Real Talk: Intersectionality and AI
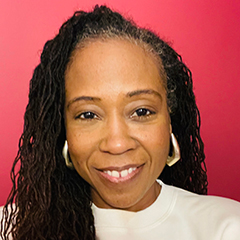
Topics
Column
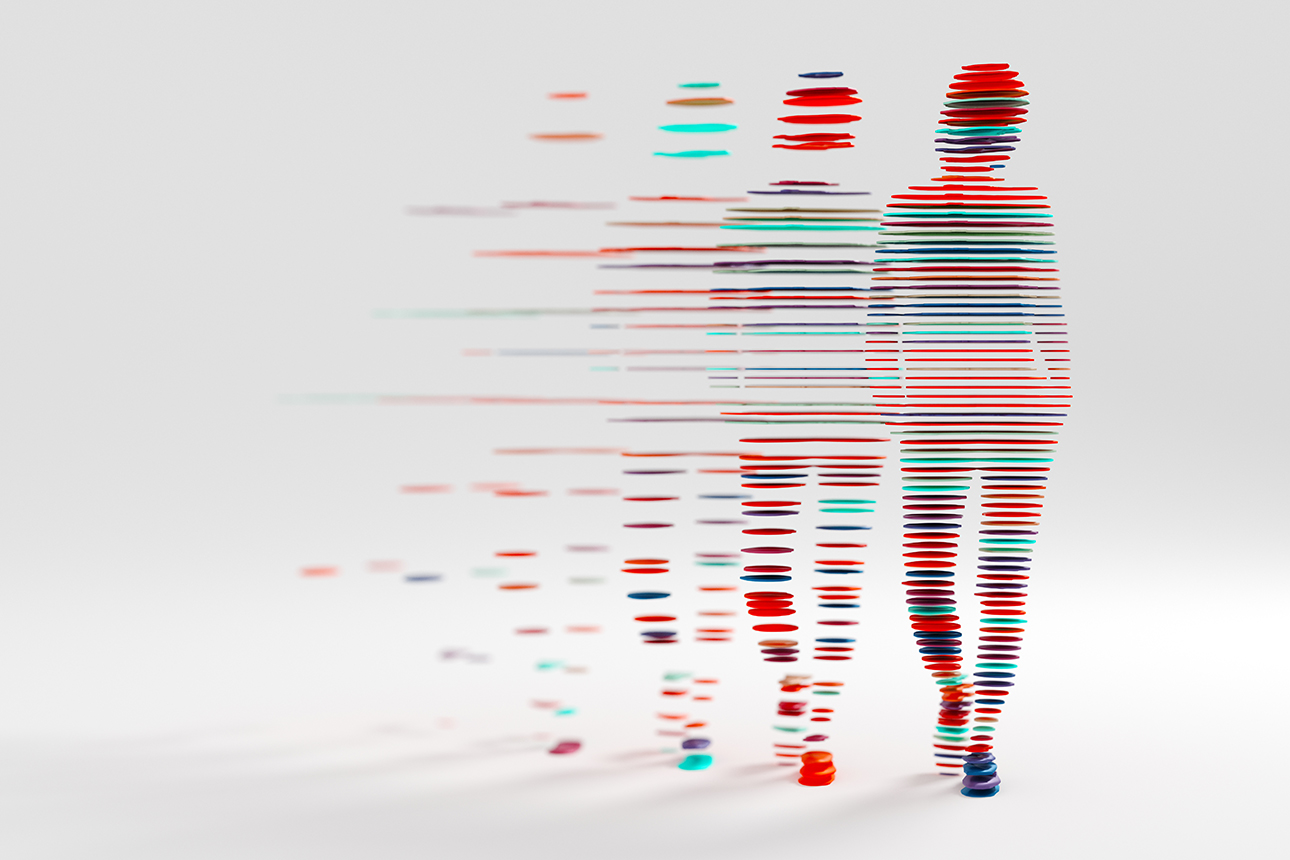
In 1989, Kimberlé Crenshaw, now a law professor at UCLA and the Columbia School of Law, first proposed the concept of intersectionality. In an article published in the University of Chicago Legal Forum, she critiqued the inability of the law to protect working Black women against discrimination. She discussed three cases, including one against General Motors, in which the court rejected discrimination claims with the argument that anti-discrimination law only protected single-identity categories. Black women, the court said, could not be discriminated against based on the combination of identities, in this case race and gender.
Intersectionality, at its core, represents the interconnected nature of our identity. It describes how our race, gender, and disabilities can converge to create systemic structures of discrimination or disadvantage. Intersectionality helps highlight the fact that treating each unique attribute in isolation, such as gender or race, continues to disadvantage those who possess multiple attributes. As Crenshaw wrote, the “intersectional experience is greater than the sum of racism and sexism.”
Get Updates on Leading With AI and Data
Get monthly insights on how artificial intelligence impacts your organization and what it means for your company and customers.
