Pair People and AI for Better Product Demand Forecasting
A new framework helps leaders orchestrate human and AI agents to accurately forecast product demand.
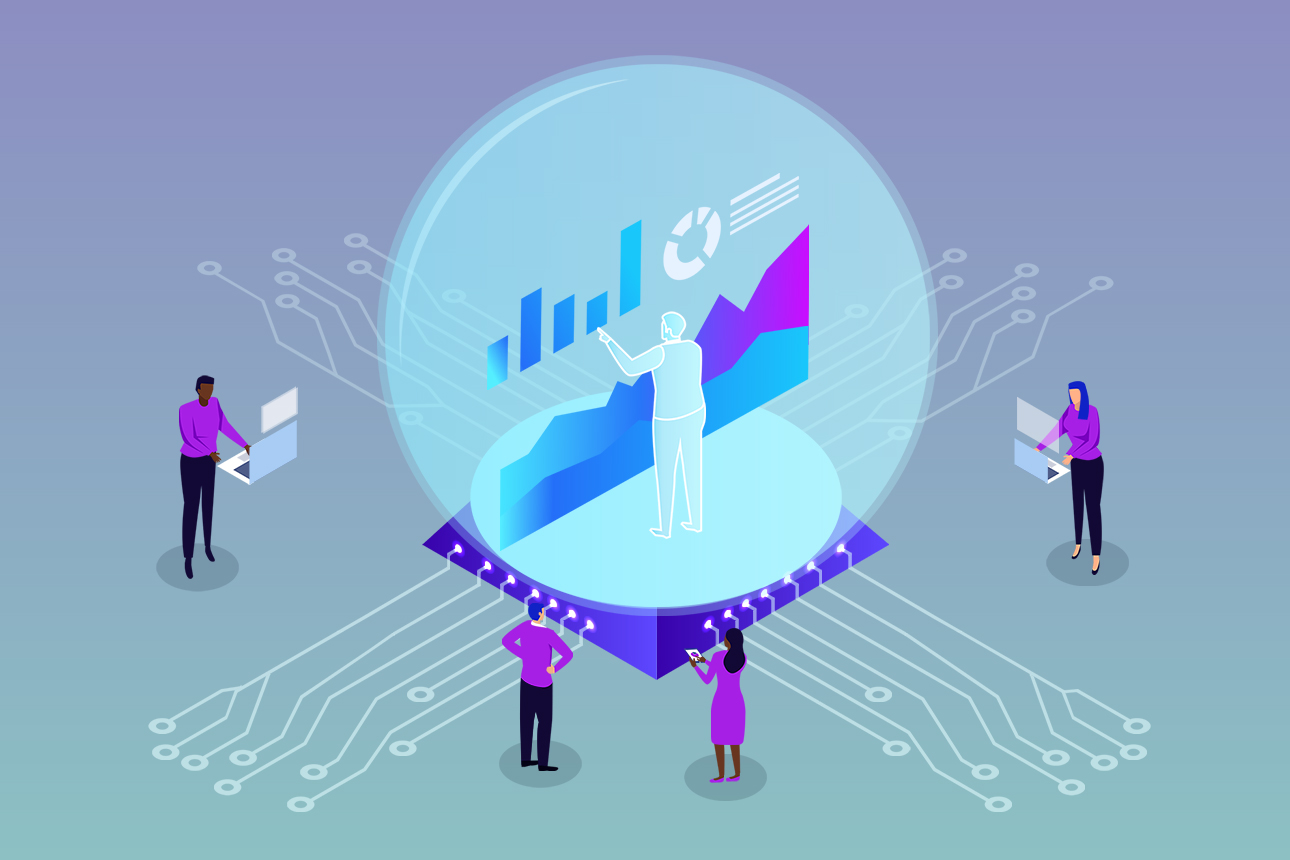
Carolyn Geason-Beissel/MIT SMR | Getty Images
Hot pink made a comeback this past summer. But as numerous fashion brands eagerly jumped on the Barbie-driven trend, many struggled to get their numbers right. Aldo, an official brand collaborator, saw its Barbie-based platform shoes fly off the shelves within 24 hours, thanks to the viral hashtag #TikTokMadeMeBuyIt.
The Barbie phenomenon highlights a modern dilemma: how to accurately forecast product demand when the commercial landscape is constantly changing. This has always been a challenge, but the retail environment is now more volatile and fad-driven than it was in the past, requiring companies to take demand forecasting to a higher level.
Get Updates on Innovative Strategy
The latest insights on strategy and execution in the workplace, delivered to your inbox once a month.
Please enter a valid email address
Thank you for signing up
Many companies have implemented next-generation tools like artificial intelligence to meet the challenge. While algorithms are improving forecasting performance, human interventions are still needed to contextualize market changes and bring other attributes, like responsiveness, to the table.1 Because there’s no standard template yet for such relationships, we have developed a framework to help companies marry human expertise with AI-driven forecasting solutions, based on a product’s characteristics.
