What’s Holding Your Data Program Back?
To deliver on the promise of data-backed technology, such as AI, companies must address underlying restraining forces.
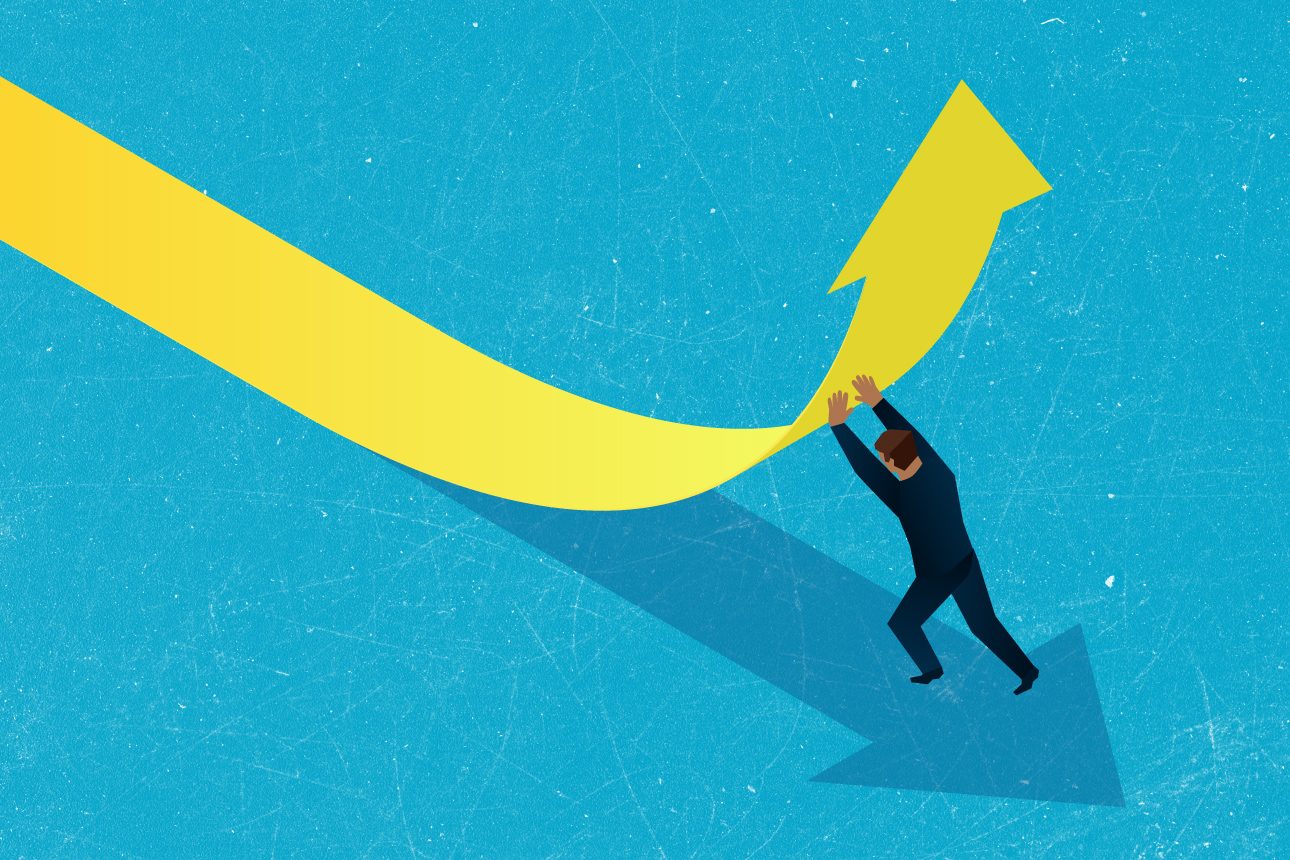
In my career, I’ve spent more than 25 years helping companies with their data and data quality programs. Beginning in 2018, I undertook a broad-based research effort to understand why, so many years into the digital revolution, progress in the data space is so slow. This article synthesizes a review of my clients’ and others’ successes and failures, discussions with dozens of experts in data and analytics, and study groups that dived deeply into various aspects of the topic.
The headline result: Today’s organizations are unfit for data. Until companies address the underlying issues, progress will remain halting and uncertain.
Get Updates on Leading With AI and Data
Get monthly insights on how artificial intelligence impacts your organization and what it means for your company and customers.
Please enter a valid email address
Thank you for signing up
To be clear, the many successes I’ve observed — in data science, analytics, artificial intelligence, data quality, and other ways to put data to work — confirm that the approaches, methods, and technologies around data work just fine. But most companies still struggle. Organizational structures, people issues, a lack of accountability, and other traps get in the way. This conclusion has far-reaching consequences, and leaders should not expect faster progress until they make some real changes. To do so, they must first understand and address the underlying issues that hinder progress.
To better understand both driving forces (those pushing progress forward) and restraining forces (those holding back progress) that are impacting data science progress, I’ve used force field analysis (FFA), a powerful visual tool derived from Kurt Lewin’s change management model. A companion toolkit provides information on how to build out your own FFA. (See “Visualizing Change With Force Field Analysis.”)
Five Areas Key to Success
“Data” is a very broad space, so for this analysis, we will look at five areas of critical importance to companies: data quality, putting data to work, organizational capability, technology, and defense. Failure to deliver in any of these areas can scuttle an otherwise terrific data program:
- Data quality: Poor-quality data adds incredible cost and friction.
- Putting data to work: Unless companies put data to work in ways that return value, there is little business benefit. Ways to do so include data science (including AI and machine learning), exploiting proprietary data, creating a data-driven culture, monetizing data by selling it or building it into products and services, and treating data as an asset.
- Organizational capability: This refers to the people, structure, and culture within the organization that support data programs.
Comments (5)
Heather Hill
Saradhi Motamarri
Riona Rooplal
Fong SM
Francisco Araujo