There’s Always a Time Lag (With a Price Tag)
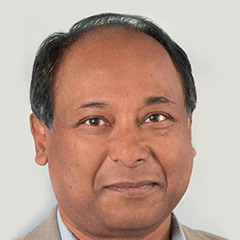
Topics
Column
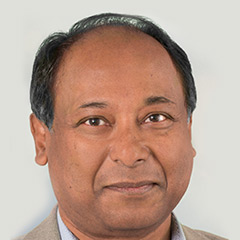
Amit S. Mukherjee is a professor of leadership and strategy at IMD’s Singapore campus and president of Ishan Advisors LLC, a boutique consulting and executive education company. He has led technology development teams, served as an executive officer of a public company, and advised CEOs of global companies on strategy and organization design.
Leaders in business perennially seem to under-appreciate the fact that technological changes occur (much) faster than associated, necessary — even essential — non-technological changes. This time lag usually has a very high price tag.
This pattern is currently playing out in relation to artificial intelligence. Leaders must act to mitigate it, if AI is to benefit society.
The Price of the Time Lag, Circa 1985
To understand how this dynamic works, consider how it unfolded in the development of the modern quality movement.
The U.S. invented statistical process control methodologies in the 1920s and deployed these in the weapons industries just before World War II. Post-war, Japan’s civilian industries adopted these processes, making simple and sophisticated additions (for example, Ishikawa diagrams and the Taguchi method, respectively). Japanese companies packaged these changes with new production control methodologies, such as just-in-time manufacturing. Collectively, these adaptations powered Japan’s rebirth. By the early 1980s, they enabled Japan’s successful economic challenge to the hitherto dominant West.
During the early 1980s, Western companies struggled to respond. They failed to appreciate that quality technologies demanded a new organizational structure — teams — and a new leadership philosophy — empowerment.
This time lag had an immediate, massive cost: a rise in U.S. unemployment rates and corporate bankruptcies. (Those same levels would be echoed later in the downturns of 2009.) Decades later, we are still struggling at a societal level, because U.S. secondary schools still don’t reliably prepare graduates for entry-level jobs anchored on quality technologies.
Today’s Conversation Around AI Is Insufficient
Two discussions of AI that I attended over the last year suggest we are absorbing the spread of artificial intelligence as ineffectively as we did quality technologies.
Get Updates on Leading with AI and Data
Monthly insights on how artificial intelligence impacts your organization and what it means for your company and customers.
Please enter a valid email address
Thank you for signing up
At one conference, a senior partner of a global consultancy urged his audience not to fear AI. Technologies require “one to three decades” for the “plateauing of adoption,” he said. CEOs were making implementation decisions incrementally, since the key value (that is, ROI) lay in improving existing marketing and operations systems. AI wouldn’t produce large-scale changes in organizations any time soon because AI could automate only 50% of an average job. Indeed, only roughly 1% of businesses had implemented AI at scale. Overall, the speaker’s comments implied that CEOs were safe in taking a reactive, and even relaxed, approach to this emerging technology.
Half a world away, three corporate AI experts and an academic complained about the environment in which they worked. Most existing companies weren’t deploying AI broadly. This failure was depriving AI of access to the massive amounts of data — “the next natural resource” — it needed to see patterns and ask, not merely answer, questions. People such as Elon Musk, founder of Tesla and Neuralink, who are urging regulatory oversight of AI to prevent it from becoming an existential threat are flat-out wrong, these folks argued; such naysayers would slow the progress to “new-collar jobs” that would “augment humans” and enable “self-goal setting” that would let people lead more meaningful lives, with more free time. Where the consultant speaking at the conference had reassured his audience that they had time before they had to think too deeply about AI, the experts in this room were anxious to barrel forward as fast as possible — with little regard to AI’s human costs.
