How Marketers Can Address Data Challenges to Drive Growth
A research-based tool offers marketers methods for designing an analytics architecture that fits the business need.
Topics
Connecting With Customers in the Age of Acceleration
Brought to you by
Brightcove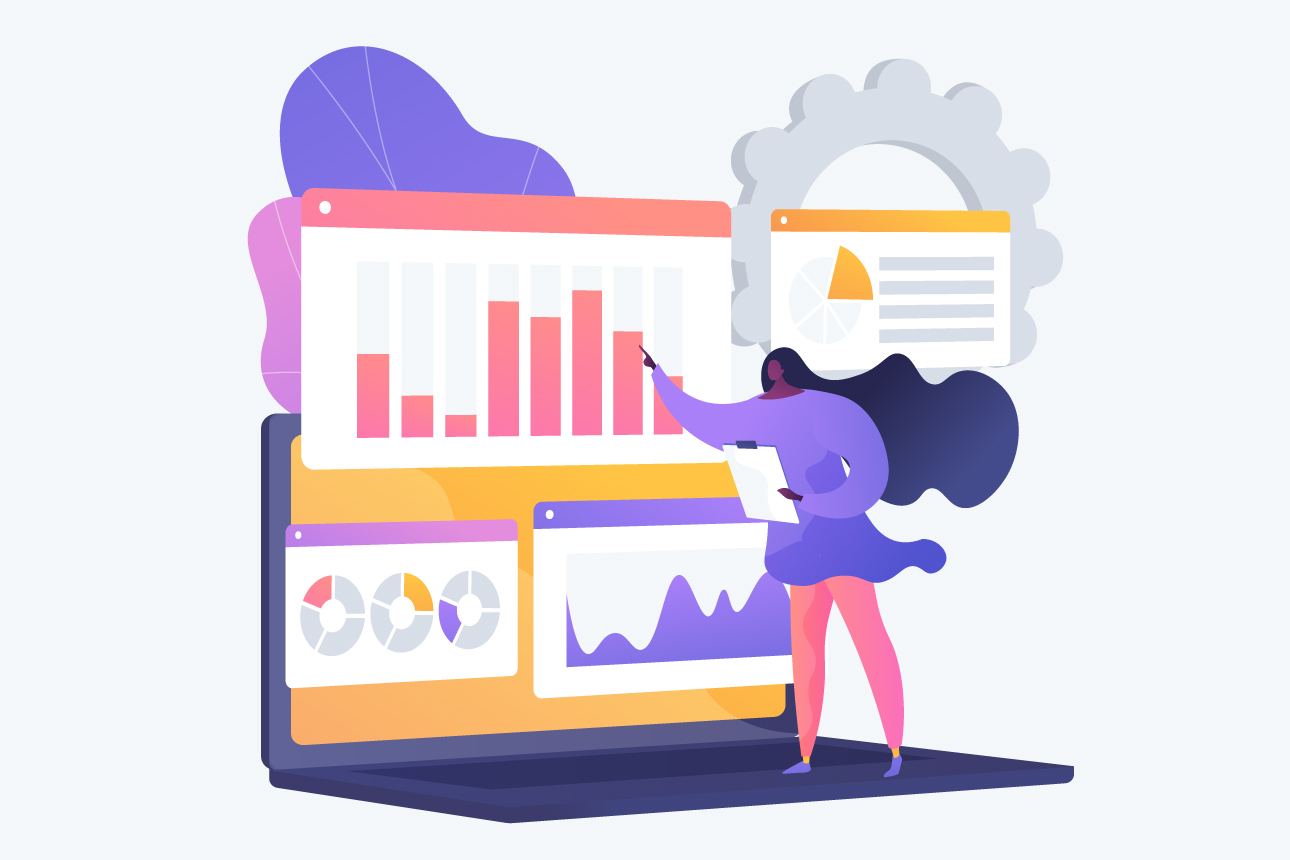
Imagine that you have just been promoted to manager at a large food company and will be in charge of a popular candy brand primarily sold in grocery stores. The brand has been declining for some time, and you have to figure out the source of the decline and fix it. What do you do? If you’re like most marketers, you turn to readily available reports and market studies to find the answer. But is this the best approach?
Today’s marketers are more responsible than ever for leveraging their company’s data acquisition, integration, and analytical efforts to shape a better customer experience (CX). In fact, a recent McKinsey study found that 83% of global CEOs expect marketers to be a major driver for most or all of their company’s growth agenda. Despite marketing leaders’ increasingly important role in building analytical capability, many still struggle to effectively move beyond the conceptual goal of “delivering analytical value” to actually use analytics to drive growth. In the same McKinsey study, 23% of CEOs indicated they don’t believe their marketers are able to deliver on the growth agenda.
Get Updates on Transformative Leadership
Evidence-based resources that can help you lead your team more effectively, delivered to your inbox monthly.
Please enter a valid email address
Thank you for signing up
In our research into this gap between marketing analytics availability and capability, we have interacted with over 200 senior executives across the world in seminars, onsite visits, observations, consulting engagements, and interviews. We also partnered with analytics software provider Teradata to collect survey data from 300 executives. An important source of variance in marketing leaders’ ability to deliver analytical value traces back to where they are getting their data. We found there is a tendency to rely on existing data and reports rather than start with a clean slate to map out what is needed. In many cases, existing reports are simply insufficient or ineffective at addressing CX issues.
