Build a Diverse Team to Solve the AI Riddle
‘Hire English majors’ is common advice, but the practicalities of hiring, training, and integrating diverse team members are important.
Topics
Frontiers
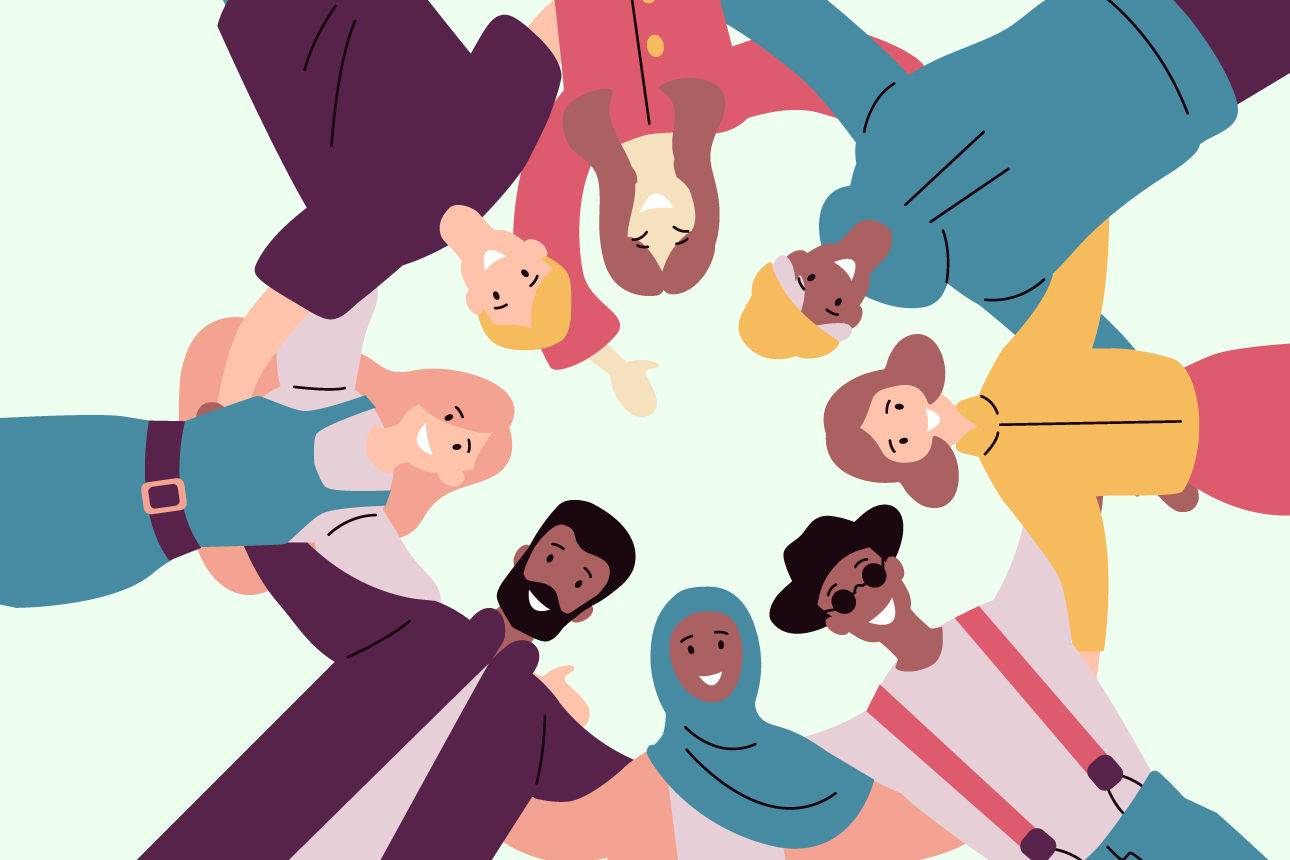
The term artificial intelligence describes algorithms that run on powerful computers to solve complex tasks, and computer scientists are indeed the most skilled at writing such algorithms. Yet systems designed by narrowly focused technical experts — such as computer scientists, engineers, and mathematicians — can produce disappointing results, as each expert sees every problem through the lens of his or her respective discipline. Mathematicians, for instance, attempt to solve every problem with statistics.
While it’s natural to assume that computer scientists play the lead role in AI development, not every problem lends itself to such obvious solutions. Systems that actually get the job done are in fact built by better-rounded teams. A diverse approach can maximize a project’s chance of success.
Get Updates on Leading With AI and Data
Get monthly insights on how artificial intelligence impacts your organization and what it means for your company and customers.
Please enter a valid email address
Thank you for signing up
When our team at Principal set out to create an AI-based decision support tool for financial analysts, we found that the essential ingredient was diversity — in our case, having enough English majors on the team.
It’s All About Text
Our goal was to craft an AI system that sifts through financial reports and news bulletins, appropriately highlighting the most urgent items to prioritize the critical information requiring human attention. This digital triage would enhance the analysts’ awareness of the most relevant market conditions so that their judgments would have a strong, factual foundation and thereby be more effective.
Converting the written word into the mathematical forms that a machine can process is no simple task. The very act of breaking down words can destroy meaning, which makes natural language processing tricky. The most commonly used AI software packages begin their analysis by jettisoning stop words such as the, was, and for, under the theory that these most common words in our language tend to add little information to a sentence. Then the process of lemmatization reduces words to their base forms, stripping away tense, mood, gender, and so on: studying and studied become study; went becomes go.
The machine has an easier time processing the compacted expression using statistics, largely because what remains is devoid of nuance and, ultimately, of meaning. This is why an AI textual analysis project benefits from having a more diverse development team with linguists on board — linguists who know there has to be a better way of processing language.