Governance for Smarter KPIs
Effective governance will ensure that KPIs evolve, remain aligned with strategic aspirations, and are trusted by workers and managers alike.
Topics
Artificial Intelligence and Business Strategy
In collaboration with
BCG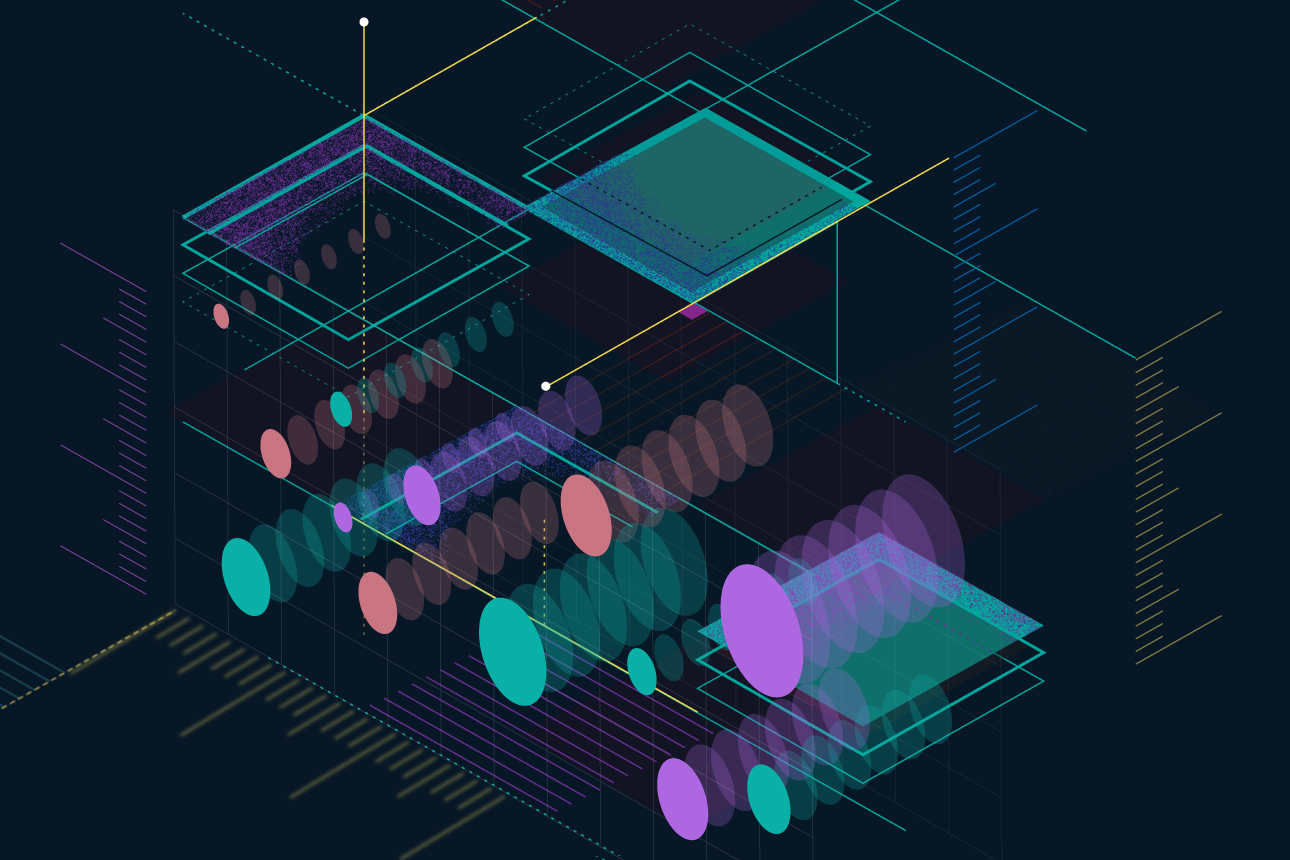
Without adaptation and refinement, static business metrics create two types of strategic risk. One is encouraging performance on the wrong measures. The 2008 global economic crisis, for example, was triggered in part by banks’ dependence on a then widely used metric: value at risk, which measures potential portfolio losses in normal market conditions at a single point in time. Financial institutions did not adjust this measure as riskier subprime mortgages and securitized default swaps became a larger part of their portfolios. Guided by a metric that severely underestimated potential losses — in some cases, by orders of magnitude — many financial institutions went bankrupt or suffered significant losses.
