Preserving Privacy While Sharing Data
Differential privacy can safeguard personal information when data is being shared, but it requires a high level of expertise.
Topics
Frontiers
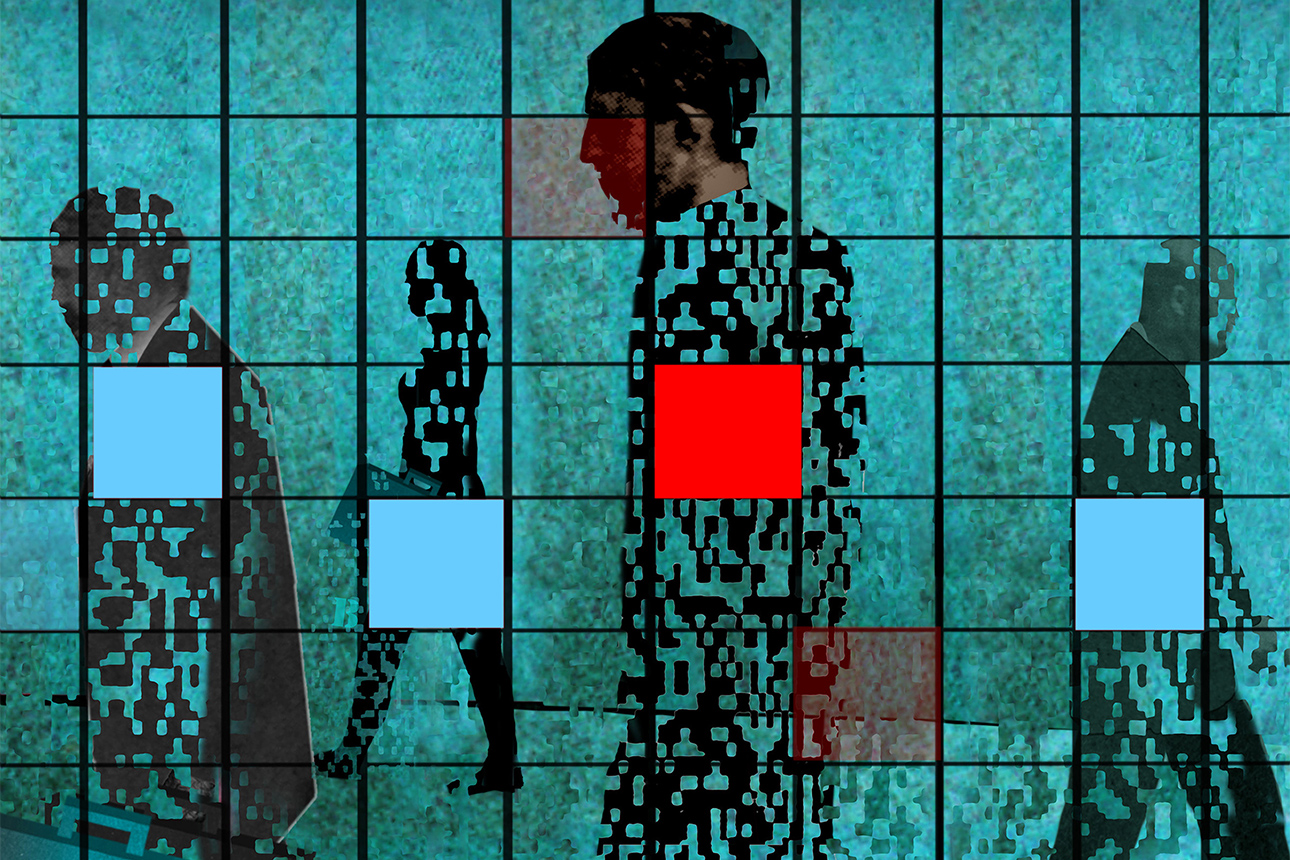
Michael Morgenstern/theispot.com
As organizations increasingly seek to exploit data, both for internal use and for sharing with partners in digital ecosystems, they face more laws mandating stronger consumer privacy protections. Unfortunately, traditional approaches to safeguarding confidential information can fail spectacularly, exposing organizations to litigation, regulatory penalties, and reputational risk.
Since the 1920s, statisticians have developed a variety of methods to protect the identities and sensitive details of individuals whose information is collected. But recent experience has shown that even when names, Social Security numbers, and other identifiers are removed, a skilled hacker can take the redacted records, combine them with publicly available information, and reidentify individual records or reveal sensitive information, such as the travel patterns of celebrities or government officials.
Get Updates on Leading With AI and Data
Get monthly insights on how artificial intelligence impacts your organization and what it means for your company and customers.
Please enter a valid email address
Thank you for signing up
The problem, computer scientists have discovered, is that the more information an organization releases, the more likely it is that personally identifiable information can be uncovered, no matter how well those details are protected. It turns out that protecting privacy and publishing accurate and useful data are inherently in opposition.
In an effort to tackle this dilemma, computer scientists have developed a mathematical approach called differential privacy (DP), which works by making that trade-off explicit: To ensure that privacy is protected, some accuracy in the data has to be sacrificed. What’s more, DP gives organizations a way to measure and control the trade-off. Many researchers now regard DP as the gold standard for privacy protection, allowing users to release statistics or create new data sets while controlling the degree to which privacy may be compromised.
How Differential Privacy Works
Invented in 2006, DP works by adding small errors, called statistical noise, to either the underlying data or when computing statistical results. In general, more noise produces more privacy protection — and results that are less accurate. While statistical noise has been used for decades to protect privacy, what makes DP a breakthrough technology is the way it gives a numerical value to the loss of privacy that occurs each time the information is released. Organizations can control how much statistical noise to add to the data and, as a result, how much accuracy they’re willing to trade to ensure greater privacy.1
The U.S. Census Bureau developed the first data product to use DP in 2008. Called OnTheMap, it provides detailed salary and commuting statistics for different geographical areas.
References
1. While we will not explore the mathematics of DP here, readers who wish to know more are directed to C.M. Bowen and S. Garfinkel, “The Philosophy of Differential Privacy,” Notices of the American Mathematical Society 68, no. 10 (November 2021): 1727-1739; and A. Wood, M. Altman, A. Bembenek, et al., “Differential Privacy: A Primer for a Non-Technical Audience,” Vanderbilt Journal of Entertainment and Technology Law 21, no. 1 (fall 2018): 209-276.
2. For a discussion of the controversy involving the deployment of DP and the 2020 U.S. Census, see S. Garfinkel, “Differential Privacy and the 2020 U.S. Census,” MIT Case Studies in Social and Ethical Responsibilities of Computing (winter 2022), mit-serc.pubpub.org.