Product Complexities
One hurdle to developing demand forecasting models today is the widely varying characteristics of different product categories. Short life cycle products, such as those in the fashion, beauty, and high-tech industries, have little to no historical data, making forecasting demand a challenging task. These products also pose a higher risk to companies because they are susceptible to higher stockouts or excess stock levels, as seen in the case of the Barbie merchandise. Long life cycle products, such as furniture and basic household appliances, on the other hand, benefit from an extended presence in the market and thus provide ample historical data to forecast their demand. These products require less-frequent adjustments in response to demand shifts. However, each product within a category might follow different demand patterns, and the demand forecasting methods used must reflect these variations.
Higher demand volatility typically involves high-risk products that are difficult to forecast, such as beauty products. Companies selling such items face frequent stockouts and lower service levels and aim for a responsive supply chain strategy to keep up with changing consumer demand patterns. In contrast, lower demand volatility — for example, for toilet paper — translates to stable demand, which is easier to predict; this makes efficiency through the minimization of supplier and delivery costs the focus of the supply chain.
The Human-AI Forecasting Framework
Our framework for customized demand forecasting provides a structured approach based on two factors: product life cycle and demand volatility. Based on this product life cycle-demand volatility matrix, human-AI forecasting methods can be divided into four quadrants: Demand Sense AI, Expert Edge, Traditional Extrapolation, and Cluster and Predict. (See “The Human-AI Demand Forecasting Framework.”)
The pie charts indicate the degree of involvement of each agent in the demand forecasting process. For products with both high demand volatility and a long life cycle, human intervention becomes the most critical factor because the forecasting task requires expert judgment and adaptability. Moving counterclockwise through the quadrants, the importance of human intervention decreases, and forecasting models can handle the task more effectively; products with short life cycles and high demand volatility require the least human involvement.
For products with both high demand volatility and a long life cycle, human intervention becomes the most critical factor.
For products with short life cycles, the primary role of humans is statistical modeling and data analysis. In contrast, for long life cycle products, forecasts depend on the domain knowledge of humans and their judgment more than their analytics and technical capabilities. Furthermore, short life cycle products might benefit more from advanced and agile forecasting techniques and therefore require investment in the latest technology, whereas long life cycle products might be well served by more traditional time-series analysis and human judgment.
What follows are descriptions of the roles of humans and AI for each of the forecasting methods, along with strategies for successfully implementing them.
Demand Sense AI: Short Life Cycle, High Demand Volatility
The role of AI versus human agents: Fast fashion brands such as Forever 21, H&M, and Zara have mastered the art of churning out fresh styles in record time, with some products hitting store shelves in as little as two weeks. The influencer hype on TikTok has catapulted Shein to stardom as consumers demand instant gratification.
AI models excel in this volatile environment because they assimilate information from a vast array of internal and external data sources to quickly react to changing demand patterns that would take longer for humans to forecast. Companies have gone beyond merely including data on past sales and product characteristics; Amazon mines the text of online product reviews, while H&M uses loyalty card data to learn about consumer buying patterns. AI-driven natural language processing allows companies to analyze tweets and Instagram posts to identify the latest trends, as well as what is no longer in fashion. German fashion retailer Zalando effectively uses deep learning models to predict demand and subsequently make pricing decisions.
In sharp contrast, human forecasters often struggle with predicting rapid changes in demand, especially when faced with complex time-series signals. These forecasters rely on simplified heuristics like anchoring (wherein an individual relies too heavily on an initial piece of information), which can result in subpar forecasts. But humans can help provide valuable inputs to AI models. For example, H&M has over 100 designers at its Stockholm headquarters who are responsible for spotting up-and-coming fashion trends and checking the algorithms for unreliable outputs. Essentially, for such products, humans play the role of data scientist and modeler and are highly involved in model development, feature selection and engineering, and the interpretation of model outcomes rather than participating in the forecasting activity itself.
Human forecasters often struggle with predicting rapid changes in demand, especially when faced with complex time-series signals.
Strategy for success: Companies in this category should invest in building digital capabilities internally. Zara initially adopted standard solutions from vendors and then gradually transitioned to developing its own demand-planning software, although the company continues to partner with vendors to develop other digital capabilities. Nike incorporated retail analytics platform Celect into its operations for inventory management. In contrast, Amazon develops all of its software in-house — an effort that is bolstered by a robust team of data scientists. Companies should also develop data hubs to capture high-volume, high-quality data from various sources and incorporate them into the AI models. It is recommended that organizations have skilled interpreters internally who understand how the AI models can effectively drive business decisions; while this is true for all categories, this particular segment is characterized by highly advanced AI/machine learning models.
Expert Edge: Long Life Cycle, High Demand Volatility
The role of AI versus human agents: For products with long life cycles, such as furniture, kitchenware, and other home goods, historical data can be advantageous in forecasting demand. Standard statistical models can therefore be implemented to learn from past patterns to predict future demand. However, humans have demonstrated their ability to capture the mean trend better than time-series models can. Managers possess valuable insights into market dynamics, customer behavior, and other external factors that can influence shifts in demand patterns. For long life cycle products, human expertise plays a critical role, in combination with simple statistical models such as regression, to achieve accurate and reliable demand forecasts. (During the COVID-19 pandemic, in particular, when historical data was deemed unreliable in a very volatile environment, human forecasters were forced to override model outputs.)