Please enter a valid email address
Thank you for signing up
Although anti-discrimination law fundamentally attempts to prevent discrimination in areas like employment, a growing number of studies highlight the limitations of these laws. They have been inadequate for redressing the issues experienced by those who are disadvantaged based on their lived intersectional experiences. For example, algorithmic bias with respect to Black women cannot be explained away as a sum of the bias with respect to Black men and the bias against White women. One simple example is wages: In 2019, the average woman earned 82 cents for every dollar earned by men. Black women earned 91 cents for every dollar earned by Black men. But compared with White men, Black women earned just 61 cents. Latinas earned even less compared with White men: 53 cents.
All of this has implications in the world of artificial intelligence. Imagine, if you will, what might happen if this real-world data were fed into an AI-based compensation tool for setting pay for new hires or determining promotions.
Intersectionality Challenges in AI
The word intersectionality has been thrown around with good, but not always concentrated, intention in the artificial intelligence world. I and other AI researchers have looked at attributes of diversity in the data sets that contribute to the accuracy of AI algorithms, as well as how that accuracy diminishes when looking at the intersection of these attributes. Joy Buolamwini of the MIT Media Lab and Timnit Gebru, then of Microsoft Research, have discussed how facial recognition algorithms had different outcomes for different intersectionality groups. By examining algorithm performance associated with different human attributes at the intersection of race and gender, Buolamwini and Gebru showcased the significance of conducting intersectional audits for these types of AI systems.
Early work that De’Aira Bryant conducted as part of my research team at the Georgia Institute of Technology’s Human-Automation Systems Lab also looked at this concept of intersectionality and came up with a diversity rating. The rating is based on combining various attributes — including age, gender, and ethnicity — for use in auditing data sets used to train AI algorithms.
Alas, although promising, these efforts are pushed primarily within the AI research community. Adoption of these types of external third-party intersectional audits on systems deployed in consumer-facing applications has been slow. Although companies have been ramping up their efforts to develop fair AI, most of these algorithms still treat human attributes as single, isolated components.
In fact, most AI systems are designed with a single-axis solution in mind — gender is an independent component from age, age is an independent factor from socioeconomic status, and so on. The criteria typically used for computing error rates are limited to a single variable. When accuracy is computed across multiple attributes, each is typically viewed separately.
Take the example of bias in word embedding models, a fundamental AI technique used in many natural language processing applications, ranging from chatbot conversational agents to hate speech detection algorithms. An obvious bias is when a chatbot assumes that “doctor” indicates “man” and “nurse” indicates “woman.” Research has shown that even when language biases based on race, ethnicity, and gender have been mitigated in these word embedding models, the models still display biases against intersectional groups. This was highlighted in work published in 2020 by Wei Guo and Aylin Caliskan, which found that the bias toward intersectionality groups such as “Mexican American females” had worse algorithmic performance than for the single-attribute groups of Mexican Americans or females alone.
Intersectionality Approaches as a Solution for Bias
Addressing intersectionality across an organization is one of the best ways to address larger issues of inclusiveness in the workplace, in the C-suite, and in AI algorithms. Organizations must recognize that all unique intersectional experiences of identity are valid and informative.
I see four specific ways to address the problem within AI applications:
Be intentional in identifying the intersectional groups interacting with your AI system. Look at the ways gender identity, age, ability and/or disability status, and race and/or ethnicity could be at a disadvantage. Look at the ways other groups may have an advantage.
Statistically evaluate metrics among different intersectional groups. Do metrics such as disparate impact rates vary? AI methods rather than brute-force methods where trial and error is used to make guesses about impact are most suited for this type of deep dive. Evaluating performance in this way can assist companies in really getting a grasp on how many individuals are (or are not) being disadvantaged.
Ask what these differences tell you about your system, your processes, or your practices. This is perhaps the most important step. AI has the ability to improve quality of life and well-being for all individuals when carefully crafted. When developing AI systems through an intersectional framework, the magnification of certain biases can be mitigated.
Design a model that purposefully overserves statistically underrepresented intersectionality groups. Put your data to use by designing a model that fixes problems and then some. Believe it or not, research has shown that developing specialized learners that pay special attention to the intersectionality classes yields better results for both the underrepresented and the overrepresented classes. Since algorithms can lack impartiality, designing for intersectionality constraints from the beginning helps mitigate some of the intersectionality bias while not introducing non-intersectionality bias.
Intersectional bias is a real thing in our society, in our community-government interactions, and in our employee-manager interactions. This bias is not just resident at the employee level; it drifts and slithers itself up the management chain and into the C-suite, where 21% of leaders are women, 4% are women of color, and only 1% are Black women. Employees who face discrimination linked to intersectionality have higher turnover rates, which results in an expense that cannot be salvaged. Given that the cost of voluntary turnover in the United States has been estimated to exceed $617 billion, imagine even the low-hanging benefits that could be attained if companies addressed intersectionality in all of their practices — from their workplace hiring efforts to the applications of their artificial intelligence technologies.