AI Ethics at Unilever: From Policy to Process
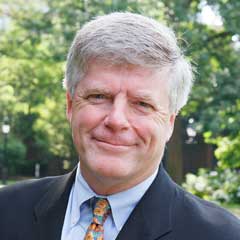
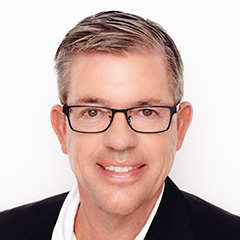
Topics
AI in Action
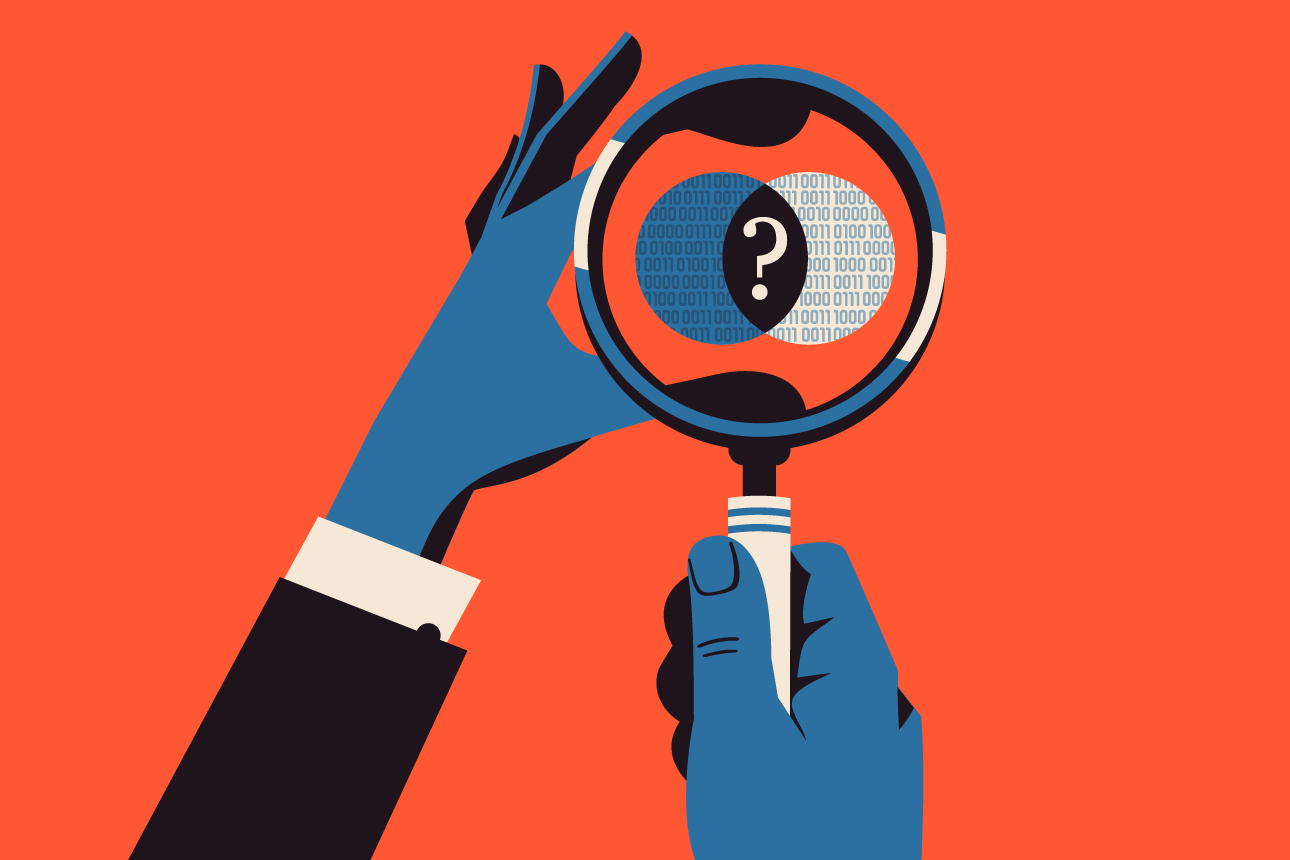
Carolyn Geason-Beissel/MIT SMR | Getty Images
Many large companies today — most surveys suggest over 70% globally — have determined that artificial intelligence is important to their future and are building AI applications in various parts of their businesses. Most also realize that AI has an ethical dimension and that they need to ensure that the AI systems they build or implement are transparent, unbiased, and fair.