Strategy for success: Incorporating contextual information from internal and external experts is essential for enhancing the accuracy and relevance of forecasting models. How humans interpret and utilize information is as important as the abundance and quality of the information and depends on their professional competencies. Including company executives from various functions reduces bias and improves the quality of the inputs.
Traditional Extrapolation: Long Life Cycle, Low Demand Volatility
The role of AI versus human agents: In stable markets with gradual changes in demand trends and a wealth of historical sales data, such as batteries, electric vehicles, and aerospace components, statistical models can accurately predict future demand, reducing the need for frequent human intervention. Traditional models, such as time series, are typically better at forecasting demand trends based on historical sales and macroeconomic variables than advanced AI models are.
Nevertheless, when the market experiences unique events or shifts that are not adequately captured in the historical data, human forecasters, armed with their contextual knowledge and understanding of the business environment, can step in to make informed adjustments to the model forecasts. This human touch ensures that the forecasting process remains adaptable and responsive to changes. For example, lithium-ion battery demand forecasts often fall short of the market size and are frequently adjusted upward. Humans can also incorporate information pertaining to geopolitical events, technological advances, and regulatory changes into the model forecasts.
Strategy for success. While demand is stable for these products, high supply uncertainty can be driven by material, machine, or labor shortages. If an increase in lead times is expected, it might be necessary to stock up on inventories or accelerate shipments. For example, the battery supply chain also faces environmental, social, and governance challenges that can affect demand planning. Greater consumer adoption rates for greener technologies will lead to increased demand for batteries for electric vehicles. Therefore, companies need to be wary of the upstream challenges while implementing forecasting methods. Generative AI can be used to design the necessary production and delivery schedules to match stock levels.
Cluster and Predict: Short Life Cycle, Low Demand Volatility
The role of AI versus human agents: Highly innovative consumer technology products such as PCs, smartphones, and smart home appliances are available in the market for a span of less than five years. These products typically have a short growth phase followed by a quick decline in demand. The difficulty lies in achieving a precise life cycle forecast during the initial stages, when limited sales data is available. Dell, which started off as a manufacturer of configure-to-order computers and then pivoted to a make-to-stock model, sells computers with short life cycles with no maturity stage and a rapid decline. This stable pattern of demand is suitable for fitting simple product life cycle curves, such as the triangular and trapezoidal curves, after clustering the products based on similarity.2 Humans are involved in the forecasting process at Dell only when they run the product life cycle algorithm on a quarterly basis. Similarly, Philips applies Bass diffusion models (which predict new-product adoption in the market over time) on its consumer health care products, which are clustered based on the market.3
Strategy for success: Forecast accuracy undeniably influences safety inventory and capacity needs. However, it also profoundly affects sourcing commitments and transportation choices, especially for consumer electronics companies like Dell, which largely depends on the revenue from its new make-to-stock products. Therefore, the demand planner should consider these additional factors while devising a forecast, as well as the potential influence of newly introduced products that could cannibalize the demand for existing products. Additionally, the historical data of comparable products should be fed into the forecasting models. The AI models should also be flexible enough to make occasional adjustments for seasonal variations and promotional activities. Several product clustering algorithms should be tested to identify the most suitable one to use as the basis for demand prediction. They could be based either on the features of the product/market or on data-driven time-series similarity measures.
An Assured Future
Our framework will help companies raise their demand forecasting game, but will the need for human-AI collaboration wane as algorithms gain in sophistication and scope? We don’t believe so. The human-AI interface will continue to be vitally important because markets, and the context of market-turning changes, remain highly unpredictable. There will be new Barbie-style disruptions as well as geopolitical shifts and economic fluctuations.
In addition to providing invaluable contextual insights and quick reactions, humans can scale up the benefits of AI to other products and geographies. Importantly, humans can also train AI to understand the intricacies of context. The competitive value of human-AI duets that synergistically understand how market changes interact with each other and can respond with better demand forecasts will not decline anytime soon.
References
1. See E. Revilla, M.J. Saenz, M. Seifert, et al., “Human-Artificial Intelligence Collaboration in Prediction: A Field Experiment in the Retail Industry,” Journal of Management Information Systems 40, no. 4 (December 2023): 1071-1098.
2. K. Hu, J. Acimovic, F. Erize, et al., “Forecasting New Product Life Cycle Curves: Practical Approach and Empirical Analysis,” Manufacturing & Service Operations Management 21, no. 1 (winter 2019): 66-85.
3. X. Li, Y. Yin, D.V. Manrique, et al., “Lifecycle Forecast for Consumer Technology Products With Limited Sales Data,” International Journal of Production Economics 239 (September 2021): 1-10.