The Rise of Connector Roles in Data Science
Connectors can bridge the organizational gaps that often thwart success with data science projects. Here’s how to manage this new kind of data professional.
Topics
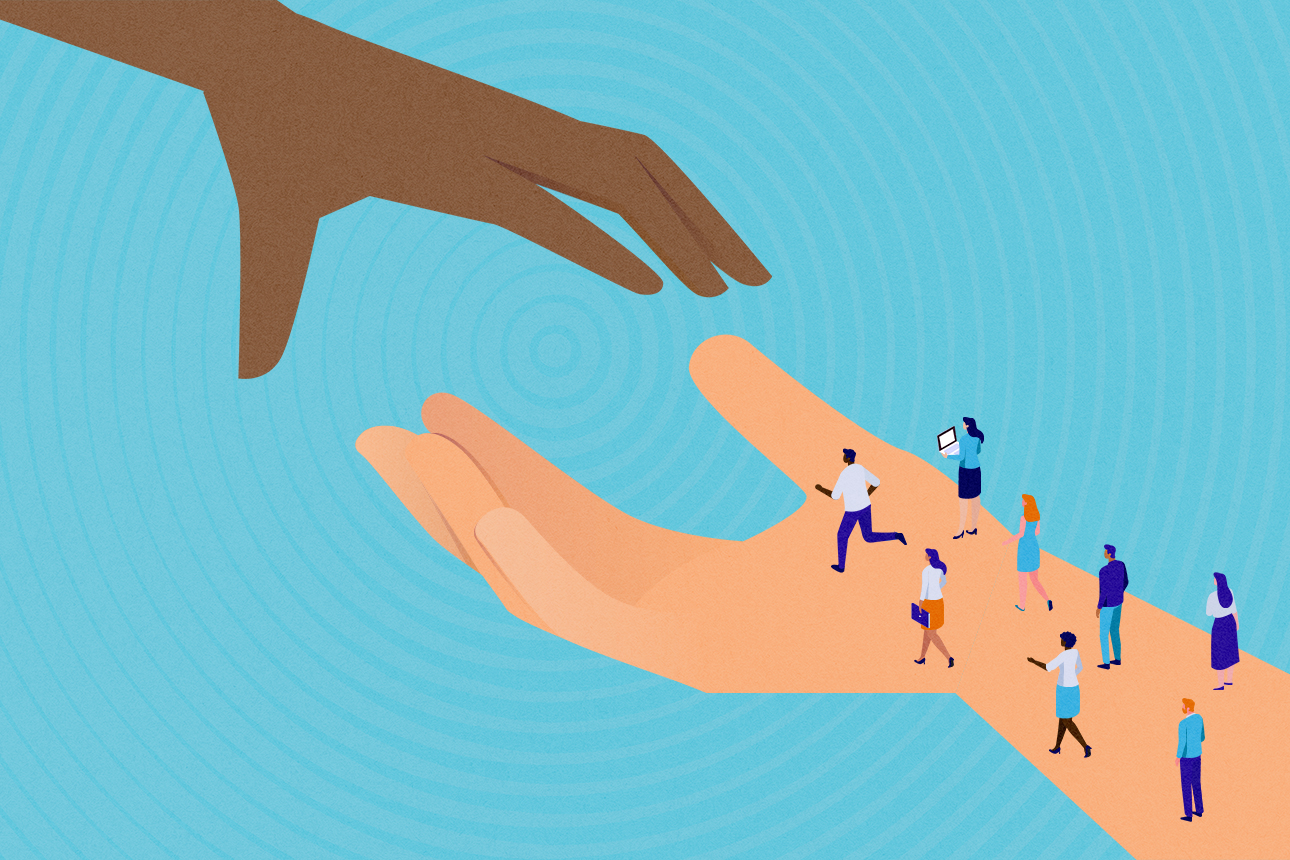
Carolyn Geason-Beissel/MIT SMR | Getty Images
For all of the current focus on using data, analytics, and AI to improve organizational decisions and operations, too many data science projects fail. Even for those that succeed, progress is often slow and expensive. Why? Organizational gaps between teams are wreaking havoc with the ability to develop, apply, and scale data science projects. A new type of role is needed to bridge these gaps.
Let’s not minimize the many aspects of data science that can trip a company up — from defining the right business problem to developing a solution and then supporting it properly. However, our diagnosis of the situation, researched in part with our colleagues Roger Hoerl and Diego Kuonen, shows that many companies struggle less with the solution and more with the organizational issues.
Get Updates on Innovative Strategy
The latest insights on strategy and execution in the workplace, delivered to your inbox once a month.
Please enter a valid email address
Thank you for signing up
We’ve identified three root causes for this. First, data science has been “bolted on” to the organizational chart. This might have been acceptable a generation ago, when data science was reserved for relatively few problems, but it needs to be built in now that data science matters to every team.
Second, data science aims to improve day-to-day operations and decision-making, and that means disruption. But most line managers strive for control, predictability, and no surprises. Given that disruption and control are polar opposites, there is a far greater-than-normal tension between line management and data science.
Third, many companies seem to expect data scientists to do it all: magically understand the business and all its nuances, deal with data quality issues, and convince recalcitrant managers and staff members to change their long-cherished processes. Many data scientists prefer to simply create better algorithms, which means those other tasks often receive short shrift.
There is no minimizing these issues — they are deep, structural, and often political and may well demand additional management strategies. Here, we will address one critical aspect of the organizational problem, namely the gaps between the various departments that inhibit the development and deployment of data science models.
Closing the Gaps With Connectors
The obvious way to close organizational gaps is to fill them with people. We generically call people in these roles connectors. For some time, companies have assigned people who have job titles such as systems analyst, business analyst, coverage officer, and systems engineer to close gaps between business and technical departments.
Comment (1)
Shubharanjan Dasgupta