Predictive Models Can Lose the Plot. Here’s How to Keep Them on Track.
Algorithmic inertia can result in guidance that leads businesses astray.
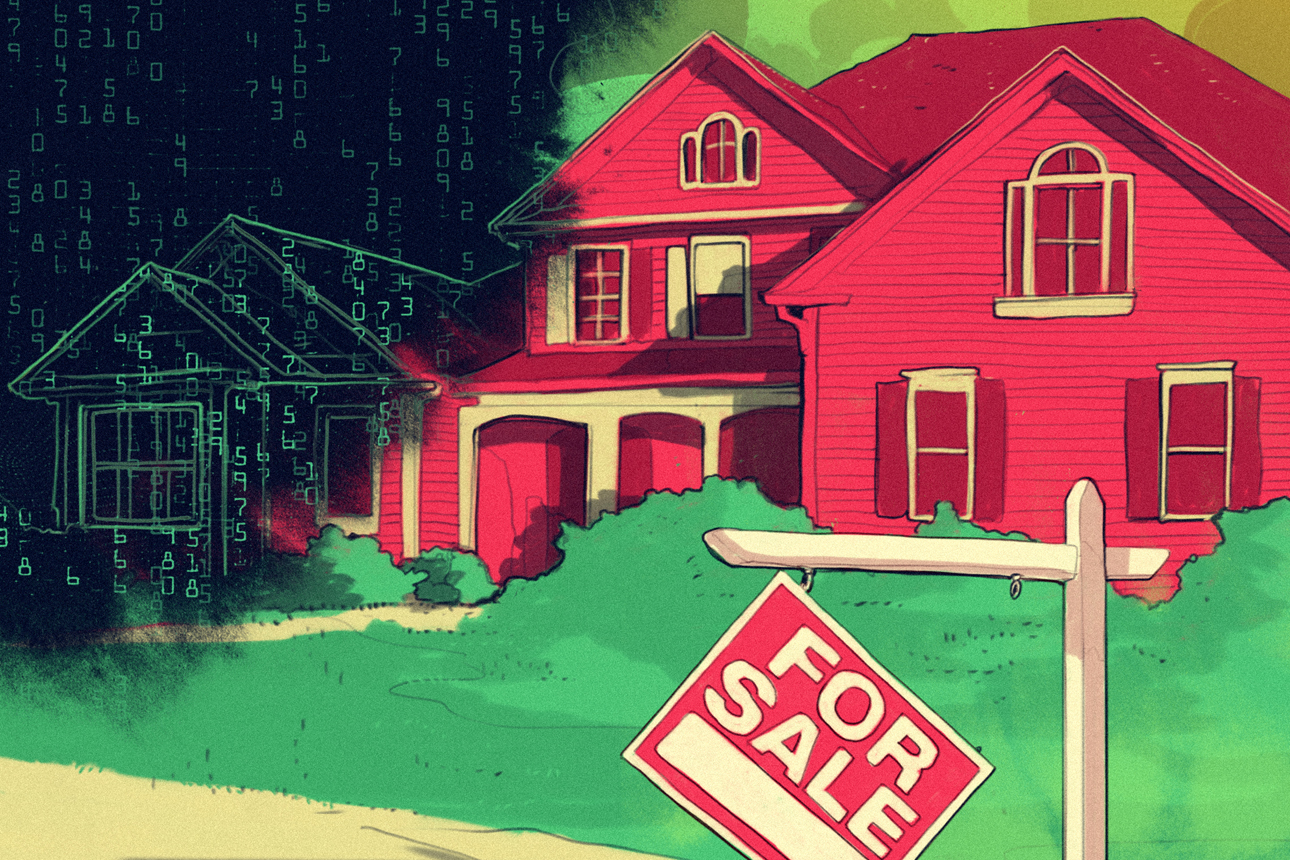
Simon Prades
Organizations are increasingly turning to sophisticated data analytics algorithms to support real-time decision-making in dynamic environments. However, these organizational efforts often fail — sometimes with spectacular consequences.
