The Transformational Power of Recommendation
Recommendation engines are revolutionizing how customers buy and employees work.
Topics
Frontiers
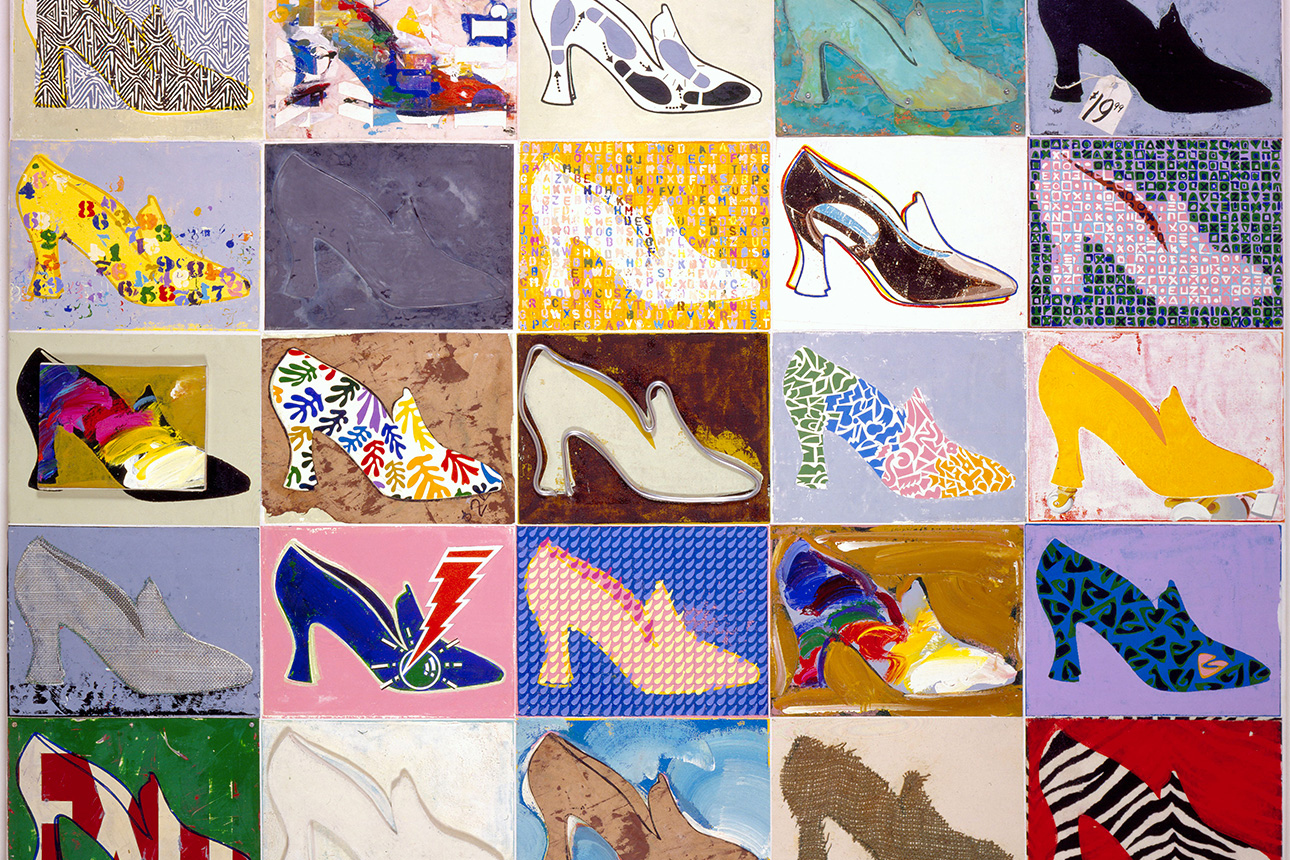
Image courtesy of Paul Giovanopoulos/theispot.com
Wikipedia defines recommendation engines (and platforms and systems) as “a subclass of information filtering system that seeks to predict the ‘rating’ or ‘preference’ a user would give to an item.”1 But as a tool, technology, and digital platform, recommendation engines are far more intriguing and important than this definition suggests.
In data-driven markets, the most effective competitors reliably offer the most effective advice. When predictive analytics are repackaged and repurposed as recommendations, they transform how people perceive, experience, and exercise choice. The most powerful — and empowering — engines of commerce are recommendation engines.
Get Updates on Leading With AI and Data
Get monthly insights on how artificial intelligence impacts your organization and what it means for your company and customers.