Thus far, many companies pursuing ethical AI are still in the early stages of addressing it. They might have exhorted their employees to take an ethical approach to AI development and use or drafted a preliminary set of AI governance policies. Most have not done even that; in one recent survey, 73% of U.S. senior leaders said they believe that ethical AI guidelines are important, yet only 6% had developed them.
Get Updates on Leading With AI and Data
Get monthly insights on how artificial intelligence impacts your organization and what it means for your company and customers.
Please enter a valid email address
Thank you for signing up
We see five stages in the AI ethics process: evangelism, when representatives of the company speak about the importance of AI ethics; development of policies, where the company deliberates on and then approves corporate policies around ethical approaches to AI; recording, where the company collects data on each AI use case or application (using approaches such as model cards); review, where the company performs a systematic analysis of each use case (or outsources it to a partner company) to determine whether the case meets the company’s criteria for AI ethics; and action, where the company either accepts the use case as it is, sends it back to the proposing owner for revision, or rejects it.
It is only in the higher-level stages — review and action — that a company can actually determine whether its AI applications meet the transparency, bias, and fairness standards that it has established. For it to put those stages in place, it has to have a substantial number of AI projects, processes, and systems for gathering information, along with governance structures for making decisions about specific applications. Many companies do not yet have those preconditions in place, but they will be necessary as companies exhibit greater AI maturity and emphasis.
Early Policies at Unilever
Unilever, the British consumer packaged goods company whose brands include Dove, Seventh Generation, and Ben & Jerry’s, has long had a focus on corporate social responsibility and environmental sustainability. More recently, the company has embraced AI as a means of dramatically improving operations and decision-making across its global footprint. Unilever’s Enterprise Data Executive, a governance committee, recognized that the company could build on its robust privacy, security, and governance controls by embedding the responsible and ethical use of AI into the company’s data strategies. The goal was to take advantage of AI-driven digital innovation to both maximize the company’s capabilities and promote a fairer and more equitable society. A multifunctional team was created and tasked with exploring what this meant in practice and building an action program to operationalize the objective.
Unilever has now implemented all of the five stages described above, but, looking back, its first step was to create a set of policies. One policy, for example, specified that any decision that would have a significant life impact on an individual should not be fully automated and should instead ultimately be made by a human. Other AI-specific principles that were adopted include the edicts “We will never blame the system; there must be a Unilever owner accountable” and “We will use our best efforts to systematically monitor models and the performance of our AI to ensure that it maintains its efficacy.”
Committee members realized quickly that creating broad policies alone would not be sufficient to ensure the responsible development of AI. To build confidence in the adoption of AI and truly unlock its full potential, they needed to develop a strong ecosystem of tools, services, and people resources to ensure that AI systems would work as they were supposed to.
One Unilever policy states that any decision that has a significant life impact on an individual should not be fully automated.
Committee members also knew that many of the AI and analytics systems at Unilever were being developed in collaboration with outside software and services vendors. The company’s advertising agencies, for example, often employed programmatic buying software that used AI to decide what digital ads to place on web and mobile sites. The team concluded that its approach to AI ethics needed to include attention to externally sourced capabilities.
Developing a Robust AI Assurance Process
Early on in Unilever’s use of AI, the company’s data and AI leaders noticed that some of the issues with the technology didn’t involve ethics at all — they involved systems that were ineffective at the tasks they were intended to accomplish. Giles Pavey, Unilever’s global director of data science, who had primary responsibility for AI ethics, knew that this was an important component of an AI use case. “A system for forecasting cash flow, for example, might involve no fairness or bias risk but may have some risk of not being effective,” he said. “We decided that efficacy risk should be included along with the ethical risks we evaluate.” The company began to use the term AI assurance to broadly encompass its overview of a tool’s effectiveness and ethics.
The basic idea behind the Unilever AI assurance compliance process is to examine each new AI application to determine how intrinsically risky it is, both in terms of effectiveness and ethics. The company already had a well-defined approach to information security and data privacy, and the goal was to employ a similar approach that would ensure that no AI application was put into production without first being reviewed and approved. Integrating the compliance process into the compliance areas that Unilever already had in place, such as privacy risk assessment, information security, and procurement policies, would be the ultimate sign of success.