Get Updates on Leading With AI and Data
Get monthly insights on how artificial intelligence impacts your organization and what it means for your company and customers.
Please enter a valid email address
Thank you for signing up
Opportunity costs represent another risk from static key performance metrics. Our research persuasively demonstrates that companies deliberately using artificial intelligence to design and create more dynamic KPIs enjoy greater situational awareness, stronger ties between operations and strategic outcomes, and improved results overall.1 These smart KPIs reflect deeper understandings of performance drivers and produce more reliable predictions about future outcomes than comparable KPIs not informed by AI. (See “Three Types of Smart KPIs.”) A “set them and forget them” approach to key metrics is neither desirable nor sustainable in volatile and fast-changing markets.
It takes effective governance to ensure that KPIs evolve, remain aligned with strategic aspirations, and are trusted by workers and managers alike. As AI-infused workflows and processes colonize and expand business capabilities, leaders need to update and upgrade their key performance indicators to keep up. From Schneider Electric to Walmart to Maersk, we see strategists, technologists, and business leaders working together to refine and enhance KPIs to ensure their alignment with evolving organizational goals.
Among these companies, responsibility for making KPIs more predictive, dynamic, and insightful extends beyond strategists and business leaders. They encourage technologists to make a material contribution to the formulation, organization, and prioritization of KPIs. That’s a big change from the way companies have traditionally established and updated KPIs.
As Schneider Electric chief governance officer and secretary general Hervé Coureil observes, “When processes are digitized, they start to produce ‘exhaust data’ that can be turned into relevant telemetry metrics, providing insights on process behaviors, defects, etc. Secondly, digital transformation triggers significant decisions and investments — portfolio and investment choices, business model implementation, new systems and infrastructure — and therefore, there is a need to measure the impact of those decisions as well as the effectiveness of the various programs that comprise the transformation.”
As AI-infused workflows and processes colonize and expand business capabilities, leaders need to update and upgrade their key performance indicators to keep up.
Developing such measures depends on more than executive judgment, intuition, and experience. Transforming static, legacy performance metrics into smarter, more dynamic KPIs demands a greater role for data, analytics, and AI. We suggest that leaders adopt a three-pronged approach to governing the development and use of AI-driven KPIs and, more broadly, their strategic measurement systems. This governance approach rests on three factors:
- A solid data foundation with responsible data and analytics practices.
- Organizational structures with responsibility and accountability for evolving KPIs.
- Trust in KPIs trained to adapt to and learn from changes in circumstances.
Governance of Smart KPI Development: Get Your Data in Order
Effective KPI governance requires effective data governance. Every organization participating in our research emphasized data quality and discipline as the technical enabler for its AI-driven KPI initiatives. Most legacy IT and database systems, however, aren’t configured to generate or make accessible the quality data necessary for measuring or defining smart KPIs.
Pierre-Yves Calloc’h, the chief digital officer at Pernod Ricard, recalls that the spirits giant realized it needed three years’ worth of weekly consumer sales data to enable meaningful AI interventions. A preliminary review revealed huge gaps. “When we started, we had almost no data governance,” says Calloc’h. “Almost 80% of the data we needed wasn’t there because it was mainly external data. Our statistical experience is that you need weekly data; if you have monthly data, it doesn’t work, so you need to source the information manually. We even called it ‘archeology’ because of the level of effort needed, even going into old PowerPoint and Excel files in mailboxes. In addition, you need a strong business case for the project, because without clear benefits in mind, people will not invest the time.” Essentially, the business case for AI-powered KPIs could not be made without technically reliable and robust governance that transformed data archaeology into data architecture.
Jon Francis, General Motors’ chief data and analytics officer, who previously oversaw Starbucks’s digital transformation, echoed Calloc’h’s observations. “It’s actually really hard to just create a KPI because of the underlying data challenges,” he says. “It takes all of your effort, usually, to create the KPI and report on it.” Poor data and metadata governance undermines an organization’s ability to develop the right metrics. Francis notes that technology systems are often just “spooling data that’s not defined or useable” and, as a result, “you end up with these data dumping grounds.” He candidly observes that for most organizations, the generation of data from production systems is an afterthought.
Effective KPI governance remains contingent upon a company’s technological capabilities to harness data: It demands diligent data governance, a structured approach to analytics management, and a path for technologists and business unit leaders to collaborate on developing optimal KPIs on an ongoing basis.
Embedding Governance in Teams or Functions
Our research finds top management rethinking how to develop and continuously improve their key performance measures and metrics. While approaches vary, a common theme among interviewed executives is choosing to locate responsibility for transforming KPIs in a specific team or group of teams.
