What Is a Minimum Viable AI Product?
To be successful, an AI pilot needs to go beyond the basic requirements of standard IT projects.
Topics
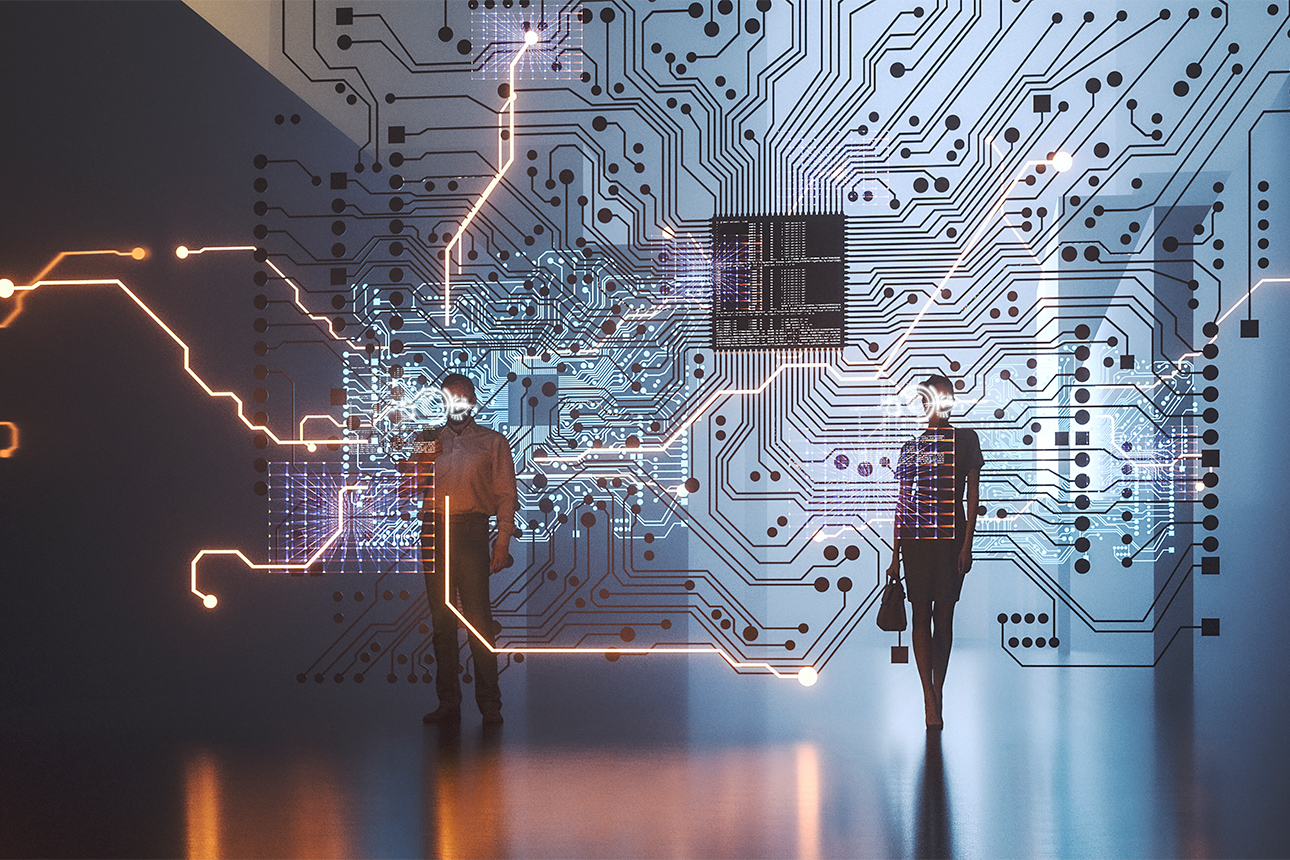
One of the key attributes of the lean startup approach popularized by Steve Blank and Eric Ries is the development and refinement of a minimum viable product (MVP) that engages customer and investor attention without large product development expenditures. Initially defined by technologist Frank Robinson, an MVP may not meet all customer needs, but it offers enough capabilities for highly interested customers to get started. It’s a paradigm that has become well established in technology product development.
But what does the concept of an MVP mean for artificial intelligence? This is a question that is relevant not only to startups but also to large enterprises. Many companies are developing AI pilots in different areas of their business that they hope will demonstrate the potential value of AI and eventually lead to production deployment. An MVP for a large organization has many similarities to a pilot or proof of concept.
Get Updates on Leading With AI and Data
Get monthly insights on how artificial intelligence impacts your organization and what it means for your company and customers.
Please enter a valid email address
Thank you for signing up
For any organization pursuing AI, then, it’s important to understand what constitutes a successful MVP. It’s equally important to a venture capital firm that invests primarily in AI companies — like Glasswing Ventures, with which we are both involved — to understand AI MVPs and what it takes to improve them. Based on several Glasswing portfolio companies and others we’ve researched, we’d argue that while some of the necessary attributes are true of IT products in general — that it’s useful even in its earliest stages, that customers’ early use can be monitored in order to improve the product, and that it can be developed relatively quickly and cheaply — early AI products have some unique requirements in terms of what qualifies them for MVP status.
Data and the MVP
Machine learning is a common underlying technology for AI, and it improves via copious amounts of data. Supervised learning, by far the most common type in business, requires data with labeled outcomes. Therefore, data is perhaps the single most critical resource for an AI product, and it is necessary even at the MVP stage. With no data, there is no trained machine learning algorithm.
Comment (1)
Jo koorapati