Please enter a valid email address
Thank you for signing up
Recommendation engines have been essential to the success of digital platforms Alibaba, Amazon, Netflix, and Spotify, according to their founders and CEOs. For companies such as these, recommendation engines aren’t merely marketing or sales tools but drivers of insight, innovation, and engagement. Superior recommendations measurably build superior loyalty and growth; they amplify customer lifetime value. Computing compelling recommendations profitably reshapes human behavior.
The influence and purpose of recommendation engines are not limited to customers or consumption. Large employers, most notably Google, have adopted and adapted recommendation engines as internal productivity platforms to nudge workers to their best decision options. Indeed, in late 2016, Laszlo Bock, the senior vice president of people operations at Google, left the company to launch Humu, a recommendation-engine startup for advising workforce behavior change.
While data remains the essential advisory ingredient, the global recommendations revolution reflects profound and ongoing algorithmic innovation, enabling machine learning and AI to power improvements in deep learning and generative adversarial networks. Successful recommendation engines learn how to learn. The more people use them, the smarter they become; the smarter they become, the more people use them. Done right, recommendation engines enable virtuous cycles of value creation.
The networked nudges and prompts of recommendation engines increasingly influence people’s choices in clothing, entertainment, food, and medicine; they also influence the texts we send, which friends we contact, the customers and prospects we prioritize, the experts we seek, the job candidates we hire, the investments we choose, the memos we edit, and the schedules we follow.
But prompts and nudges shouldn’t obscure the subtle but vital design principle that makes the recommendation-engine value cycle more virtuous: Recommendation is about ensuring better options and choices, not obedience or compliance. Recommendation engines don’t seek to impose optimal, best, or right answers on their users. To the contrary, their point and purpose are greater empowerment and agency. Influence, not control, is the algorithmic aspiration. In this, successful recommendation-engine design depends more on how recommenders seek to influence than on how much they know.
Recommendation engines transform human choice. Much as the steam engine energetically launched an industrial revolution, recommendation engines redefine insight and influence in an algorithmic age. Wherever choice matters, recommenders flourish, and this profound digital transformation of choice will only become more pervasive as recommenders become smarter. Better recommenders invariably mean better choices.
Helping Customers Make Better Choices Drives Business Growth
Shopping, commerce, and consumption are the most obvious examples of the growing influence of recommendation engines. “The recommender system is the key to the success of e-commerce websites as well as other indexing service providers, such as Alibaba, eBay, Google, Baidu, YouTube, etc.,” observed data scientists from JD.com, one of China’s largest e-commerce sites, in an academic paper describing next-generation recommendation engines.2
One reason is that more people have more choices around more opportunities in more domains than ever before: Amazon Prime Video, for example, offers more than 20,000 movies and videos; every minute, more than 500 hours of video are uploaded to YouTube; every day, more than 50 million photos are posted on Instagram. This great and growing abundance inherently shrinks the window for thoughtful decision. So people who want to make better choices are increasingly willing to accept recommendations from smart machines.
Spotify, the world’s largest independent music-streaming platform, with more than 200 million users, hosts more than 50 million songs. The Swedish innovator attained its scale by successfully making discovery, recommendation, and personalization pillars of its growth and user-experience strategies.
Discover Weekly is the company’s premier recommender system — and an incisive case study in how rethinking and combining recommendation algorithms profoundly changes people’s paths to novelty. Every Monday, Spotify customers receive a customized mixtape of 30 songs they’ve likely never heard before but are probabilistically likely to love. Quietly launched in 2015, when Spotify had about 75 million users, Discover Weekly was created by an ad hoc team without official support or sanction.
Similarity is Spotify’s secret sauce. Different algorithms address different features, attributes, and elements in the computational pursuit of delivering a predictably pleasant surprise to users. The algorithmic ensemble consists of a collaborative filtering algorithm, natural language processing, outlier/anomaly detection, a deep learning/convolutional neural network, and most obviously, how much the user liked or listened to the songs in the previous week’s Discover Weekly playlist. It examines users’ behaviors and identifies the critical commonalities between songs and artists, crawling user activity logs, user playlists, music news and reviews from around the web, and raw audio files — using spectrograms to capture qualities such as tempo, key, and loudness. The service then screens out music that users have heard before and sends out the bespoke playlists.
When testing Discover Weekly in early 2015, the ad hoc team quietly pushed its prototype to the Spotify accounts of all company employees. “Everyone freaked out in a good way,” recalled engineer Edward Newett, a Discover Weekly cocreator, “saying things like, ‘It’s as if my secret music twin put it together.’”3
Next, Discover Weekly was rolled out to 1% of the user base. Again, enthusiasm reigned. Then came the mid-2015 rollout to the rest of Spotify’s users around the world. It quickly became a hit, with users streaming 1 billion recommended tracks in its first 10 weeks.
