Creating Business Value with Analytics
Our new survey suggests that companies experienced in analytics use are increasingly gaining competitive advantage — but their approaches vary.
Topics
Competing With Data & Analytics
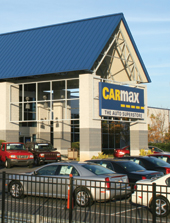
A proprietary information system helped make CarMax the largest specialty retailer of used cars in the U.S. and the fastest retailer in U.S. history to $1 billion in revenues.
Several prominent technology companies recently predicted that a zettabyte of data will soon be racing about the Internet.1
This raises several important questions, including, just what is a zettabyte? The answer: a nearly unfathomable quantity of data, roughly equivalent to the information contained in 100 million Libraries of Congress.
The next big data measure after zettabyte is a yottabyte. It is not named after a Star Wars character. Describing the size of a yottabyte makes you sound like a 5-year-old: “You know, it’s a thousand trillion billion bytes…” It would take billions of years to download a yottabyte file at current high-speed broadband speeds.
If Internet traffic continues to grow at current rates, we will likely approach the yottabyte milestone before the end of this century.2 At that point or, more likely, long before, we will have to invent some new words for what comes next. The International Organization for Standardization and the International Electrotechnical Commission — the official name givers for this sort of thing — have no words for chunks of data that large.
It is no small problem when words fail to capture the world’s immensity. When old concepts fail to keep up with change, traditions and past experience become inadequate guides for what to do next. When the normal ties between what is known and what is wise, between knowledge and practical wisdom tease apart, a gap emerges and the routes to wisdom shift.
The Leading Question
What kinds of organizations are gaining a competitive advantage from analytics, and how?
Findings
- There is a widening gap between organizations that are gaining advantage.
- Management support for analytics, including sponsors and top-down mandates, is critical.
- Data-oriented cultures have three key characteristics that can be developed and refined.
For managers, the pressure is on to find new approaches to their portion of the zettabyte — to develop new data-oriented management systems that make sense of the enormous amount of data their organizations are generating. The increasing trend toward the use of analytics in business is driven by the need — and the ability — to use data to create not just business value but also competitive advantage.
References
1. A 2011 research study conducted by the research firm IDC (sponsored by EMC) declares that we have already passed the zettabyte threshold. IDC predicts that global data volumes will increase by 29 times over the next 10 years. A more conservative growth figure has been offered by Cisco, which predicts we will achieve a zettabyte of Internet traffic by 2015. See “The 2011 IDC Digital Universe Study,” June 2011, www.emc.com.
2. This claim is based on IDC’s growth rate projections. Even if the yottabyte never appears on the Internet, it may be created elsewhere.
3. S. LaValle, E. Lesser, R. Shockley, M.S. Hopkins and N. Kruschwitz, “Big Data, Analytics and the Path from Insights to Value,” MIT Sloan Management Review 52, no. 2 (winter 2011): 21-32.
4. R. Lal and D. Kiron, “Carmax,” Harvard Business School case studies, prod. no. 505080 (Boston: Harvard Business Publishing, 2005).
5. J. Schafer, “SEO Speedwagon: The Rapid Rise and Sale of Arianna Huffington’s Post,” Feb. 7, 2011, www.slate.com.
6. M. Tushman, D. Kiron and A. Kleinbaum, “BT Plc: The Broadband Revolution (A)” Harvard Business School case studies, prod. no. 407001 (Boston: Harvard Business Publishing, 2006).
7. M. Tushman, D. Kiron and A. Kleinbaum, “BT Plc: The Broadband Revolution (A) and (B),” Harvard Business School case studies, prod. no. 407002 (Boston: Harvard Business Publishing, 2006).
Comments (2)
Steven Weiss
PAUL HOFMANN