Don’t Get Distracted by the Hype Around Generative AI
Tech bubbles are bad information environments.
Topics
Frontiers
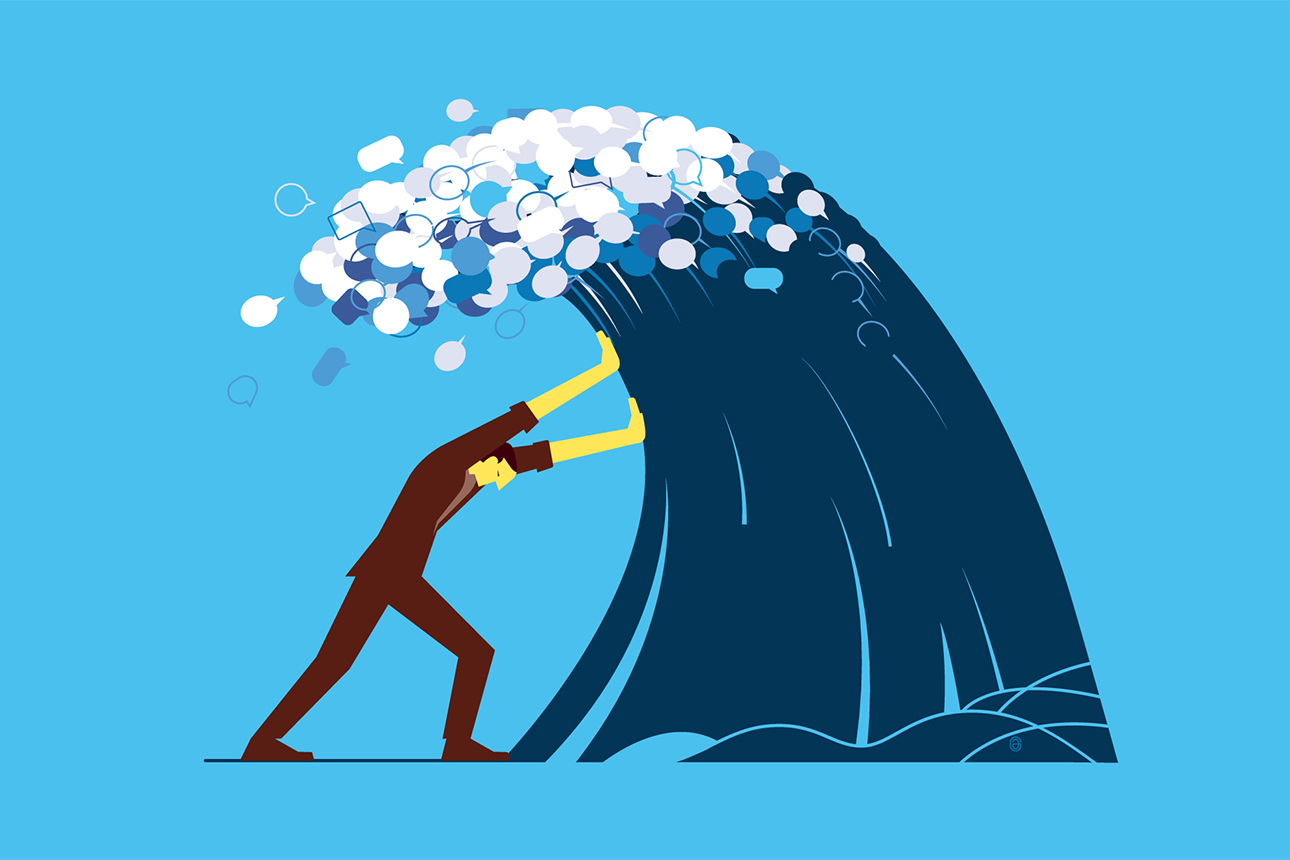
Aad Goudappel/theispot.com
Technology bubbles can pose difficult quandaries for business leaders: They may feel pressure to invest early in an emerging technology to gain an advantage over competitors but don’t want to fall for empty hype. As we enter a period of greater economic uncertainty and layoffs in multiple industries, executives are grappling with questions about where to cut costs and where to invest more.
The rapidly developing field of artificial intelligence and machine learning poses a particular challenge to business decision makers. Investments in proven predictive models are increasingly seen as sound and are expected to drive an increase in spending on AI from $33 billion in 2021 to $64 billion in 2025. But further out on the cutting edge, generative AI is sparking a huge amount of noise and speculation.
Get Updates on Leading With AI and Data
Get monthly insights on how artificial intelligence impacts your organization and what it means for your company and customers.
Please enter a valid email address
Thank you for signing up
Generative AI refers to machine learning models — such as ChatGPT, Bing AI, DALL-E, and Midjourney — that are trained on vast databases of text and images to generate new text and images in response to a prompt. Headlines like “Ten ChatGPT Hacks You Can’t Live Without!” and “You’re Using ChatGPT Wrong! Here’s How to Be Ahead of 99% of ChatGPT Users” have begun to proliferate. Meanwhile, Axios reported that funding is pouring into generative AI, rising from $613 million in 2022 to $2.3 billion in 2023 — money that will only fuel the hype cycle.
Business leaders who don’t want to miss a great opportunity but don’t want to waste time and money implementing oversold technologies would do well to keep in mind some fundamental realities about tech bubbles. First, these phenomena rely on narrative — stories that people tell about how the new technology will develop and affect societies and economies, as business school professors Brent Goldfarb and David Kirsch wrote in their 2019 book, Bubbles and Crashes: The Boom and Bust of Technological Innovation. Unfortunately, the early narratives that emerge around new technologies are almost always wrong. Indeed, overestimating the promises and potencies of new systems is at the very heart of bubbles.
Business futurists and analysts have terrible track records when it comes to accurately predicting the future of technological development, because no one can foresee the creative ways in which humans will adopt and implement tools over time or the myriad opportunistic ways in which humans will use new tools to exploit or gain power over others.