Our interviews and experience with successful CMOs suggest that in order to address this problem, marketers need to understand the strategic business challenge first, identify the required data needed, and then design the appropriate analytical solution. In some cases, this may mean acquiring new data, accessing new data within the company, or bringing in analytical skills missing in the current team. Importantly, this approach increases the likelihood that marketers will use better data sources and analytical methods that map directly onto their business challenges.
The Marketing Analytics Canvas Tool
To help marketers address such challenges, we created a tool that guides the process of designing an analytics architecture: the Marketing Analytics Canvas (MAC). The objective is to provide a road map that helps marketers approach customer experience challenges where analytics plays a key role. We relied on marketers’ familiarity with marketing technology blueprints and customer journey maps to create a tool that would enable marketers to go beyond existing data and reports to think through how to solve challenging CX issues.
The MAC provides a structured framework to elaborate the analytics challenge, the options that can be tested to address it, the business or financial consequences expected from implementing the options, and the data and analytical methods for measuring and estimating the connections between the strategic options and business and financial consequences. (See “The Marketing Analytics Canvas.”)
This tool helps address marketers’ analytics challenges in two ways. First, by anchoring the solution on the core strategic business challenge, this approach increases the likelihood that the data and analytics will help solve it. Second, it opens marketers’ minds to letting the hypotheses drive the identification of data needed and analytical method rather than what is readily available or has been used in the past. This increases the likelihood that the data and analytics being used are the most appropriate ones for the business challenge.
Compare.com: A Case Study
To provide insights on how to use the MAC, we offer the case study of Compare.com, a comparison shopping website that gives customers a list of real-time available quotes from affiliated automotive insurance providers. Its offering is unique in the U.S. market because customers receive actual price quotes (in contrast to the estimates provided by other companies) after completing a questionnaire.
A significant strategic challenge Compare.com faced in 2016 was low and declining completion rates on its questionnaires — 12% in March 2016, after a peak of 18% in January 2016 (a 33% decline in three months). Improving completion rates was a critical goal, given that Compare.com gets paid only when a customer fills out a questionnaire and accepts a quote provided by an insurance partner on the platform.
To begin development of its MAC, Compare debated three options for solving the questionnaire completion rate challenge: (1) create a mobile app, (2) obtain emails from customers so that they could fill out the questionnaire later, and (3) provide a banner ad that predicted the insurance premium a customer would be offered. The company selected the “predict premium” option for initial testing based on the speed with which it could test it, estimates that indicated it would likely yield a more immediate impact, and limitations associated with the other two options. For example, Compare worried that consumers would not download a mobile app and that connecting via email might not work because of spam filters.
As shown in “The Compare.com Marketing Analytics Canvas,” the rationale for choosing the predictive banner ad was that customers would be motivated to finish the questionnaire if they saw an estimate of the savings they could obtain from Compare.com. Improving questionnaire completion rates would directly translate into higher rates of customers clicking through and purchasing one of the policies presented to them, subsequently increasing the total revenue Compare.com would obtain from insurance providers. The MAC also included other processes the company was already using to improve questionnaire completion rates, including providing questionnaires on a single page, asking for vehicle information first and personal information later, pre-filling forms for customers coming from broker websites, and asking customers for email addresses so that the questionnaire could be emailed to them. The premium-prediction initiative needed to improve completion rates beyond these status quo actions. The MAC helped the team formalize the problem, design the solution, and test the outcomes. If the MAC hadn’t been used, Compare.com would have likely directed its efforts toward redesigning the user experience of the questionnaire instead of exploring the premium-prediction option.
The projected results from implementing the prediction banner was a 15% improvement in questionnaire completion rates and a corresponding increase in revenue from insurance providers.
Many marketing teams fail to structure the business challenge in a way that helps to identify the appropriate data and analytical methods necessary to address opportunities and challenges.
In completing the MAC for this particular business challenge, the Compare.com team detailed the data necessary to conduct the tests, sources of such data, and the analytical methods required. Importantly, these are the final steps of filling out the canvas, to ensure that the right data and analytical methods are identified. Website visitors from three states were assigned to the test group to receive the predicted premiums as a banner advertisement as soon as they landed on Compare.com. Visitors from the rest of the states were in the control group. The test showed an improvement of 4% in questionnaire completion rates and a 6% improvement in consumers choosing to purchase insurance from one of the providers. A follow-up test showed a 22% improvement in completion rates among consumers who chose to receive a predicted premium after answering five questions before completing the rest of the questionnaire. The results from these experiments motivated Compare.com to explore personalized nudges for improving completion rates based on the preferences of different consumer segments. Using the MAC allowed the team to adopt new methods to improve the CX of the website and develop new analytics methods for solving the company’s strategic challenges.
Despite the fact that most marketing leaders would like to leverage data and analytics more effectively, many continue to struggle. It is not for lack of interest or will, but rather because many marketing teams fail to structure the business challenge in a way that helps to identify the appropriate data and analytical methods necessary to address opportunities and challenges.
Consider the example from the introduction. Many managers in charge of a popular candy brand facing a business decline would immediately turn to readily available data and previously tested methods. The issue is that these reports and efforts might miss macroeconomic effects. They might not capture a shift in consumer habits and practices. Or they might ignore significant, recent competitive changes. The Marketing Analytics Canvas tool enables marketing leaders to step back and craft an approach that is driven by the business challenge so that they can then acquire the right data and employ a methodology to address the problem more effectively. By following an easy-to-use framework, marketers can avoid putting the proverbial cart before the horse.