In fact, there has been remarkably rapid progress on developing sophisticated AI. In 1997, AI was expected to be able to play (and win) the Chinese board game Go in 100 years; in 2014, the estimate dropped to 10 years. By 2015 a system was actually competing, and in 2016, AlphaGo, the artificially intelligent player developed by Google DeepMind, beat Lee Sedol, a top world player. Independent incarnations of IBM’s Watson are now targeting 50 different problem areas for the computer system to work on.
The Price of Today’s Time Lag Around AI
Experts making predictions about their fields have a long track record of being spectacularly wrong. And so it will be with AI: Regardless of the difficulties the technologists previously anticipated, AI’s success with Go suggests that its development and use will accelerate rapidly.
AI’s development is moving far faster than that of previous technologies. It took decades, for instance, for IBM’s Deep Blue computer to learn enough about chess to defeat the game’s grandmaster, Garry Kasparov, in 1997, and Go presented AI with a far tougher challenge than did chess. A model that is over 75 years old — the experience curve — suggests why this is: The more any technology is used, the more opportunities to improve it are identified. The growing applications of Google’s DeepMind, the 50 incarnations of IBM’s Watson, and other such efforts will inevitably win the day.
Along the way, today’s AI will make decisions driven solely by technological needs. AI teams will choose readily available data sets, without considering whether the data represents the population at large or poses ethical conundrums. AI will produce the best “intellectual” decisions, without considering whether these decisions can be explained to nonexperts. AI will inadvertently base predictions on data sets that assume a stable future. Without any individual having any explicit bias, AI development teams will produce biased outcomes.
ROI-driven, incremental decision-making by CEOs around AI is problematic — and will become more so. If CEOs make small, gradual additions, they are not likely to spend the money needed to get good data. Projects initiated this way are also more likely to fall prey to an insidious challenge: As AI algorithms are being shared across organizations and geography, they are spreading their shortcomings.
As in the case of the quality movement, executives are not pondering whether they should fundamentally reorganize companies to better use AI, according to a global survey by MIT Sloan Management Review and The Boston Consulting Group. And researchers are not commenting on this failure.
The solution is not formally incorporating AI systems as voting members of executive teams, as one Finnish company has done. That would merely give a voting right to big data without doing the same for, say, creativity.
How Leaders Must Respond to the Gap
That decades may pass before the adoption of AI plateaus should be irrelevant to real leaders. As with prior technologies, leaders will face profound challenges much earlier.
I asked the consultant-speaker to estimate when driverless vehicles would become common. In cities like Cairo, Mumbai, and Jakarta, becoming a professional driver gives millions of people a tenuous path to the very lowest rung of the local middle class. There should be conversations around whether business leaders have any responsibility to help address the upheaval that will accompany this rung’s removal. But the consultant didn’t answer. After the session, many attendees commented on the expert’s nonresponse.
We routinely hold our peers and subordinates accountable for their actions or nonactions. But when it comes to AI, CEOs and other experts seem to be escaping this standard. Instead of challenging, shaping, guiding, and choosing, they are merely reacting. They are not considering broad consequences nor being responsible stewards of valuable assets.
Given the speed of AI’s technological development, incremental decision-making by CEOs won’t help with the issues AI will invariably raise. Indeed, it will likely exacerbate the time-lag problems that will arise at a societal level — problems like people losing their jobs and challenges like reeducating humans in knowledge-intensive fields. Nor will localized decision-making help. At best, it will lead to suboptimal solutions, with potential benefits undermined or reversed by equally local decisions made by customers, suppliers, partners, regulators, external pressure groups, and other stakeholders.
And real leaders, particularly CEOs, should avoid making a fundamental error that the speakers in both sessions made: Faced with a technology that demands knowledge, they focused only on how their decisions would affect the most well-educated people in organizations. Businesses, however, do not exist in a vacuum, isolated from society.
Real leaders should always keep in mind that AI, like all technology, has the power to help some humans improve their lives and the power to violently disrupt the lives of others. The day executives forget this is the day they cease to be leaders.
Comment (1)
ashish