In 2018, real estate marketplace Zillow launched Zillow Offers, an “instant buyer” arm of the business. It leveraged a proprietary algorithm called Zestimate, which calculates how much a given residential property can be expected to sell for. Based on these calculations, Zillow Offers planned to purchase, renovate, and resell properties for a profit.1 While it had some success for the first few years, the model failed to adjust to the new dynamics of a more volatile market in 2021. Zillow lost an average of $25,000 on every home it sold in the fourth quarter that year — resulting in a write-down of $881 million.2
This is an example of what we call algorithmic inertia: when organizations use algorithmic models to take environmental changes into account but fail to keep pace with those changes. In this article, we explain algorithmic inertia, identify its sources, and suggest practices organizations can implement to overcome it.
Get Updates on Leading With AI and Data
Get monthly insights on how artificial intelligence impacts your organization and what it means for your company and customers.
Please enter a valid email address
Thank you for signing up
A Credit-Rating Catastrophe
To understand the phenomenon of algorithmic inertia, we conducted an in-depth study of another organization that failed to respond to changes in the environment: Moody’s, a financial research firm that provides credit ratings for bonds and complex financial instruments such as residential mortgage-backed securities (RMBSs). These securities aggregated bundles of individual mortgages into distinct tranches with unique characteristics during the period leading up to the global financial crisis of 2008.
References
1. C. Stokel-Walker, “Why Zillow Couldn’t Make Algorithmic House Pricing Work,” Wired, Nov. 11, 2021, www.wired.com.
2. W. Parker, “Zillow’s Shuttered Home-Flipping Business Lost $881 Million in 2021,” The Wall Street Journal, Feb. 10, 2022, www.wsj.com.
3. O. Omidvar, M. Safavi, and V.L. Glaser, “Algorithmic Routines and Dynamic Inertia: How Organizations Avoid Adapting to Changes in the Environment,” Journal of Management Studies 60, no. 2 (March 2023): 313-345.
4. “Justice Department and State Partners Secure Nearly $864 Million Settlement With Moody’s Arising From Conduct in the Lead Up to the Financial Crisis,” U.S. Department of Justice, Jan. 13, 2017, www.justice.gov.
5. “The Financial Crisis Inquiry Report: Final Report of the National Commission on the Causes of the Financial and Economic Crisis in the United States,” PDF file (Washington, D.C.: Financial Crisis Inquiry Commission, January 2011), www.govinfo.gov.
6. Ibid.
7. A. Rona-Tas and S. Hiss, “The Role of Ratings in the Subprime Mortgage Crisis: The Art of Corporate and the Science of Consumer Rating,” in “Markets on Trial: The Economic Sociology of the U.S. Financial Crisis: Part A,” eds. M. Lounsbury and P.M. Hirsch (Bingley, U.K.: Emerald Group Publishing, 2010), 115-155.
8. “The Financial Crisis Inquiry Report.”
9. J. Siegel, “Moody’s Mortgage Metrics: A Model Analysis of Residential Mortgage Pools” (New York: Moody’s Investors Service, April 1, 2003).
10. “The Financial Crisis Inquiry Report.”
11. D. Lindebaum, V. Glaser, C. Moser, et al., “When Algorithms Rule, Values Can Wither,” MIT Sloan Management Review 64, no. 2 (winter 2023): 66-69.
12. V.L. Glaser, “Design Performances: How Organizations Inscribe Artifacts to Change Routines,” Academy of Management Journal 60, no. 6 (December 2017): 2126-2154.
13. R.W. Hoerl, D. Kuonen, and T.C. Redman, “To Succeed With Data Science, First Build the ‘Bridge,’” MIT Sloan Management Review, Oct. 22, 2020, https://sloanreview.mit.edu.
14. Ibid.