At Schneider Electric, leadership came to recognize that the company’s legacy metrics could become incompatible with its aspirations to strategically collaborate and innovate with its global customers. Coureil helped sponsor the creation of a Performance Management Office within the company’s data team to oversee performance measurement. The office was deliberately positioned in the governance team to ensure a neutral cross-functional perspective.
The Performance Management Office helped top management transform their portfolio of KPIs and align them with operations. It also pushed the businesses to become far more data-driven and aware of the links between improvement against a given performance metric and enhanced outcomes. “Measurement has been very core for us,” adds Coureil. “We actually tried to make metrics a complete, integral part of our digital transformation, as opposed to an afterthought.”
What’s the desired outcome of a given KPI? “It’s an ontology or taxonomy question at some point: What behaviors are you looking for?” asks Coureil. “I think people put a lot of things in the KPI basket, and we’ve spent a lot of time trying to be extremely clear about what we’re looking at: What is the desired outcome? It looks very simple, but it is not that simple, actually … being extremely sharp on what the desired outcome of a given KPI is.” That’s why having a performance management office is proving to be essential to strategically coherent and consistent KPI governance, Coureil says.
Instead of using a performance management office, DBS Bank governs KPI development through cross-functional teams that create value maps linking customer journeys to desired outcomes. The Singapore-based financial services leader revisited its operating model to reflect the fact that using smarter KPIs could measurably improve outcomes for both the business and its customers. “Over the past three years, we moved to something we call a data-driven operating model,” says group chief analytics officer and managing director Sameer Gupta.
At the heart of the model are cross-functional squads. Representatives from various functions, such as product, risk, data, marketing, call center, and human resources, form a squad, Gupta explains. Every squad member is then aligned on the same set of outcomes mapped to four categories: customer experience, employee experience, profitability, and risk.
The factors driving performance on each outcome are represented in a value map that connects performance metrics with the four types of outcomes. Each squad is responsible for identifying and optimizing these factors. “The squads look at optimizing those drivers,” says Gupta. “We look at many things to optimize those drivers: experimentation, data, customer immersion, AI, machine learning — all kinds of tools to try and continuously optimize in coordination and consultation with control towers and management dashboards.”
The result, Gupta notes, is not just greater data-driven operational efficiencies and effectiveness but KPIs that expand the boundaries of the DBS client and customer experience. Revisiting processes for KPI governance have led to both better KPIs and better KPI outcomes.
While the specific approaches vary, most leaders we spoke with are in the early stages of building governance structures to help make their KPIs more intelligent, detailed, and predictive.
Effective KPI Governance Is Based on Trust
While these technological and organizational investments in KPI governance are crucial to enhancing KPIs, our research suggests that their practical value to teams is contingent upon people’s willingness to trust them. During Schneider Electric’s digital transformation efforts, Coureil recognized that “one of the changes, versus a more traditional way of doing business, is that you don’t build your dashboard anymore. Somebody else does it for you. So you have to trust those metrics.”
Anecdotal evidence indicates that establishing trust in AI-sourced metrics can be challenging.
At Maersk, for instance, the long-held assumption was that getting a vessel out of port and on its way to the next one as quickly as possible was ideal. A digital twin simulation, however, showed that being on time was better than being early: With an end-to-end connected schedule, as is also the situation for commercial airlines, the port that a vessel is heading to next can’t adjust its schedule to immediately accommodate earlier arrivals. In other words, “faster isn’t better” was a counterintuitive assertion from the data team, and it was only after the on-time approach was put into practice that the front-line operators — and even those at the most senior levels managing the entire terminal — were finally convinced.
Cultivating the trust necessary to develop and use dynamic, AI-enhanced KPIs is a critical leadership and governance challenge. Developing data-savvy managers who are receptive to developing and embracing AI-driven KPIs is a significant part of that challenge. At one media organization, an executive interviewee noted that she’d had a hard time persuading her superiors to look at KPIs and make decisions based on data. Their usual retort was that executives are “paid millions for their gut instincts” — in other words, experience and intuition were valued over data-driven insight.
Cultivating the trust necessary to develop and use dynamic, AI-enhanced KPIs is a critical leadership and governance challenge.