Debbie Cartledge, who took on the role of data and AI ethics strategy lead for the company, explained the process the team adopted:
When a new AI solution is being planned, the Unilever employee or supplier proposes the outlined use case and method before developing it. This is reviewed internally, with more complex cases being manually assessed by external experts. The proposer is then informed of potential ethical and efficacy risks and mitigations to be considered. After the AI application has been developed, Unilever, or the external party, runs statistical tests to ascertain whether there is a bias or fairness issue and could examine the system for efficacy in achieving its objectives. Over time, we expect that a majority of cases can be fully assessed automatically based on information about the project supplied by the project proposer.
Depending on where within the company the system will be employed, there also might be local regulations for the system to comply with. All resume checking, for example, is now done by human reviewers. If resume checking were fully automated, the review might conclude that the system needs a human in the loop to make final decisions about whether to move a candidate to interview. If there are serious risks that can’t be mitigated, the AI assurance process will reject the application on the grounds that Unilever’s values prohibit it. Final decisions on AI use cases are made by a senior executive board, including representatives from the legal, HR, and data and technology departments.
Here’s an example: The company has areas in department stores where it sells its cosmetics brands. A project was developed to use computer vision AI to automatically register sales agents’ attendance through daily selfies, with a stretch objective to look at the appropriateness of agents’ appearance. Because of the AI assurance process, the project team broadened their thinking beyond regulations, legality, and efficacy to also consider the potential implications of a fully automated system. They identified the need for human oversight in checking photos flagged as noncompliant and taking responsibility for any consequent actions.
Working With an Outside Partner, Holistic AI
Unilever’s external partner in the AI assurance process is Holistic AI, a London-based company. Founders Emre Kazim and Adriano Koshiyama have both worked with Unilever AI teams since 2020, and Holistic AI became a formal partner for AI risk assessment in 2021.
Holistic AI has created a platform to manage the process of reviewing AI assurance. In this context, “AI” is a broad category that encompasses any type of prediction or automation; even an Excel spreadsheet used to score HR candidates would be included in the process. Unilever’s data ethics team uses the platform to review the status of AI projects and can see which new use cases have been submitted; whether the information is complete; and what risk-level assessment they have received, coded red, yellow (termed “amber” in the U.K.), or green.
The traffic-light status is assessed at three points: at triage, after further analysis, and after final mitigation and assurance. At this final point, the ratings have the following interpretations: A red rating means the AI system does not comply with Unilever standards and should not be deployed; yellow means the AI system has some acceptable risks and the business owner is responsible for being aware of and taking ownership of it; and green means the AI system adds no risks to the process. Only a handful of the several hundred Unilever use cases have received red ratings thus far, including the cosmetics one described above. All of the submitters were able to resolve the issues with their use cases and move them up to a yellow rating.
For leaders of AI projects, the platform is the place to start the review process. They submit a proposed use case with details, including its purpose, the business case, the project’s ownership within Unilever, team composition, the data used, the type of AI technology employed, whether it is being developed internally or by an external vendor, the degree of autonomy, and so forth. The platform uses the information to score the application in terms of its potential risk. The risk domains include explainability, robustness, efficacy, bias, and privacy. Machine learning algorithms are automatically analyzed to determine whether they are biased against any particular group.
For leaders of AI projects, the platform is the place to start the review process.
An increasing percentage of the evaluations in the Holistic AI platform are based on the European Union’s proposed AI Act, which also ranks AI use cases into three categories of risk (unacceptable, high, and not high enough to be regulated). The act is being negotiated among EU countries with hopes for an agreement by the end of 2023. Kazim and Koshiyama said that even though the act will apply only to European businesses, Unilever and other companies are likely to adopt it globally, as they have with the EU’s General Data Protection Regulation.
Kazim and Koshiyama expect Holistic AI to be able to aggregate data across companies and benchmark across them in the future. The software could assess benefits versus costs, the efficacy of different external providers of the same use case, and the most effective approaches to AI procurement. Kazim and Koshiyama have also considered making risk ratings public in some cases and partnering with an insurance company to insure AI use cases against certain types of risks.
We’re still in the early stages of ensuring that companies take ethical approaches to AI, but that doesn’t mean that it’s enough to issue pronouncements and policies with no teeth. Whether AI is ethical or not will be determined use case by use case. Unilever’s AI assurance process, and its partnership with Holistic AI to evaluate each use case about its ethical risk level, is the only current way to ensure that AI systems are aligned with human interests and well-being.