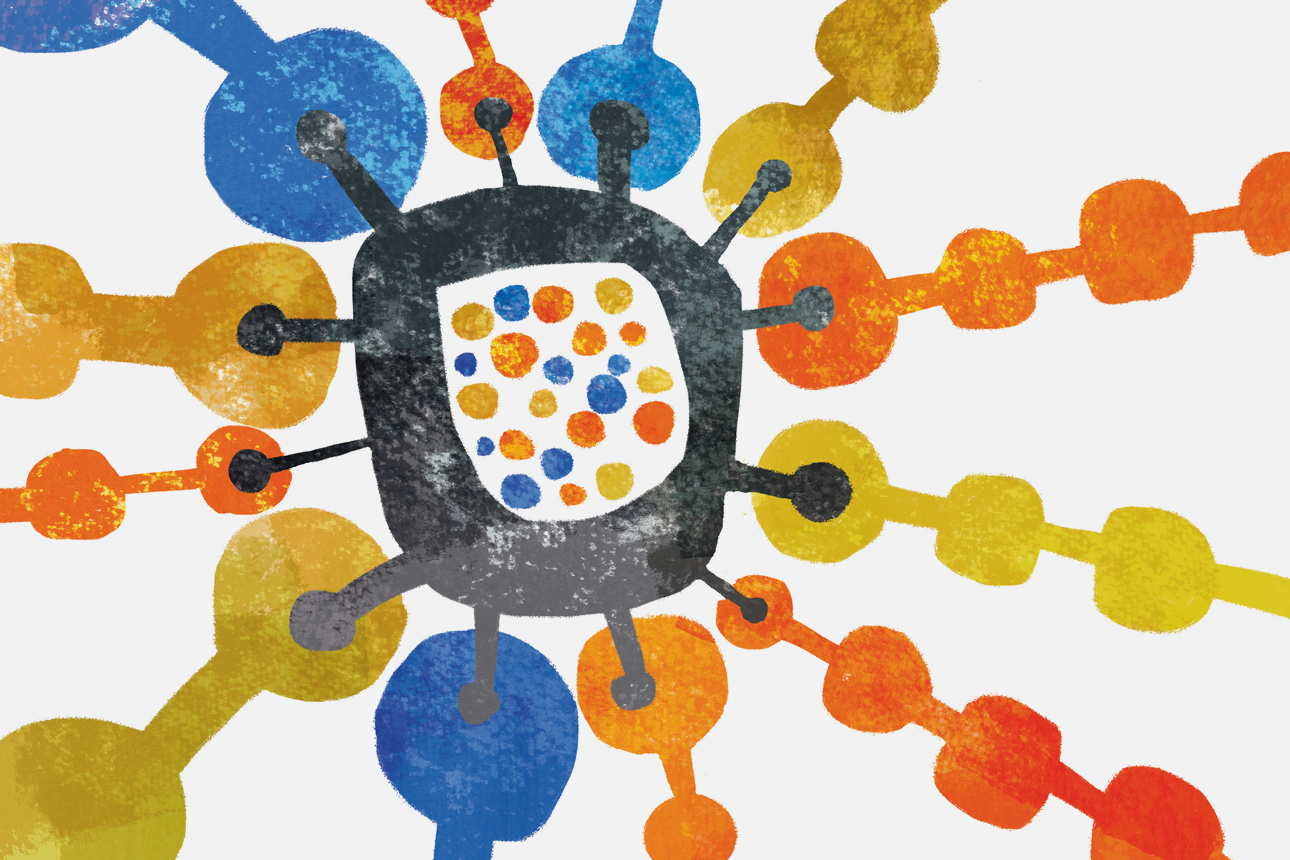
Jing Jing Tsong/theispot.com
Deep pockets, access to talent, and massive investments in computing infrastructure only partly explain why most major breakthroughs in artificial intelligence have come from a select group of Big Tech companies that includes Amazon, Google, and Microsoft. What sets the tech giants apart from the many other businesses seeking to gain an edge from AI are the vast amounts of data they collect as platform operators. Amazon alone processes millions of transactions each month on its platform. All of that big data is a rich strategic resource that can be used to develop and train complex machine learning algorithms — but it’s a resource that is out of reach for most enterprises.
Access to big data allows for more sophisticated and better-performing AI and machine learning models, but many companies must make do with much smaller data sets. For smaller companies and those operating in traditional sectors like health care, manufacturing, or construction, a lack of data is the biggest impediment to venturing into AI. The digital divide between big and small-data organizations is a serious concern due to self-reinforcing data network effects, where more data leads to better AI tools, which help attract more customers who generate more data, and so forth.1 This gives bigger companies a strong competitive AI advantage, with small and midsize organizations struggling to keep up.
Get Updates on Leading With AI and Data
Get monthly insights on how artificial intelligence impacts your organization and what it means for your company and customers.
Please enter a valid email address
Thank you for signing up
The idea of multiple small-scale companies pooling their data in a jointly controlled central repository has been around for a while, but concerns about data privacy may quash such initiatives.2 Federated machine learning (FedML) is a recent innovative technology that overcomes this problem by means of privacy-preserving collaborative AI that uses decentralized data. FedML might turn out to be a game changer in addressing the digital divide between companies with and without big data and enabling a larger part of the economy to reap the benefits of AI. It’s a technology that doesn’t just sound promising in theory — it has already been successfully implemented in industry, as we’ll detail below. But first, we’ll explain how it works.
Small Data and Federated Machine Learning
FedML is an approach that allows small-data organizations to train and use sophisticated machine learning models. The definition of small data depends on the complexity of the problem being addressed by AI.
References
1. S.S. Levine and D. Jain, “How Network Effects Make AI Smarter,” Harvard Business Review, March 14, 2023, https://hbr.org.
2. Y. Bammens and P. Hünermund, “How Midsize Companies Can Compete in AI,” Harvard Business Review, Sept. 6, 2021, https://hbr.org.
3. R. Ramakrishnan, “How to Build Good AI Solutions When Data Is Scarce,” MIT Sloan Management Review 64, no. 2 (winter 2023): 48-53.
4. H. Ceulemans, “Melloddy: A Bold Idea Implemented,” July 28, 2020, Melloddy (blog), www.melloddy.eu.
5. M. Galtier, “Melloddy: A ‘Co-Opetitive’ Platform for Machine Learning Across Companies Powered by Owkin Technology,” Feb. 17, 2020, Melloddy (blog), www.melloddy.eu.
6. Y. Bammens and J. Lilienweiss, “How Tech Startups Protect Against the Downside of Corporate Venture Capital,” Entrepreneur & Innovation Exchange, Dec. 2, 2022, https://eiexchange.com.
7. A. Agrawal, J. Gans, and A. Goldfarb, “A Simple Tool to Start Making Decisions With the Help of AI,” Harvard Business Review, April 17, 2018, https://hbr.org.
8. H.B. McMahan, E. Moore, D. Ramage, et al., “Communication-Efficient Learning of Deep Networks From Decentralized Data,” Proceedings of the 20th International Conference on Artificial Intelligence and Statistics 54 (April 2017): 1273-1282.