How Scotiabank Built an Ethical, Engaged AI Culture
This Canadian bank has become known for developing AI tools that are both innovative and responsible.
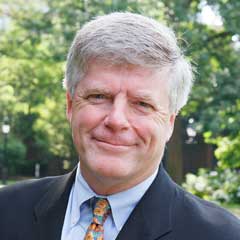
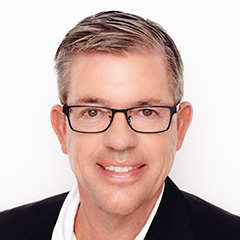
Topics
AI in Action

Carolyn Geason-Beissel/MIT SMR | Getty Images
What does it take to produce an award-winning AI initiative? Scotiabank — officially the Bank of Nova Scotia, and one of Canada’s largest banks — recently won two awards for AI at one event. DataIQ gave the bank a Most Innovative Use of AI award for its chatbot and recognized its overall data and AI ethics program as the Best Responsible AI Program, calling it “a pioneering initiative in the financial industry.”
We think it would be helpful to describe how an AI use case that’s both innovative and responsible came about, and how a particular AI use case reflects the culture in which it’s developed. We last wrote about Scotiabank in 2021, but a lot has changed since then — and not just the advent of generative AI.
How to Make a Successful Chatbot
The application that won the DataIQ innovation award supports a chatbot for Scotiabank’s contact center. You may know that AI-based chatbots are common among large banks. What makes one chatbot better than another is the quality of the underlying knowledge embedded in it, and the quality of the AI models that serve it up to customers. Scotiabank is addressing both issues.
Grace Lee, the bank’s chief data and analytics officer, said she is particularly proud of the contact center’s participative effort to improve knowledge quality. The center took ownership of its knowledge base and curated it effectively to ensure that each document fed into the chatbot was clear, unique, and up to date. The award acknowledged the tool’s creative application of auxiliary AI models that enhance and maintain the chatbot’s training. This AI-for-AI strategy has enabled Lee’s team to automate significant portions of the bot training process, such as identifying optimal new training topics, saving thousands of hours in manual labor. With these efficiencies has come a better product: Since its introduction in late 2022, the chatbot’s accuracy level has increased from 35% accurate to 90%.
Each document fed into the chatbot was clear, unique, and up to date.
More than 40% of customer questions via chat are answered without human intervention. When customers decide they need to speak to a human agent, they expect the agent to be familiar with what they have discussed with the chatbot. To provide this capability, the bank has developed a rapid summarization capability using large language models. The agent is provided with a short text summary of the conversation, including the customer’s intent and requested action. Having the summary available reduces the overall time required for the agent to come up to speed by 60% to 70%.
Contact center staff weren’t the only ones who worked on the chatbot. Other groups included Lee’s customer insights, data, and analytics organization; digital product and design; and software engineering. Lee told us that the participative and collaborative development of the chatbot is indicative of a cultural change at the bank.
As generative AI tools like ChatGPT have made AI more accessible, people are sufficiently excited and engaged about what AI can now do that they are more willing to undertake improvements in the unstructured data used to feed it. This is consistent with the findings of our most recent survey of large organizations’ data environments about the state of data and AI at leading companies in 2024: For the first time ever, the number of respondents saying their organizations had established a data and analytics culture doubled (from 21% to 43%) in a year. We concluded that generative AI was the most likely cause, and Lee’s comments seem to support that hypothesis.
A New Data Domain
When we wrote about Scotiabank three years ago, the data management focus was on creating reusable authoritative data sets, or RADs. As the bank has moved its data to the cloud, the focus is now on consolidating RADs in a cloud-hosted enterprise data model for the entire bank to access: This creates one version of the truth and simplifies management, governance, and use of data. Structured data of this type will always be important for banks and the majority of other organizations.
What is new at Scotiabank, though, is the attempt to manage unstructured data, as the contact center did with its customer questions and answers. This type of data is the fuel of generative AI, but most organizations haven’t really begun to manage it effectively. In our survey of data leaders at the end of 2023, 93% agreed that a new data strategy was critical for success with generative AI, but 57% had taken no steps toward a new approach.
But Scotiabank’s Lee is taking action. She said, “[despite the fact that] knowledge management is a perennial and daunting exercise, with many documents and policies related to a large number of products and services across multiple geographies,” she views the management of knowledge, information, and documents as part of her data remit. “We will no doubt discover lots of duplication and challenges with our knowledge base,” she said, citing the contact center’s discovery of multiple versions of the same bank policy, often in paper printouts.
The bank has begun to address the issue with a variety of initiatives. Since there is such a groundswell of interest in generative AI experiments, and a close connection between knowledge of the business and knowledge in each part of it, Lee said she expects that the business side of the bank will increasingly take ownership over unstructured data quality. In addition to the contact center, Scotiabank has also cleaned up the knowledge base for the payments business. It was a narrower product suite than the contact center needed to address, and Lee said that her group managed to get that group’s business team up to speed quickly on how to curate their own content. She expects her group to be doing that progressively in other parts of the bank.
Ethics Built In
As we discussed in a previous column on Unilever, we’ve been arguing that organizations need to embed ethics-oriented thinking into the process of building AI solutions from an early stage. Scotiabank is taking that approach not only for generative AI but for all types of AI and analytics, and the use of data in the bank more broadly. On top of having an AI risk management policy, Scotiabank has a data ethics policy and a data ethics team to advance it. The policy is now part of the bank’s code of conduct, to which all employees must attest their acceptance each year. The data ethics approach also won an award for that work at the Qorus-Accenture Banking Innovation Awards (OK, a bronze medal, but that was out of more than 680 banking entries).
Organizations need to embed ethics-oriented thinking into the process of building AI solutions from an early stage.
To identify AI ethics issues at an early stage of use case development, Scotiabank worked with Deloitte Canada to develop Ethics Assistant, an application that evaluates the ethical impact of an AI use case before it is fully deployed. Running the assistant is the first step for all new AI and machine learning projects at the bank. If it uncovers ethics issues, the use case is at an early enough stage to change the design.
In addition, the bank developed a mandatory data ethics education program for anyone in the customer insights, data, and analytics organization or doing advanced analytics work elsewhere in the bank. All of these ethical foci together were distinctive enough for Scotiabank to stand out from other financial organizations and win DataIQ’s responsible AI award.
When we described Scotiabank in our 2021 column, we described a bank in catch-up mode relative to its competitors. Now it appears to be in the lead in many respects. The breadth of activities and participation in AI throughout the organization augurs well for its future.