The Working Limitations of Large Language Models
Overestimating the capabilities of AI models like ChatGPT can lead to unreliable applications.
Topics
Frontiers
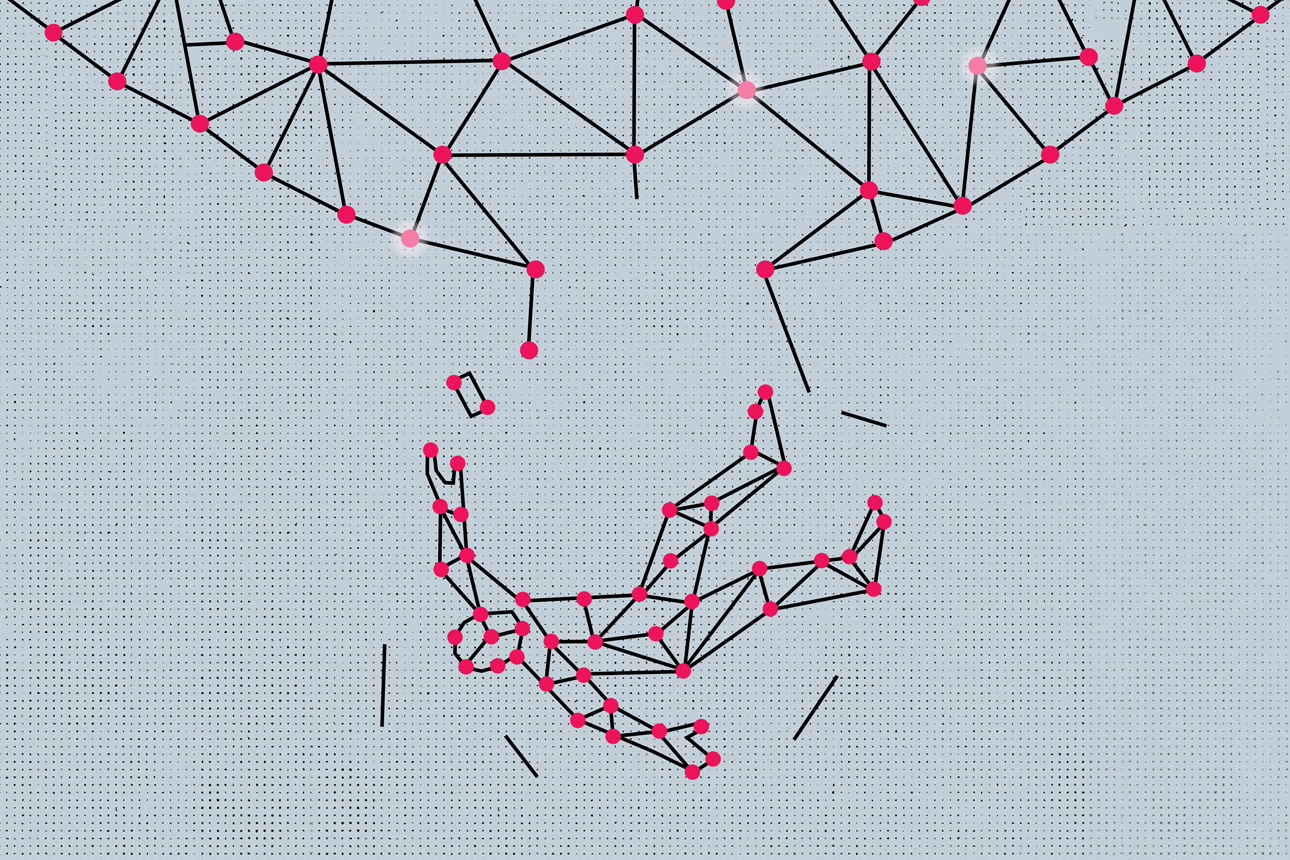
Dan Page/theispot.com
Large language models (LLMs) seem set to transform businesses. Their ability to generate detailed, creative responses to queries in plain language and code has sparked a wave of excitement that led ChatGPT to reach 100 million users faster than any other technology after it first launched. Subsequently, investors poured over $40 billion into artificial intelligence startups in the first half of 2023 — more than 20% of all global venture capital investments — and companies from seed-stage startups to tech giants are developing new applications of the technology.
But while LLMs are incredibly powerful, their ability to generate humanlike text can invite us to falsely credit them with other human capabilities, leading to misapplications of the technology. With a deeper understanding of how LLMs work and their fundamental limitations, managers can make more informed decisions about how LLMs are used in their organizations, addressing their shortcomings with a mix of complementary technologies and human governance.
Get Updates on Leading With AI and Data
Get monthly insights on how artificial intelligence impacts your organization and what it means for your company and customers.
Please enter a valid email address
Thank you for signing up
The Mechanics of LLMs
An LLM is fundamentally a machine learning model designed to predict the next element in a sequence of words. Earlier, more rudimentary language models operated sequentially, drawing from a probability distribution of words within their training data to predict the next word in a sequence. (Think of your smartphone keyboard suggesting the next word in a text message.) However, these models lack the ability to consider the larger context in which a word appears and its multiple meanings and associations.
The advent of the latest neural network architecture — transformers — marked a significant evolution toward modern LLMs. Transformers allow neural networks to process large chunks of text simultaneously in order to establish stronger relationships between words and the context in which they appear. Training these transformers on increasingly enormous volumes of text has led to a leap in sophistication that enables LLMs to generate humanlike responses to prompts.
This ability of LLMs hinges on several critical factors, including the model’s size, denoted by the number of the trainable weights (known as parameters), the quality and volume of the training data (defined by number of tokens, referring to word or subword units), and the maximum size of input the model can accept as a prompt (known as its context window size).