Companies like Spotify are betting that helping customers make better choices — that is, making them smarter — will make them more loyal and profitable. The evidence suggests it’s a winning bet: One 2019 vendor survey asserts that personalized product recommendation accounted for almost 31% of the revenues in the global e-commerce industry. A Salesforce study found online shoppers are 4.5 times more likely to add items to their shopping cart and complete a purchase after clicking on any product recommendation. These are not marginal numbers.
Netflix observes that 75% of what people watch on the service comes from their personalized product recommendations. (Recommender data is also used to suggest new shows and original programming on the service.) Independent research strongly suggests recommenders directly and indirectly account for roughly a third of Amazon’s revenue. Alibaba’s self-reported recommender impact was even more impressive. China’s largest e-commerce platform disclosed that from the first quarter of 2015 to the first quarter of 2016 — a single year — its machine-learning-enhanced recommenders more than tripled their impact on the company’s gross merchandise volume (GMV) sales, which exceeded a half-trillion dollars in 2016.
Clearly, unavoidable conflicts of interest lurk here: Are recommendations computed with the company’s or the customer’s best interests in mind? Cui bono (who stands to gain)? Managing these competing interests reveals less about technical ingenuity than enterprise values. Empowering users is different from algorithmically exploiting them.
Recommenders enjoy their greatest power, influence, and value when trusted by users. Users who are confident that recommenders respect their best interests are open to the novel, unexpected, and unproven. They make themselves vulnerable.
That vulnerability creates real risks for manipulation and exploitation. As Dan Tunkelang, who oversaw recommender research at LinkedIn, observed, “The moment that recommendations have the power to influence decisions, they become a target for spammers, scammers, and other people with less-than-noble motives for influencing our decisions.”4
Technically, manipulating recommendation engines is relatively easy. Biasing recommendations to favor one brand or movie or restaurant or person or song over another is not hard. Simply put, betrayal is but a line of code away. Tricking users for one’s own benefit requires little ingenuity. But, pragmatically, is cheating worth it? Will discrete transactions truly trump investments in customer lifetime value?
Amazon’s Jeff Bezos flatly rejected the business rationale for distorting trustworthy recommendations. He noted and quoted a vendor unhappy with Amazon’s willingness to prominently post critical reviews, saying, “One wrote to me and said, ‘You don’t understand your business. You make money when you sell things. Why do you allow these negative customer reviews?’ And when I read that letter, I thought, we don’t make money when we sell things. We make money when we help customers make purchase decisions.”5
The recommendations people follow — and ignore — also reveal a great deal about who they are. By design and default, greater personalization requires more personal data and information. Seemingly unrelated data sets may algorithmically blend to yield surprisingly intimate insights. No one should be surprised that researchers and innovators worldwide declare their desire to build recommender systems that know more about what you want than you do.
This innovation aspiration makes security and confidentiality even more important. As with health care, informed consent becomes more important as recommenders grow more powerful, pervasive, and predictive.
Better Employee Choices Boost Performance
Recommenders promote greater personal — as well as enterprise — productivity. For instance, marketers and salespeople worldwide use recommenders to plot campaigns and target prospects. Enterprise leaders such as IBM and Salesforce both use and offer such sales recommenders, two examples of the expanding workplace analytics movement.
SalesPredict, an Israeli startup founded in 2012 and purchased by eBay four years later, developed analytics focused on recommending high-potential leads and prospects for sales teams. The company quickly discovered that while most salespeople didn’t want to be told what to do, they were open to data-driven suggestion. “Using the word ‘recommendations’ sounds a little awkward for some businesspeople, and we don’t exactly want that kind of Amazon-like connection,” said SalesPredict cofounder and CEO Yaron Zakai-Or. “But that is, in fact, the direction we went.”6
But how best to package and present these workplace analytics to boost productivity? Spreadsheets, dashboards, and dynamic visualizations only go so far. Which rhetorical formulation is more persuasive: “You should do this” or “Managers would like you to consider these next steps”? While many workplace analytics tools explicitly offer best or optimal or normative answers, others embrace recommender (em)power: Don’t digitally dictate; advise.
Launched in 2011 as an online subscription and personalized styling service for women, Stitch Fix grew to billion-dollar status by blending — and scaling — data science with fashion sense. In 2016 the company sold $730 million worth of clothing; $977 million in 2017; and more than $1.25 billion in 2018. Stitch Fix wants its brand to clothe men and families, too.
