How to Have Better Strategy Conversations About Monetizing Data
Leaders can’t identify and manage data monetization opportunities if they can’t productively discuss the topic. Try this practical matrix approach.
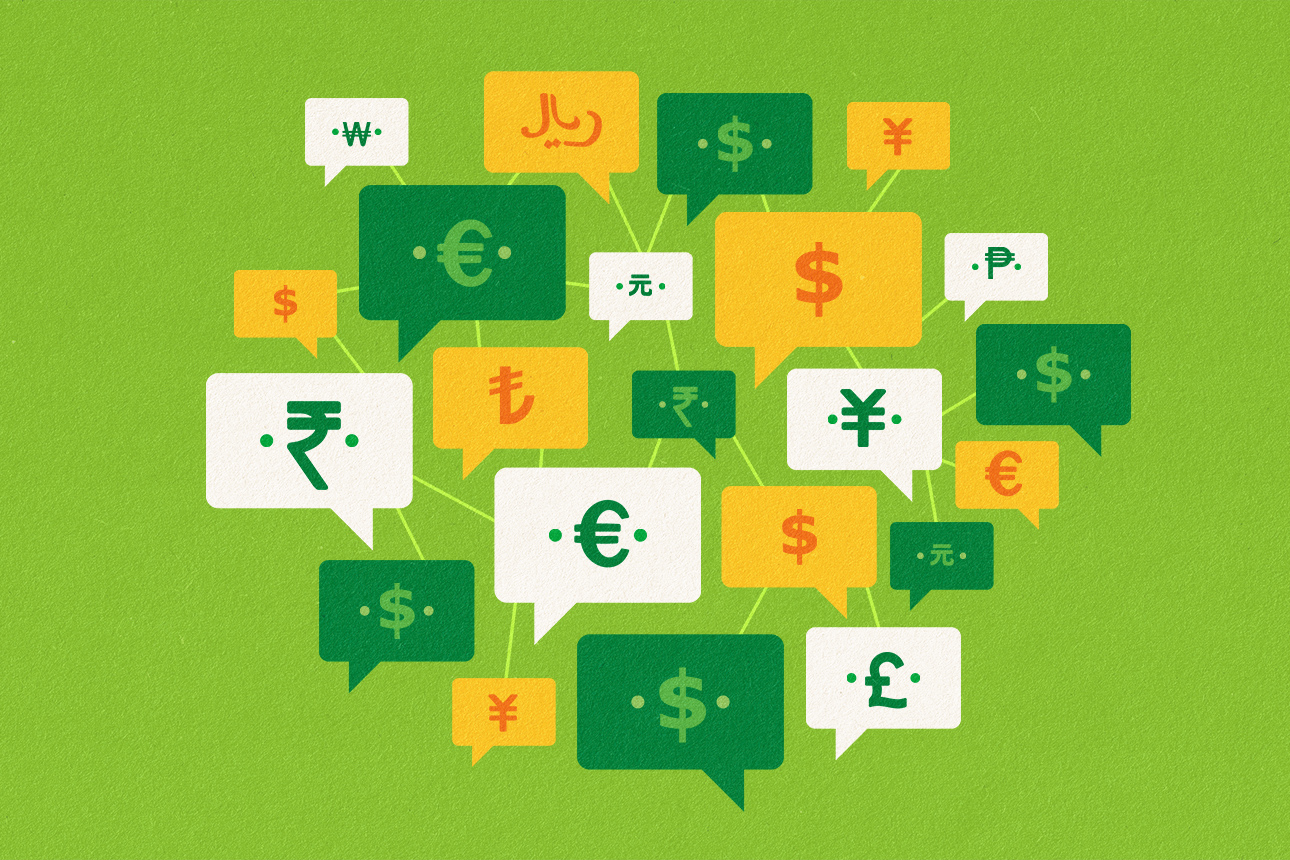
Carolyn Geason-Beissel/MIT SMR | Getty Images
Companies can’t manage what they don’t measure. They also can’t manage what they can’t discuss. Take the term data monetization: Definitions range from the narrowly focused “selling data sets” to the overly broad “creating benefits from data.” Too little consistency among curricula in academia and too much siloed business thought leadership only add to the proliferation of data babel. When leaders try to have productive conversations about a data monetization strategy within a complex business environment, they often reach an impasse. They need a simple, common language to break through.
Try using this definition: Data monetization is the conversion of data into financial returns. In the new book Data Is Everybody’s Business, we offer two simple data frameworks that — when combined — represent an easy yet comprehensive set of data products. The first framework offers three different approaches to converting data into money: Improve, wrap, or sell. The second framework reflects three points along the data value creation process: People or systems need to use data to develop insight that informs action. Combine the two frameworks, and you have a matrix that offers nine distinct product choices, each with its own set of commitments and outcomes.
Get Updates on Innovative Strategy
The latest insights on strategy and execution in the workplace, delivered to your inbox once a month.
Please enter a valid email address
Thank you for signing up
Leaders can use the data monetization matrix to explore current activities, future opportunities, and the interrelatedness of work. The simplicity of the matrix can help leaders be more transparent and open to breakthrough ideas. The objectivity of the matrix removes the heat from discussions and encourages conversations about how to monetize data instead of whether to monetize data.
Explaining the Monetization Approaches: Improve, Wrap, and Sell
If you sat down and began to list possible ways to create value from your company’s data assets, you likely could come up with hundreds of ideas. Fundamentally, however, you can organize your ideas into three buckets. Does the idea make a work task or process better, cheaper, or faster? If so, you’re looking at the improving approach. Does the idea make a product more valuable to customers? That’s wrapping. Does the idea identify information that a customer would pay for? You’re proposing selling. Improving, wrapping, and selling are very different in terms of risk, ownership, and requirements. When you know whether you are improving, wrapping, or selling, you can have smart conversations about data investments, capabilities, and returns.