Building a data-oriented management community is one way to create trust among the leaders using enhanced KPIs. At Sanofi, the most senior 150 leaders, representing various functions and businesses, are being trained in boot camps to become more data-centric and to seek out more information before making decisions. As chief digital officer Emmanuel Frenehard says, “Our goal as part of our cultural change is that these 150 people, when they leave the boot camp, are far more inclined to adopt that data-centric view and better understand the potential of AI for us. We are making sure that the core of our leadership is trained on how to use the next generation of AI-driven KPIs.”
Further Considerations
To ensure that their AI-enabled smart KPIs are setting them up for strategic success, leaders must prioritize the technological, organizational, and cultural dimensions of KPI governance discussed above. As they begin to do so, these related considerations should be top of mind.
Establish a steering committee to guide continuous improvement. Transforming legacy KPIs into enhanced KPIs demands regular experimentation to ensure that key performance is focused on the right outcomes. Determining where this experimentation happens, who does it, and how often are critical leadership and governance decision points. Is it in a central team, like a performance office, or is it more widely distributed? Should certain KPIs be revisited and reviewed more frequently than others? Who has decision rights when changing KPIs changes accountability? There are no one-size-fits-all answers to these questions. Leaders need flexible processes to effectively govern KPIs that might be siloed or cross-functional. We suggest establishing a cross-functional KPI steering committee with business and technical expertise to elicit necessary input and buy-in from relevant stakeholders.
Oversee investment in capabilities to optimize groups of KPIs. Does your company have the capability to assess whether its KPIs are good enough or fully optimized? Our research suggests that AI can play a valuable role in assessing KPI quality, prioritizing KPIs, and addressing trade-offs among them. AI can help determine, for example, under what conditions customer-centricity imperatives should take priority over improving operational efficiencies, or when to prioritize revenue considerations over market share investments. Integrating AI into the process for developing, setting, and prioritizing KPIs can be a disruptive change for managers who are accustomed to making these decisions themselves. To preempt or address such disruptions requires organizational, technological, and cultural investments.
Govern KPIs like assets, not tracking tools. Enhanced KPIs can redefine key performance, not just track it: That makes KPIs, and the capability to enhance them, valuable assets. As with other assets, KPIs can depreciate with time or inattention. Governing KPIs like assets, not tracking tools, ensures that they become more valuable, insightful, and predictive over time. Given a wide variety of asset management frameworks, effective governance means choosing the appropriate asset management framework for KPI assets, including relevant, underlying data assets. Some companies are already beginning to explore using KPIs for KPIs to ensure that the value of their KPI assets consistently improves.
Elevate KPI oversight. As KPIs become more intelligent and more effective proxies for corporate strategy, it becomes more important for boards to be involved with governing KPI development and use. To be sure, developing and using KPIs are management functions. However, boards sign off on strategies that KPIs both embody and inform. Signing off on strategy but not the company’s approach to developing KPIs is short-sighted and can undermine strategic aspirations. Engage the board directly in efforts to develop organizational capabilities for enhancing KPIs. Investing in enhanced KPIs is just as strategically relevant as investing in improving performance itself.
Conclusion
Our three-pronged approach to governing the development and use of KPIs expands on the idea that AI can transform KPIs into strategic assets that do more than track performance. They serve as a GPS for the organization, delivering greater foresight and a deeper understanding of interdependencies among key performance drivers.
Making the transition to AI-enhanced KPIs is not an incremental shift. It is a dramatic change from exclusively human, top-down approaches to setting and refining KPIs. Organizations that have made this transition are putting technology in the (KPI development) loop.
In the future, KPIs will no longer be passive tools for measurement but active agents for empowering decision makers. Ongoing innovation using generative AI promises to expand the purpose, power, and potential of smart KPIs. Holding conversations with chat-enabled smart KPIs, for instance, is already a goal for several of the executives we interviewed. With proper governance, empowering smart KPIs will empower strategists and managers alike.
Editor’s note: The authors would like to thank Gaurav Jha, David Zuluaga Martínez, Allison Ryder, and Barbara Spindel for their contributions to this article.
References
1. D. Kiron, M. Schrage, F. Candelon, et al., “Strategic Alignment With AI and Smart KPIs,” MIT Sloan Management Review, Sept. 5, 2023, https://sloanreview.mit.edu.
Acknowledgments
Pierre-Yves Calloc’h, chief digital officer, Pernod Ricard
Hervé Coureil, chief governance officer and security general, Schneider Electric
Jon Francis, chief data and analytics officer, General Motors
Emmanuel Frenehard, chief digital officer, Sanofi
Sameer Gupta, group chief analytics officer and managing director, DBS Bank
Holly Landry, chief data officer, Maersk