Bundling analytics, advice, and nudges into choice architectures that inspire both customer loyalty and copycat competition, the company is unabashedly data-driven. “Data science reports directly to me, and Stitch Fix wouldn’t exist without data science. It’s that simple,” said founder and CEO Katrina Lake.7
That commitment informs every link of the Stitch Fix value chain. Drawing on its inventory of roughly 1,000 brands — and Stitch Fix’s own labels — an algorithm generates a bespoke list of recommendations for each customer, which is reviewed by one of the company’s 3,500+ stylists on call.
The stylists are indispensable. “Take something like a leopard-print dress,” says chief algorithms officer Eric Colson. “Machines are pedantic: They can distinguish leopard print from cheetah print, but don’t have the social sense to know that a woman who likes leopard print would very likely also like cheetah print.”8
The stylist picks out five items — a “fix” — to send on a monthly, bimonthly, or quarterly basis, along with a personal note describing how best to style and/or accessorize the selected items. “Data enriched” stylists, Stitch Fix insists, bring an essential human touch to the selection process. Stitch Fix charges a $20 styling fee for each fix.
Stitch Fix’s division of recommendation labor, says Colson, leverages Nobel laureate Daniel Kahneman’s behavioral economics/choice architecture insights articulated in Thinking, Fast and Slow. “The machines take the calculations and probabilities,” he observes. “The humans take the intuition.”9
“A good person plus a good algorithm is far superior to the best person or the best algorithm alone,” asserts Lake. “We aren’t pitting people and data against each other. We need them to work together. We’re not training machines to behave like humans, and we’re certainly not training humans to behave like machines. And we all need to acknowledge that we’re fallible — the stylist, the data scientist, me. We’re all wrong sometimes — even the algorithm. The important thing is that we keep learning from that.”10
In the best-case scenario, successful recommenders will unlock a wealth of high-impact productivity opportunities. For example:
- An executive recognizes his written communications lack clarity, energy, and forcefulness, and shares his missives and messages with software like IBM’s Watson Tone Analyzer. The software proposes revisions, bringing force and focus to the prose.
- A global project manager seeks to encourage greater cooperation, collaboration, and esprit within her team. Her recommender performs social-network analyses, prioritizes project milestones, and reviews post-meeting communications to propose a daily checklist.
- A technically competent but uninspired user-interface designer wants to become and be seen as more boldly creative. His visual recommenders offer prototype imagery and wireframes based on those dimensions of creative and/or bold UX design.
In each use case, no right answer or normative solution exists. Instead, employees get clear, compelling, and customized choices they wouldn’t otherwise have.
Technologically, the workday is near when instrumentation and personal KPI dashboards will be able to physiologically sense when employees are not in the mood to take advice, respond to recommendations, or “Slack chat” the boss. These more granular self-data and analytics could become essential ingredients for boosting personal productivity and performance. Then, recommenders could deliver even better cues, nudges, and recommendations at the right moments.
With relentlessly ongoing innovation in machine learning, artificial intelligence, sensors, augmented reality, neural technologies, and other digital media, recommendation’s reach becomes more pervasive, powerful, and important. The recommendation future promises to be not just more personal, relevant, and better informed but transformative in ways guaranteed to (persuasively) surprise. A strategy and technology that learns to reliably deliver serendipity has long-term prospects.
This promise presents, for better or for worse, enormous opportunities and threats. To paraphrase Winston Churchill, we’re nowhere near the beginning of the end of recommendation engines innovation. It might fairly be observed, however, that we are at the end of the beginning.
References
1. “Recommender System,” last modified July 14, 2019, https://en.wikipedia.org.
2. Y. Yan, W. Guo, M. Zhao, et al., “Optimizing Gross Merchandise Volume via DNN-MAB Dynamic Ranking Paradigm,” Cornell University, Aug. 14, 2017, ArXiv:1708.03993.
3. M. Cowan, “How Spotify Chooses What Makes It Onto Your Discover Weekly Playlist,” Wired, Jan. 9, 2017, www.wired.co.uk.
4. D. Tunkelang, “Taste and Trust,” LinkedIn Pulse, Jan. 11, 2015, www.linkedin.com.
5. M. Schrage, “Invest in Your Customers More Than Your Brand,” Harvard Business Review, Feb. 25, 2013, https://hbr.org.
6. Y. Zakai-Or, interview with author, 2015.
7. K. Lake, “Stitch Fix’s CEO on Selling Personal Style to the Mass Market,” Harvard Business Review 96, no. 3 (May-June 2018): 35-40, https://hbr.org.
8. “Human-Machine Algorithms: Interview With Eric Colson,” Cloudera Fast Forward, May 25, 2016, https://blog.fastforwardlabs.com.
9. Ibid.
10. K. Lake, “Stitch Fix’s CEO.”
Comments (2)
Ruth Morss
Anonymous